A Cross-Country Analysis
THE IMPACT OF REGULATION ON ECONOMIC GROWTH IN DEVELOPING COUNTRIES: A CROSS-COUNTRY ANALYSIS 1 ABSTRACT The role of an effective regulatory regime in promoting economic growth and development has generated considerable interest among researchers and practitioners in recent years. In particular, building effective regulatory structures in developing countries is not simply an issue of the technical design of the most appropriate regulatory instruments, it is also concerned with the quality of supporting regulatory institutions and capacity.
This paper explores the role of state regulation using an econometric model of the impact of regulation on growth. The results based on two different techniques of estimation suggest a strong causal link between regulatory quality and economic performance. Key words - economic growth; regulation; governance; developing countries; institutions. JEL classification: C23,I18, L33, L51, L98, O38, O50 2 Acknowledgement We would like to thank three referees for their perceptive comments on an earlier draft of this paper. The usual disclaimer applies. 3 1. INTRODUCTION
The role of an effective regulatory regime in promoting economic growth and development has generated considerable interest among researchers and practitioners in recent years (e. g. World Bank, 2004). Regulation can take many forms and the form of regulation policy adopted in developing countries has shifted over time (Minogue, 2005). From the 1960s to the 1980s, market failure was used to legitimise direct government involvement in productive activities in developing countries, by promoting industrialisation through import substitution, investing directly in industry and agriculture, and by extending public ownership of enterprises.
Order custom essay A Cross-Country Analysis with free plagiarism report
However, following the apparent success of market liberalisation programmes in some developed countries, and the evidence of the failure of state-led economic planning in developing ones (World Bank, 1995), the role of state regulation was redefined and narrowed to that of ensuring an undistorted policy environment in which efficient markets could operate. Deregulation was widely adopted, often as part of structural adjustment programmes, with the aim of reducing the “regulatory burden” on the market economy.
Privatisation and the more general process of economic liberalisation in developing countries have produced their own problems and failures and have resulted in the current focus on the regulatory state (Majone, 1994, 1997). The regulatory state model implies leaving production to the private sector where competitive markets work well and using government regulation where significant market failure exists (World Bank, 2001: 1).
Arguably, however, the performance of the new regulatory state remains under researched, especially in the context of developing countries with their own peculiar economic and social problems and institutional characteristics. Building effective regulatory structures in developing countries is not simply an issue of the technical design of the regulatory instruments, it is also concerned 4 with the quality of supporting regulatory institutions and capacity (World
Bank, 2002: 152). Many of the institutions that support markets are publicly provided, and the effectiveness of these regulatory institutions will be an important determinant of how well markets function. The quality of regulatory governance will affect regulatory outcomes, which in turn can be expected to impact on economic growth. This paper explores the role of regulation in economic growth using an econometric model.
More precisely, it assesses through econometric modelling the impact of variations in the quality of regulation on economic performance. Although earlier studies have looked at governance as a cause of cross-country productivity or income differences (Olson, et al. , 1998; Kauffman and Kraay, 2002), this paper differs in concentrating on regulation rather than wider governance issues. The results confirm that “good” regulation is associated with higher economic growth. The rest of the paper is organised as follows.
Section 2 reviews issues in the literature pertinent to the debate on the role of regulation in economic growth, before turning to regulatory measures and proxies for the quality of regulation. In section 3 the models used are presented. Section 4 deals with a descriptive analysis of the data and reports the regression results. The results confirm that the quality of state regulation impacts positively on economic growth. development policy. Finally, section 5 provides conclusions and the implications for 5 2. LITERATURE REVIEW (a) Regulation Theory
The theory of economic regulation developed from the nineteenth century and the literature is now vast (for recent reviews, see Laffont and Tirole, 1993, 2000; Levy and Spiller, 1994; Newbery, 1999). The case for economic regulation is premised on the existence of significant market failure resulting from economies of scale and scope in production, from information imperfections in market transactions, from the existence of incomplete markets and externalities, and from resulting income and wealth distribution effects.
It has been suggested that market failures may be more pronounced, and therefore the case for public regulation is stronger, in developing countries (Stiglitz 1998). More recent theoretical contributions to the regulation literature have provided a model of regulation for network industries that recognises the particular structural and institutional characteristics of developing countries and have highlighted the role of effective regulation in achieving equitable and sustainable expansion of infrastructure services in the poorer countries of the world (Laffont, 1999a; 2005).
However, regulation of markets may not result in a welfare improvement as compared to the economic outcome under imperfect market conditions. In particular, information asymmetries can contribute to imperfect regulation. The regulator and the regulated can be expected to have different levels of information about such matters as costs, revenues and demand. The regulated agent holds the information that the regulator needs to regulate optimally and the regulator must establish rules and incentive mechanisms to coax this information from the private sector.
Given that it is highly unlikely that the regulator will receive all of the information required to regulate optimally to maximise social welfare, the 6 results of regulation, in terms of outputs and prices remain “second best” to those of a competitive market, which centres attention on barriers to entry (Djankov et al. , 2002). Shapiro and Willig (1990) argue that state ownership provides more information to regulators than private ownership, so contracting should be less problematic when the state both owns and regulates.
However, state ownership is associated with inadequate incentives to gather and use this information to maximise economic welfare (Hayek, 1945). In other words, there tends to be a trade off between state ownership reducing the information asymmetries and hence transaction costs of regulation and the relative incentives under state control and private ownership for agents to maximise economic efficiency (Grossman and Hart, 1986; Sappington and Stiglitz, 1987; Shapiro and Willig, 1990; Yarrow, 1999).
Welfare-improving regulation assumes that the regulatory authority’s actions are motivated by the public interest. This has been criticised by public choice theorists who argue that individuals are essentially self-interested in or out of the public arena and it is necessary, therefore, to analyse the regulatory process as the product of relationships between different groups (Buchanan, 1972). This has been refined in the concept of “regulatory capture”, which involves the regulatory process becoming biased in favour of particular interests.
In the extreme case, the regulatory capture literature concludes that regulation always leads to socially sub-optimal outcomes because of “inefficient bargaining between interest groups over potential utility rents” (Newbery, 1999: 134; also, Laffont, 1999b). In the Chicago tradition of regulatory capture (Stigler, 1971; Peltzman, 1976), regulators are presumed to favour producer interests because of the concentration of regulatory benefits and diffusion of regulatory costs, which enhances the power of lobbying groups as rent seekers (Reagan, 1987). 7
Regulation is also subject to “political capture”; indeed, political capture may be a much greater threat than capture by producer groups outside of the political system. Where political capture occurs, the regulatory goals are distorted to pursue political ends. Under political capture, regulation becomes a tool of self-interest within government or the ruling elite (Stiglitz, 1998). More generally, it is to be expected that both the process and outcomes of a regulatory regime will be determined by the specific institutional context of an economy, as reflected in its formal and informal rules of economic ransacting (North, 1990). By setting the “rules of the game”, institutions impact on economic development (World Bank, 2002; Rodrik et. al. , 2004). Economic development is seen not simply as a matter of amassing economic resources in the form of physical and human capital, but as a matter of “institution building” so as to reduce information imperfections, maximise economic incentives and reduce transaction costs. Included in this institution building are the laws and political and social rules and conventions that are the basis for successful market production and exchange.
In particular, relevant modes of conduct in the context of the regulatory state might include probity in public administration, independence of the courts, low corruption and cronyism, and traditions of civic responsibility. “Institution building” including building a “good” regulatory regime is one of the most difficult problems facing developing countries and the transition economies at the present time (Kirkpatrick and Parker, 2004). (b) Regulatory Quality and Development Outcomes
The outcome of a regulatory system can be assessed against the yardsticks of effectiveness and efficiency. Effective regulation achieves the social welfare goals set down by the government for the regulatory authority. In developing countries, the social welfare objectives of regulation are likely to be not simply concerned with the pursuit of economic 8 efficiency but with wider goals to promote sustainable development and poverty reduction. Efficient regulation achieves the social welfare goals at minimum economic costs.
The economic costs of regulation can take two broad forms: (1) the costs of directly administering the regulatory system, which are internalised within government and reflected in the budget appropriations of the regulatory bodies; and (2) the compliance costs of regulation, which are external to the regulatory agency and fall on consumers and producers in terms of the economic costs of conforming with the regulations and of avoiding and evading them (Guasch and Hahn, 1999). Regulatory quality can also be assessed in terms of the criteria for good governance. Parker (1999: 224) argues that a well-functioning regulatory system is one that balances accountability, transparency and consistency. Accountability requires the regulatory agencies to be accountable for the consequences of their actions, to operate within their legal powers, and to observe the rules of due process when arriving at their decisions (e. g. to ensure that proper consultation occurs). Transparency relates to regulatory decisions being reached in a way that is revealed to the interested parties.
The third process which provides regulatory legitimacy is consistency. Inconsistent regulatory decisions undermine public confidence in a regulatory system. Inconsistency leads to uncertainty for investors, which raises the cost of capital and may seriously damage the willingness to invest. Since political intervention tends to undermine regulatory consistency, and politicians may be prone to alter the regulatory rules of the game for short-term political advantage, consistency is a primary argument for some kind of “independent” regulator.
This discussion suggests that the capacity of the state to provide strong regulatory institutions will be an important determinant of how well markets perform. An economy with a 9 developed institutional capacity is more likely to be able to design and implement effective regulation, which should contribute to improved economic growth. Weaknesses in institutional capacity to deliver ‘good’ regulation may be predicted to affect adversely economic development (World Bank, 2002). Evidence on the quality of regulation in developing countries is limited though growing.
But where research has occurred, the evidence suggests that the results of state regulation have been disappointing. A recent study of 13 Asian countries found that 80% of regulators had no access to training and regulatory offices were usually understaffed. The report concludes: “Asia’s governments rely too much on under-equipped and unsupported independent regulators to carry out tasks that are beyond their capabilities” (Jacobs, 2004: 4). In Latin America there is often a lack of political support for independent regulation and a lack of commitment to maintaining regulatory independence (Ugaz, 2003).
In the context of Africa, it was found that “regulation is being examined as part of individual sector initiatives, but these efforts are uncoordinated, and implementation is being left to follow privatization instead of being put in place concurrently” (Campbell-White and Bhatia, 1998: 5). A similar pattern of regulatory weaknesses can be discerned in the evidence for individual countries. In India, regulatory structures are associated with acute failures in institution building and with a bureaucratic approach that curtails enterprise (Lanyi, 2000).
South Africa’s proliferation of regulatory bodies is associated with a lack of clarity about roles and responsibilities and with the adoption of policy-making roles independent of government (Schwella, 2002: 3). In Malawi, the electricity industry regulator remains closely connected to the state electricity industry, compromising any notion of real regulatory independence and encouraging capture. 2 In Sri Lanka, the policies governing the regulatory process are judged to have been ad hoc and based on short-term political interests, with deficiencies apparent at each stage of 10 the process (Knight-John, 2002).
Experiences in the transitional economies also demonstrate much variability in the performance of the newly established regulatory institutions (Cave and Stern, 1998). In recognition that not all is well, the World Bank (2001: v) has stressed the importance of “improving regulatory regimes and building institutions and capacity effectively to supervise the private sector”. The Asian Development Bank (2000: 18) has also emphasised the need for improved regulation. Several papers have identified the causal effects of better governance on higher per capita incomes in the long run, using regressions with nstrumental variables on a cross-section of countries (Barro, 1997; Hall and Jones, 1999; Kauffman and Kraay, 2002). The causal chain between governance and economic outcome has also been examined. Some studies find that the quality of governance and institutions is important in explaining rates of investment, suggesting that one way in which better governance can improve economic performance is by improving the climate for capital creation (World Bank, 2003; Kirkpatrick, Parker and Zhang, forthcoming,). Olson et al. 1998) find that productivity growth is higher in countries with better institutions and quality of governance. Kauffman and Kraay (2002) reinforce these findings, relating the quality of governance to economic outcomes using a data set covering 175 countries for the period 2000-01. (c) Measures of Regulatory Governance The literature suggests, therefore, that the ability of the state to provide effective regulatory institutions will be an important determinant of how an economy performs. The major variable of interest is the quality of regulation.
Other researchers have operationalised the 11 broader concept of governance using two different groups of variables. The International Country Risk Guide (ICRG) data set is produced annually and covers three aspects of government – bureaucratic quality, law and order and corruption (Political Risk Services, 2002). Each variable is measured on a points scale with higher points denoting better performance with respect to the variable concerned. The assessment is based on expert analysis from an international network and is subject to peer review.
The ICRG variables have been used as proxies for the quality of governance in research (Neumayer, 2002; Olson et al. , 1998). The second set of governance variables comprises a set of six aggregate indicators developed by the World Bank and drawn from 194 different measures (Kauffman, Kraay and Mastruzzi 2005). These indicators are based on several different sources (including international organisations, political and business risk rating agencies, think tanks and non-governmental bodies) and a linear unobserved components model is used to aggregate these various sources into one aggregate indicator. The indicators are normalised with higher values denoting better governance. The six indicators provide a subjective assessment of the following aspects of a country’s quality of governance: Voice and accountability: respect for political rights and civil liberties, public participation in the process of electing policy makers, independence of media, accountability and transparency of government decisions. Political instability: political and social tension and unrest, instability of government.
Government effectiveness: perceptions of the quality of public provision, quality of bureaucracy, competence of civil servants and their independence from political pressure, and the credibility of government decisions. 12 Regulatory quality: burden on business via quantitative regulations, price controls and other interventions in the economy. Rule of law: respect for law and order, predictability and effectiveness of the judiciary system, enforceability of contracts. Control of corruption: perceptions of the exercise of public power for private gain.
The focus of this study is on regulation rather than governance. We therefore use the two variables in the World Bank data set that come closest to capturing the quality of the outcome and process dimensions of regulation, namely the regulatory quality and government effectiveness indices. The regulatory quality index measures the regulatory burden on business associated with inefficient quantitative controls and can be taken as a proxy for the quality of the outcomes of applying regulatory instruments. The government effectiveness index measures the quality of ublic provision, competence of civil servants and the credibility of government decisions, and can therefore act as a proxy for the process dimensions (consistency, accountability, transparency) of regulatory governance. The objective of the empirical analysis reported below, in section 3, is to test for a causal link between regulation quality and economic performance. The approach is to adopt a growth accounting framework, where economic growth is used as the measure of economic performance and regulation is entered as an input in the production function.
Neoclassical growth modelling began with the work of Solow (1956), who employed a neoclassical production function to explain economic growth in the USA during the first half of the twentieth century. Important assumptions of this approach are constant returns to scale and diminishing returns to investment, which imply that for a given rate of saving and 13 population growth economies move towards their steady-state growth path. This can be extended to differences in income levels between countries, to argue that in the long run income per capita levels will converge.
A lack of empirical support for convergence and the presence of a large, unexplained “residual” factor in the function estimates have presented a major challenge to these models. The endogenous growth theory put forward by Romer (1986) and Lucas (1988) led to renewed interest in economic growth analysis. An important advantage of endogenous over traditional growth models is that, through the assumption of constant or increasing returns to a factor input, in particular human capital, it is possible to explain a lack of growth and income convergence between countries and to account more fully for the residual factor in Solow-type analyses.
The “growth accounting” exercises, popularised by Barro and others (Barro, 1991, 2000; Barro and Sala-i-Martin, 1992), fall within the generalised Solow-type growth model. An important characteristic of this Most empirical approach is the inclusion of various indicators of economic structure. research using this approach has found evidence of “conditional” convergence, where convergence is conditional on the level or availability of complementary forms of investment, including human capital and a supportive policy environment.
This suggests that the failure of developing countries to converge on the income levels of developed countries may be attributed, at least in part, to institutional factors. 4 The importance of institutional capacity for the design and implementation of effective economic policy has been demonstrated in various empirical studies of cross-country growth, for example Sachs and Warner (1995) and Barro (2000). A similar approach is adopted in this study to examine the role of regulatory institutional capacity in accounting for cross-country variations in economic growth.
An issue that needed to be addressed at the outset is causality. It could be argued that instead of regulatory quality determining economic growth, regulatory quality could be determined 14 by the economy’s growth rate. Economies that grow faster are able to generate higher levels of income and are therefore able to support the development of better institutions. Or, alternatively, there may be a level of simultaneity, in the sense that institutional quality generates more sustained economic growth, which in turn supports more and better regulatory institutions.
The Granger causality test is commonly used in empirical work to establish the direction of causation. However, this test is sensitive to the length of lags of the variables used and therefore requires a relatively long time series dimension to be able to select the right length of lag and to be relatively confident about the conclusion drawn. Since the time dimension of our regulation data is limited, we are unable to apply the Granger causality test.
Fortunately, there is a substantial literature that indicates that better governance leads to higher income rather than causation being in the opposite direction (Olson et al 1998; Acemoglu et al 2000; Rodrik et al 2004). Kauffman et al (2005: 38) implement an empirical procedure for testing for causation, which leads to the identification of strong positive causal effects running from better governance to higher per capita incomes and suggest that a one standard deviation improvement in governance leads to a two- to three-fold difference in income levels in the long run. The authors state, ‘Some observers have argued that ….. here is a strong causal impact of income on governance. However, we argue that the existing evidence does not support a strong causal channel operating in this direction – most of the correlation between governance and per capita income reflects causation from the former to the latter’ (Kauffman et al 2005, p3). They conclude: “available evidence suggests that the causal impact of incomes on governance is small. Rather, the observed correlation between governance and per capita incomes primarily reflects causation in the other direction: better governance raises per capita incomes”.
However, we accept that because we are unable to rigorously demonstrate causation in our modelling, the results should be read with this caveat. 15 Endogeneity is another issue that should be addressed. To cope with the possible problem of endogeneity, a 2SLS or IV technique can be used. But to to do this effectively requires good sets of instruments for the variables that potentially could suffer from this problem, including lags of the variables concerned. Once again, data availability, particularly relating to the regulatory proxies, does not permit an effective test for endogeneity.
We accept that this remains a weakness. 3. THE MODELLING The approach used in the modelling is to assume that each country’s production possibility set, in common with most literature in this area, is described by a Cobb-Douglas production function: Yit Ait K it Lit (1) where Y is the output level; A, level of productivity; K, stock of capital; and L, stock of labour - ‘i’ and ‘t’ stand for country and time respectively. Assuming that the production function exhibits constant return to scale with respect to physical inputs, (2) can be written in per capita terms as: yit
Ait k it (2) where lower case letters refer to per capita units. Assume a simple Keynesian capital accumulation rule according to the following specification: 16 dk / dt sy (n )k (3) where dk/dt is the rate of change of the per capita capital stock, which is assumed to be equal to the flow of saving (equal to investment) minus capital depreciation and the growth of the labour force. In this equation s is the share of gross saving in output per capita, is the depreciation of capital and n the rate of growth of population as a proxy for the growth of the labour force.
Setting (3) equal to zero gives us the steady state solution for the stock of per capita capital; k=sy/(n+ ). Taking the logarithm of both sides of equation (2) and replacing the steady state solution for k from above into (2) gives the steady state solution for output per capita, which is as follows: * ln ( yit ) [1/(1 )][ln Ait ln ( sit /(nit it )] (4) Where (*) above the variable signifies the steady state solution. We adopt the Mankiw et al. (1992) assumption that economies move towards their steady state solution according to the following approximation: n yit lnyi 0 * (lnyit lnyi 0 ) (5) where y0 stands for the initial level of per capita income, and (1 e t ) is the adjustment dynamic towards steady state, where ' ' is the speed of convergence. From (5) we can solve for the growth of per capita output, which is as follows: 17 git * ( / t ) (lnyit lnyi 0 ) (6) * Replacing ( lnyit ) by its equivalent from (4), gives us a relationship for actual growth of per capita output: git ( / t (1 ))[ln Ait ln( sit /( nit it )] ( / t )lnyi 0 (7) Total factor productivity plays an important role in growth. We assume that ts dynamic takes the following form: Ait Ai 0 e it (8) Where Ai0 specifies the initial level of productivity and ‘ ’ its rate of efficiency growth per period. Substituting for A from (8) into (7), per capita growth of output (g) is represented by the following relationship: g 1 ln Ai 0 2 i 3 ln( sit /(nit it )) 4 lnyi 0 (9) where 1 / t (1 ), 2 /(1 ), 3 / t (1 ), and 4 / t. Adding some control and qualitative variables as well as a stochastic term to (9) provides the model which we use to assess the role that regulatory quality plays in economic growth. 18
Variables added to equation (9) broadly follow the growth empirics literature, such as Barro (1991, 2000), Mankiw et al. (1992) and Islam (1995). Amongst the control variables included in most empirical research are initial conditions, both in terms of the level of development (as proxied by GDP per capita) as well as human capital and institutions. Most also include proxies for the macroeconomic environment such as inflation, trade openness and the government’s involvement in economic activities. Qualitative variables can also be added to account for specific events in a country, as well as data heterogeneity when panel data are used.
In our analysis, depending on the nature of data set constructed, we make use of all or some of these variables with the aim of ensuring that our regressions are appropriately specified. In the context of our specification in (9), similar to Temple and Johnson (1995), we make the additional assumption, drawing on the literature relating to regulation in developing countries reviewed earlier, that the rate of efficiency growth ’ ’ directly varies with the quality of regulatory institutions in the country.
Those countries with good institutions in place can design and implement policies that allow them to continue with their future growth. If instead the country in question lacks or has a weak institutional structure, its growth potential is likely to be diminished because the design and implementation of appropriate policies are then adversely affected. In the case of developing countries, in particular, to be able to benefit from being a latecomer in terms of industrialisation and grow at a high speed to “catch up”, it is important that institutional supports are present to realise the potential for income convergence.
One of the control variables that is likely to be important in this context, is initial institutional quality. In the absence of better information about the initial institutional quality, we adopted 19 educational attainment as a proxy variable. At first reading this may seem an unusual choice, but our proxy, secondary school enrolment, is correlated with the regulatory governance variables we are using (see Table 1 below) and it has been successfully used as a proxy in other studies. 5 The finding that education is highly correlated with our regulatory variables is an nteresting finding in itself and one worthy of exploration in future research. We apply two methods of estimation to the model specified by equation (9). One is based on cross-section analysis, in which we attempt to measure directly any possible impact that regulation has on economic growth. The second is based on panel data, in which we indirectly estimate the growth contribution of regulation. The reason for applying different estimation procedures is due to our data on the indexes of regulation; we have a few observations per country.
Therefore, for the cross-section regression we average the relevant data over the period 1980-1999 and combine the result with the regulation data. 6 This allows a direct measure of the possible role that regulation plays in growth, using equation (9) as a base to estimate 2 . In the second method we adopt a variant of the one applied by Olson et al. (1998) and apply the fixed effects technique7 to the panel data constructed. This data set combines cross-section and time-series data for the countries included in the first data set.
This procedure, which essentially involves including a dummy for every country in the estimated equation, produces consistent estimates even where data are not available for some time-invariant factors that affect growth. The fixed effects estimator does require, however, that each included variable varies significantly within countries. Clearly, even if available, the regulatory variables may not satisfy this requirement since institutions usually change slowly. The estimation procedure, therefore, involves two stages. We first regress GDP per capita growth in each country per period, git on ln ( sit /(nit it it ) plus a set of country dummies. The coefficient on the country dummies reflects the effect on growth of all the 20 time-invariant variables, including regulatory institutions. In the second stage we use the coefficients of the country dummies as the dependent variable and regress them on the measures of regulatory quality and control variables. The coefficients on the measures of regulatory quality in the second stage regression reflect the impact of regulation on GDP per capita growth after controlling for capital accumulation and certain other variables. 4. THE DATA AND THE REGRESSION RESULTS
Data for the regulatory quality measures were set out in Kauffman et al (2005) and are available for downloading from the World Bank web site. 8 As discussed earlier, the two regulation indicators used from this study are regulatory quality and government effectiveness measures. Other data required for the regression analysis were taken from the World Bank’s World Development Indicators. The data set used in the analysis covers 117 countries for the cross-section regression and 96 for the panel version of the regression (for a full list of the countries see the Appendix).
Although the main focus of the study is the impact of regulation on economic performance in developing countries, a heterogeneous data set was used including some transitional and advanced countries as well as developing ones. The reason for including some nondeveloping countries was to improve the statistical reliability of the results by including more countries, with regional dummies used to capture the differing levels of economic development. However, as a cross-check on our results we repeated our analysis removing the developed countries from the data base. The results were substantially unaffected (these results can e obtained from the authors). As information on regulatory governance is only 21 based on one year, in the cross-section model, all other variables were converted into one period by averaging for 1980-2000. Initial effect variables relate to 1980. For the panel version, the data cover the period 1980-2000 (in common with most empirical research in this area, and in order to remove short-term disturbances as well as business cycle effects from the data, we have converted the time series data for the variables into 5-year period averages covering 1980-84, 1985-89, 1990-94 and 1995-99).
However, the time series dimension is not complete for a number of the countries in the data set and therefore the panel data are unbalanced, containing 432 observations. Table 1 provides the correlation coefficient matrix for the key variables used in the study. (Table 1) The first data column in Table 1 shows the simple correlation coefficients between the dependent variable, GDP growth per capita, and possible explanatory variables. The correlation coefficients have the expected signs.
The correlation coefficients between the indicators of regulatory governance, namely government effectiveness and regulatory quality, and GDP per capita growth have the expected positive sign. The bivariate correlations between inflation and the regulatory proxies used are negative, supporting the proposition that economies with better regulatory governance are also better able to design macroeconomic policies that stabilise the economy and control inflation.
There is also a high correlation between the logarithm of initial GDP per capita and initial secondary school education, both of which are in turn correlated with the various proxies for regulatory governance. 9 This suggests that, included in the same regression, parameter estimates for these variables may not be individually reliable, due to multicolinearity. This is also the case with the two regulatory proxies that we intend to use in the analysis, namely government 22 effectiveness (GE) and regulatory quality (RQ). These two are highly correlated and herefore cannot be included in the same regression in order to estimate each variable's contribution. For this reason we considered first the contribution of each of these proxies to growth in separate regressions, and then combined them by addition to form a composite regulation variable (RQGE). Before formal analysis of the model specified in (9), we checked for the possibility of convergence in our data. In general, the literature does not support unconditional convergence (Barro, 2000; Mankiw et al. , 1992; Islam, 1995) but instead finds evidence of conditional convergence. We investigated this issue using regulatory governance as a ossible pre-condition for convergence. Table 2 presents the results. There is no indication of unconditional convergence (Reg. 1 and 2), the sign on the initial GDP per capita variable (LIGDPPC) is positive. However, once an indicator of governance is included (RQ, GE and RQGE), as in Reg. 3 to 5, there is an indication of conditional convergence in the form of a negative sign. Differences between growth experiences of countries are partly explained by their state of regulatory quality. There is no indication that there is any significant regional difference in this context (cf. reg. -8, which include regional variables for Africa, Asia and Latin America). (Table 2 here) In addition to combining the two regulatory proxies (RQ and GE), and in the light of high correlation between the two, the first principal component of these two was generated (PCRQGE) and this composite index was used as a regulatory proxy. Results generated based on this proxy, as indicated by Reg 5a in table 2, are the same as those reported using 23 RQ, GE and RQGE10. We repeated this process taking into account the other four indicators of governance identified by Kauffman et al (2005) and detailed earlier.
The first principal component of all the six indicators of governance (termed PC All) was generated, as well as one based on the four, excluding RQ and GE - termed PC Others. Reg 5b and Reg 5c in Table 2 include the results based on these composite indexes. Inclusion of the four indicators of governance alongside or instead of the two regulatory proxies combined (RQGE) and its principal component (PCRQGE) has a marginal effect on the parameter estimates for the other variables in the regression, but the signs remain the same. The coefficient values for PC All and PC Others are, however, lower than for the other regulation variables.
We interpret this result as being an indication of the differential influence of different governance proxies on growth. In other words, a possible criticism of our findings that various measures on institutional quality could be highly correlated and that it is institutional quality rather than the quality of regulation in particular that matters is not borne out. More precisely, the regulation proxies we have used (RQ, GE, RQGE and PCRQGE) seem to have a higher impact on growth than the other four indicators of governance identified by Kauffman et al (2005) reflecting wider institutional factors.
Therefore, regulation rather than governance issues more generally seems to have the larger impact on growth. 11 Having considered the issue of convergence and considered the possible relative effects of regulation and governance issues more generally on growth, Tables 3 and 4 report results based on the formal analysis of the data. The results address the main focus of the research, the impact of regulation on the growth in GDP per capita. The results reported in Table 3 are based on the model specified in equation (9) using OLS and cross-country data, as detailed above.
Table 3 reports ten regressions, each containing different combinations of the independent variables in our data set. The economic variables in the full set of regressions 24 tested included the variables derived from the model itself, as specified in equation (9), and measures for general inflation, trade, government expenditure, as well as the regional dummies. However, with the exception of inflation these other variables proved to be statistically insignificant at the 10% level or better and therefore, to economise on space, the results are not reported.
The inflation variable was found to be statistically significant and negative, suggesting that unstable macroeconomic conditions have a negative effect on economic growth. (Table 3 here) The regional dummies were used to test the hypothesis that different regions may have characteristics that affect growth differently. This is validated with respect to Asia, confirming that this region had, on average, performed better with respect to economic growth than other regions in the period studied. A dummy for Africa and Latin America were found to be statistically insignificant. We also included the initial level of human apital, as measured be secondary school enrolments, as a proxy for the initial level of “institutions”. As indicated in Table 1 this variable is highly correlated with initial GDP per capita, and the results in Table 3 confirmed that it has a negative sign and is statistically significant. This result supports the conditional convergence hypothesis. The regulatory variables are correctly signed and statistically significant in all cases. The sign and level of significance of the parameter estimates for these regulatory proxies indicate that they have a statistically significant and positive effect on economic growth.
Based on the estimates for the combined regulatory variable (RQGE), a unit change in the quality and effectiveness of regulation is, on average, associated with approximately an 0. 6% to 0. 9% 25 increase in economic growth, everything else remaining equal. As with the other results reported, the regulatory proxies used here seem to have a larger impact on growth than do the other governance proxies, namely the variables PC All and PC Others. One objection to our analysis so far is that we have used regulatory data for 2000 only. Perhaps the regulatory environment has changed substantially during the period 1980-2000.
Unfortunately, World Bank regulatory data do not exist prior to 1996. But as a cross-check on the stability of the results if regulatory data for other years from 1996 are used, we first considered the correlation between the World Bank regulatory indicators between 1996 and 2000. The results gave correlation coefficients of 0. 92 to 0. 99 confirming a high degree of stability. Nevertheless, we then re-ran our regression reported in Table 3 using regulatory indicators (constructed as before) but for 1996, 1998 and 2000 separately. The results were almost identical.
As discussed earlier, the stability in the governance variables plus the very limited observations on governance (a maximum of two for each country) caused us to rule out the use of regressions based on panel data. (Table 4 here) Table 4 reports results based on the second method of estimation, which, as discussed earlier, involves two stages. In the first stage, by applying a fixed effect technique to the panel data, we arrive at the following regression results: GDP per capita = 0. 133 Log net12 gross capital formation – 0. 148 Log initial GDPPC (6. 41)* (6. 57)* 26 +0. 4 Log net schooling + Country Dummies (1. 84)** Adjusted R2 =0. 21; number of observations=432 The figure in brackets is the t-ratio; * (**) indicates significance level at 5% (10%). From the above, the regression parameter estimate associated with the country dummies is saved and used as the dependent variable in the regressions reported in Table 4. For reasons of space we report only a sub-set of the full results. We exclude reporting regressions including the full set of independent variables used, as detailed in Table 1, because a number of them proved to be statistically insignificant.
Our main interest in the regression results reported in Table 4 is with the role that the regulatory proxies are playing in explaining the variation in the country dummies. The results are consistent with those reported in Table 3. Even though the parameter estimates for the regulatory variable are lower, regulatory governance still affects the growth performance of an economy. The regional dummies in this case are all negative and statistically significant, relative to the control group which is advanced countries13.
These changes in the results were investigated and seem to reflect the differences in the modelling methods adopted, suggesting that in this type of research the modelling can affect the results. Nevertheless, the overall picture that emerges is that the quality and effectiveness of regulation has a positive effect on growth using both models. 27 5. CONCLUSIONS The provision of a regulatory regime that promotes rather than constrains economic growth is an important part of good governance. The ability of the state to provide effective regulatory institutions can be expected to be a determinant of how well markets and the economy perform.
The impact of regulatory institutions on economic growth will depend on both the efficiency of the regulatory policies and instruments that are used and the quality of the governance processes that are practised by the regulatory authorities, as discussed in the early part of the paper. This paper has tested the hypothesis that the efficiency and quality of regulation affects the economic performance of an economy. Two proxies for regulatory effectiveness were included separately and then combined as determinants of economic growth performance, using both cross-sectional and panel data methods.
The results from both sets of modelling suggest a strong causal link between regulatory quality and economic growth and confirm that the standard of regulation matters for economic performance. The results are consistent with those of Olson et al. (1998) who found that productivity growth is strongly correlated with the quality of governance, and Kauffman et al (2005) who found that the quality of governance has a positive effect on incomes. As we highlighted earlier, the proxies we use for regulatory governance are correlated with a number of other institutional proxies.
One could argue, therefore, that what we have established could equally hold for the link between institutional capacity in general and economic performance. However, the literature reviewed earlier in the paper is consistent with institutional capacity playing a strong and complementary role to regulatory governance 28 and the principal component analysis undertaken is supportive of this view. Nevertheless, the ability to model separately institutions in general and regulatory institutions or governance in particular remains problematic because of their potential complementarity.
Hence, our results are perhaps most safely interpreted as demonstrating the importance of regulatory quality for economic growth in the context of wider institutional capacity building. Also, we acknowledge that in our analysis there is no control for the different regulated industrial sectors including privatised industries. Hence, the results need to be interpreted with care because of the heterogeneity of the sectors covered. The possibility that regulatory quality inputs differently across different industrial sectors cannot be ruled out.
Unfortunately, data limitations prevented us from pursuing this issue. Finally, we acknowledge that the direction of causation between economic growth and regulatory quality deserves further investigation, Nevertheless, despite these caveats, we believe that there are good a priori grounds for assuming that better regulation leads to more rapid economic growth and that our empirical results are consistent with the view that “good” regulation is associated with higher economic growth in lower-income economies. 29 APPENDIX (a) List of countries included in the dataset14:
Angola; Albania; Argentina; Australia; Austria; Azerbaijan; Belgium; Benin; Burkina Faso; Bangladesh; Bulgaria; Belarus; Bolivia; Brazil; Botswana; Canada; Switzerland; Chile; China; Cote d'Ivoire; Cameroon; Congo, Rep. ; Colombia; Costa Rica; Cyprus; Czech Republic; Denmark; Dominican Republic; Algeria; Ecuador; Egypt, Arab Rep. ; Spain; Estonia; Ethiopia; Finland; France; Gabon; United Kingdom; Georgia; Ghana; Guinea; Gambia; Greece; Guatemala; Guyana; Hong Kong (China); Honduras; Croatia; Haiti; Hungary; Indonesia; India; Ireland; Iran, Islamic Rep. Iceland; Israel; Italy; Jamaica; Jordan; Japan; Kazakhstan; Kenya; Kyrgyz Republic; Korea, Rep. ; Lebanon; Sri Lanka; Lesotho; Lithuania; Luxembourg; Latvia; Morocco; Moldova; Mexico; Macedonia; Mali; Malta; Mozambique; Mauritius; Malawi; Malaysia; Niger; Nigeria; Nicaragua; Netherlands; Norway; New Zealand; Pakistan; Panama; Peru; Philippines; Papua New Guinea; Poland; Portugal; Paraguay; Romania; Russian Federation; Senegal; Singapore; Sierra Leone; El Salvador; Sweden; Syrian Arab Republic; Togo; Thailand; Trinidad and Tobago; Tunisia; Turkey; Tanzania; Uganda; Ukraine; Uruguay; United States; Venezuela; Vietnam; Congo, Dem.
Rep. ; Zambia; Zimbabwe. 30 NOTES 1. The World Bank defines good governance as “epitomized by predictable, open and enlightened policy making; a bureaucracy imbued with a professional ethos; an executive arm of government accountable for its actions; a strong civil society participating in public affairs, and all behaving under the rule of law” (World Bank, 1997). 2. 3. One of the authors of this paper has been involved in the design of regulatory institutions for Malawi.
This expresses the observed data in each cluster as a linear function of the unobserved common component of governance, plus a disturbance term to capture perception errors and sampling variation in each indicator. 4. However, neither neoclassical nor endogenous growth theory gave regulation an explicit role. By assuming that output is at the limit provided by the available factor inputs and technology, neoclassical growth theory implicitly assumed no regulatory distortions. 5. Benhabib and Spiegel (1994) argue that the initial level of human capital can affect the growth path of productivity.
Olson et al (1998) also use secondary school enrolment as a proxy explanatory variable in their growth study. 6. The most recent data set provided by Kauffman et al (2005) provides bi-annual data on indicators of governance over the period 1996-2004. In common with most empirical research in this area, we have converted time series data on the variables we have used in this study into 5-year averages for the period 1980-2000. However, if we were to do the same with the regulatory indices available it would give us only one observation for each country. If we were to extend our data to 2004, we would get two observations on these indices.
Time dimensions of data on regulatory governance in either case would be too few to be able to apply panel data. In addition, given that these indicators change very slowly over time, as also acknowledged by Kauffman et al. , and that they only relate to the most recent periods, we do not find it informative to try to use them in a panel data analysis. We were able to confirm the stability of the regulation variables by replacing the data for 2000 with data for 1996 and 1998. The effect on our results was negligible (the results can be obtained from the authors). 7.
There are two estimation procedures for panel data, fixed and random effects. In our case, the fixed effect method is the more appropriate one to use for the following reasons: (a) a priori we expect that 31 regulatory governance proxies to be correlated with the intercept term for each country; those with a poor or weak regulatory governance are also expected to perform relatively badly in terms of economic performance; (b) we are interested in measuring differences between countries included in our data set; the parameter estimate for country dummies (the intercept term for each country) is a proxy for these differences.
Intercepts in turn are used as a dependent variable in the second stage regression to establish the link between regulatory governance and country characteristics captured by the intercept term. The fixed effects method allows us to do this; (c) in small samples, similar to the one we are using here, there may be practical problems preventing parameter estimation when the random effect model is applied; this is not the case with the fixed effect model. For a more detailed discussion of these issues, see Verbeek (2000).
Also, we applied the Hausman specification test and this confirmed that the fixed effect model is the more appropriate technique for our data. 8. http://www. worldbank. org/wbi/governance/pubs/govmatters4. html The series constructed are composite indexes, which are based on a number of variables generated at different points in time. Information for each country on these proxies, therefore, generally relates to a period rather than a specific year. Kauffman and Kraay (2005) highlight certain issues relating to the quality of the data used, particularly when it is utilised for making comparisons across countries.
However, we are not aware of better regulatory quality data, while conceding that better quality data could reveal different results to those reported here. Nevertheless, based on the significance level of the relevant variables in our regressions, we are fairly confident that any differences in the results would relate to the magnitude of these effects rather than their sign. 9. A number of the explanatory variables were logged. In the literature the basic growth accounting model is generally exponential (e. g. Cobb-Douglas).
Once logged, it becomes a linear relationship which can then be estimated. For the other explanatory variables in our model, logging helped to solve problems of serial correlation and heteroscedasticity. 10. The difference in parameter estimates for the regulatory index is due to the scale effect generated by the weight used in calculating the first principal component of the two indicators. 11. However, we would not wish to over-emphasise the importance of this result given the data limitations as pointed out in Kauffman et al (2005).
One could also argue that different proxies may have different dynamic effects on growth and that broader indicators of governance may require a longer period of time to produce their full effect on economic growth. 32 12. Net in this case applies to the log difference of different investment shares in GDP (physical and human in this case) and (d+n+g), where d is the rate of depreciation of capital per annum; n is the rate of population growth and g is a proxy for rate of technical change. As is the practice in the literature, (d+g) is assumed to be 5%. The specification is based on a Solow/Augmented Solow model. 3. In this model the regional dummies identify whether there are regional similarities or differences between regions. 33 REFERENCES Acemoglu D. , Johnson, S. & Robinson, J. (2000). ‘The Colonial Origins of Comparative Development’, American Economic Review, 91(5): 1369-1401 Asian Development Bank (2000). Asia Development Report, Manila: ADB. Averch, H. & Johnson, L. L. (1962). ‘Behavior of the Firm under Regulatory Constraint’, American Economic Review, 52: 1052-69. Bailey, E. E. (1973). Economic Theory of Regulatory Constraint, Lexington, DC: Heath. Barro, R. J. (1991).
Economic Growth in a cross section of countries, Quarterly Journal of Economics, 106:407-33. Barro, R. J. (1997). Determinants of Economic Growth: A cross-country empirical study, Development Discussion Paper No. 579, Harvard Institute for International Development. Barro, R. J. (2000). Inequality and growth in a panel of countries, Journal of Economic growth, 5(1): 5-32. Barro, R & Sala-i-Martin, X (1992). ‘Convergence’, Journal of Political Economy, 100: 223-51. 34 Benhabib, J. J. & Spiegel, M. M. (1994). ‘The Role of Human Capital in Economic Development: Evidence from Cross-Country Data’, Journal of Monetary
Economics, 34:. 143-73. Birdsall, N. & Nellis, J. (2002). Winners and Losers: Assessing the Distributional Impact of Privatization, Working Paper No. 6, Washington, DC. : Center for Global Development. Buchanan, J. M. (1972). Theory of Public Choice, Michigan: University of Michigan Press. Campbell-White, O. & Bhatia, A. (1998). Directions in Development: Privatization in Africa, Washington, DC: World Bank. Cave, M. & Stern, J. (1998). ‘Regulatory Institutions and Regulatory Policy for Economies in Transition’, in (ed. ) C. Robinson, Regulating Utilities: Understanding the Issues, London: Institute of Economic Affairs.
Chong, A. & Lopez-de-Silanes, F. (2002). ‘Privatization and Labor Force Restructuring around the World', World Bank Policy Research Working Paper 2884, Washington, DC: World Bank. Chong A. and Lopez-de-Silanes F. (2005) Privatization in Latin America : Myths and Reality. Stanford : Stanford University Press and World Bank 35 Clague, C. , Keefer, P. , Knack, S. & Olson, M. (1995). ‘Contract-intensive Money: Contract Enforcement, Property Rights and Economic Performance’, Working Paper No. 151, University of Maryland: Institutional Reform and the Informal Sector (IRIS). Cook, P. & Kirkpatrick, C. (2003). Assessing the Impact of Privatization in Developing Countries’, in eds. D. Parker and D. Saal, International Handbook on Privatization, Edward Elgar, Cheltenham, UK & Northampton, MA, USA. De Castro, A. S, Goldin, I & da Silva, L. A. P. (2002). ‘Relative Returns to Policy Reform: Evidence from Controlled Cross-Country Regressions’, mimeo, Washington DC: World Bank. Djankov. , S. , La Porta, R. , Lopez-de-Silanes, F. And Shleifer, A. (2002) ‘The Regulation of Entry’, Quarterly Journal of Economics, 117(1):1-37. Gray, P. (2001). ‘Private Participation in Infrastructure: A Review of the Evidence’, mimeo. Washington DC: World Bank. Grossman, S. J. & Hart, O. D. (1986). ‘The Costs and Benefits of Ownership: A Theory of Vertical and Lateral Integration’, Journal of Political Economy, 94 (4):691-719. Guasch, J. L. & Hahn, R. W. (1999). ‘The Costs and Benefits of Regulation: Implications for Developing Countries’, World Bank Research Observer, 14 (1): 137-58. 36 Hall, R. E. & Jones, C. (1999). ‘Why Do Some Countries Produce So Much More Output per Worker than Others? ’ Quarterly Journal of Economics, 114 (1): 83-116. Hayek, F. A. (1945). ‘The Use of Knowledge in Society’, American Economic Review, 35: 519-30.
Hood, C. (1994). Explaining Economic Policy Reversals, Buckingham: Open University Press. Islam, N. (1995). ‘Growth Empirics: A Panel Data Approach’, Quarterly Journal of Economics, 110: 1127-70. Jacobs S. (2004). ‘Governance of Asia Utilities; New Regulators Struggle in Difficult Environments’ The Governance Brief, issue 10, ADB Governance and Regional Cooperation Division: Bangkok. Kauffman, D. & Kraay, A. (2002). ‘Growth Without Governance’, mimeo, Washington DC: World Bank. Kauffman D. , Kraay A. & Mastruzzi M. (2005). ‘Governance Matters IV: Governance Indicators for 1996-2004’ World Bank, May 7 Kirkpatrick, C. & Parker, D. (2004). ‘Regulatory Impact Assessment and Regulatory Governance in Developing Countries’ Public Administration and Development 24,4: 333-344 Kirkpatrick, C. & Parker, D. (2005). ‘The Impact of Privatization in Developing Countries: A Review of the Evidence and the Policy Lessons’, Journal of Development Studies, vol. 41, no. 4, pp513-41. Kirkpatrick C. , Parker D. , & Zhang Y-F. (forthcoming). ‘Foreign Direct Investment in Infrastructure: Does Regulation Make a Difference? ’ Transnational Corporations. Knight-John, M. (2002). The Institutional Policy Framework for Regulation and Competition in Sri Lanka’, Working Paper No. 40, Centre on Regulation and Competition, Institute for Development Policy and Management, University of Manchester. Laffont J-J (1999a) ‘Competition, Regulation and Development’ in B. Plescovic and j. Stiglitz (eds. ) Annual World Bank Conference on Development Economics 1998, Washington DC: World Bank, 237-257 Laffont, J. -J. (1999b). Incentives and the Political Economy of Regulation, Oxford: Oxford University Press. Laffont J-J (2005) Regulation and Development, Cambridge: Cambridge University Press Laffont, J. -J. Tirole, J. (1993). A Theory of Incentives in Procurement and Regulation, Cambridge, Mass. : MIT Press. 38 Laffont, J. -J. & Tirole, J. (2000). Competition in Telecommunications, Cambridge, Mass. : MIT Press. Lanyi, A. (2000). ‘The Institutional Basis of Economic Reforms’, in eds. S. Kahkonen and A. Lanyi, Institutions, Incentives and Economic Reforms in India, New Delhi and London: Sage Publications. Levy, B. & Spiller, P. T. (1994). ‘The Institutional Foundations of Regulatory Commitment: A Comparative Analysis of Telecommunications Regulation’, Journal of Law, Economics and Organization, 10 (2): 201-46. Lucas, R. E. (1988). On mechanism of economic planning’ Journal of Monetary Economics, 21 (1): 3-42. Majone, G. (1994). ‘The Emergence of the Regulatory State in Europe’, West European Politics, . 17: 77-101. Majone, G. (1997). ‘From the Positive to the Regulatory State’, Journal of Public Policy, 17 (2): 139-67. Mankiw, N. D. , Romer P & D. Weil (1992). ‘A Contribution to the Empirics of Economic Growth’, Quarterly Journal of Economics, 107: 407-37. 39 Minogue M. (2005). Apples and Oranges – Comparing International Experiences in Regulatory Reform, Occasional Lecture 13, Bath: Centre for the Study of Regulated Industries, University of Bath
Neumayer, E. (2002). ‘Is Good Governance Rewarded? A Cross-National Analysis of Debt Forgiveness’, World Development, 30 (6): 913-30. Newbery, D. (1999). Privatization, Restructuring and Regulation of Network Industries, Cambridge, Mass. : MIT Press. Nonneman, W. & Vanhoudt, P (1996). ‘A Further Augmentation of the Solow Model and the Empirics of Economic Growth for OECD Countries’, Quarterly Journal of Economics, 111: 943-53. North, D. C. (1990). Institutions, Institutional Change and Economic Performance, Cambridge: Cambridge University Press. Olson, M. , Sarna, N. & Swamy, A. V. (1998). Governance and Growth: A simple hypothesis explaining cross-country differences in productivity’, mimeo, Centre for Institutional Reform and Informal Sector (IRIS), University of Maryland. Parker, D. (1999). ‘Regulation of privati
Cite this Page
A Cross-Country Analysis. (2017, Apr 29). Retrieved from https://phdessay.com/the-impact-of-regulation-on-economic-growth-in-developing-countries-a-cross-country-analysis/
Run a free check or have your essay done for you
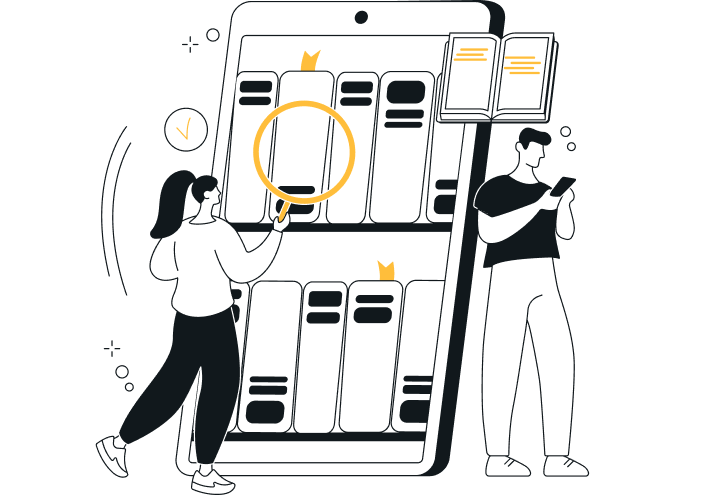