Enterprise Data Management
Master Data Management is one of the enduring technologies which define the process of reading and managing organization's data with reliability and accuracy and store it in the form of master data. Nowadays master data management Is widely practiced in most of the organization due to its significant features and for performing smooth business process. The other topic is Big Data which is the budding concept that defines the size or volume. Big data is a collection of all sorts of data (both structured and unstructured), which are huge in size and brings complexity in managing IT. Big data is expected to bring great issues in future to all enterprise which handles huge tat so many researches and development are conducted on this concept.
2. Critical review on Master Data Management
2. 1 . Summary of master data management
Order custom essay Enterprise Data Management with free plagiarism report
Master Data Management (MD) is an ongoing matured technology or concepts which convinces and resolve more IT and business oriented issues by supporting and maintaining business oriented database which is the Master data. Master data is simply the asset of each organization which provides a single view to Its users throughout the ERP system of an organization.
Risotto et al. , (201 ) describes master data as "business-oriented properties of data objects which are used in the different applications across the organization, together with their associated metadata, attributes, definitions, roles, connections and taxonomies". The contents of master data are more frequently explained by most of the experts as Customer master data, Item Master data, Account Master, finance master and so on. They are maintained separately based on each business functions.
Master data contents are normally classifieds with basic entities of business like people, items, location and concepts. These basic entitles are further classified as domain area or subject area, For example people: can be classified as customer, supplier, and employee. Therefore master data forms a tree like data architecture which suite well to navigate, find and replace data items. Finally master data differs from other kinds of data such as transactional data, meta data, unstructured data or hierarchical data by Its key features which are ambiguousness and uniqueness of data across the organization. At times master data also pretend to act as a transactional data based on the organization and its business needs, but never loses its credibility. MD Is one of the key feature of Enterprise Data Management domain that integrates IT and business process to attain business needs and business 1 OFF deals with master data. (Taps Kumar and Nanas Raman, 2011) defines Master Data Management (MD) as a combination of technology, tools, and processes required to create master data, clean master data and maintain consistency and accuracy on lists of master data.
So the main functions of MD which are performed on the organization's data assets are to create a master data with assurance to data quality, cleanses unwanted data by maintaining data free from replication, Store the master tat, synchronize the master data with other application or business process or analytical tools, support decision making for the practitioners and finally the other important function of MD to maintain master data architecture as well.
Master data architecture is the basic design constructed for master data management system to control rapid data access, avoid duplication of data and determine the flow of data within the architecture. These all together comprises provide data quality. The MD so far discussed are the first generation MD addressed by Garner. (Ted, 2011) and e also mentions about the second generation MD which is multi-domain MD technologies that deals with most complex data standards across different domains. 2. 2. Challenges of master data management MD is widely used in most of the organizations today.
But implementing MD and working of MD after implementation has more challenges. A survey conducted by (Taps Kumar and Nanas Raman, 2011) has come with few of the challenges for Master Data Management. They are, Duplicate Acid's (Customer Data 'd) were found during customer data integration with customer master data. This may cause the poor data quality. Different kinds of application are used on a enterprise information system so MD finds hectic to integrate those application based files as there is no proper interface defined.
And it is difficult to develop such interface. Data transfer between source and destination involved with MD makes it in slower rate due to mapping issues, Overall data integration process carried out by MD is unproductive. As it produces poor results. MD cannot able to tackle huge amount of data.
3. Critical review on Big Data
3. 1 . Summary of the Big Data
As mentioned in introduction part big data is newly emerged concept which heartens the enterprises that handles more than few thousand terabytes of data.
Big data concept is framed with three dimensions namely volume, velocity and variety which are commonly known as vs.. Madden (2012) came up with a simplified definition for big data that is "too big, too fast and too hard for the current enterprise data management systems to handle with big data" (Madden 2012). Too big here represents the amount of data or total size of data been handled by a normal data management system. In recent trend the data has exceeded from few thousand terabyte to few appetite and also refers to high volume in the vs..
Next is too fast which represents the velocity of vs.. It denotes the rate at which data enters into the database or warehouse or simply data management system. The contradiction with velocity is the data arrival rate can be too slow and also too fast which comes with both extent (Ted, 2011). This may create some issue while developing a strategy or tool to handle big data. Finally the "too hard" refers to variety among vs. which notify more such attributes for representing data has been widely used because of technological advancement and lack of standardization of data formats.
The major reasons for such a high volume of data is obtained through
- Streaming data for videos, audios, images, texts and other files that are available in internet,
- Process transaction histories mainly in banking sectors and other transaction related domains,
- Authentication login/logout histories (Madden 2012). All these three can be comprised under enterprise system data assets in addition to these; large amount of data generation is due to
- Social networking sites which holds huge personal data of people.
- Data created for electronic device compatibility I.
As generation passes, technology has made things comfortable like desktop is moved by laptops then tablets and now Pads so same data is reinvented to compile with each kind of devices (Ted, 2011). Garner has discovered 12 dimensions including these three dimensions velocity, variety and volume to completely describe a data (Logan, 2012)). Garner clients have categorized the 12 dimensions under three sub headings and each with four dimensions. They are
1 . Quantification describes the measure of data based on the four dimensions volume, velocity, variety and complexity.
2. Access ennoblement and control describes how well the data fit or integrate with the data architecture/ framework/ technology. This is assessed by four dimensions namely Classification, Contracts, Pervasiveness and Technology-ennoblement.
3. Qualification and assurance deals with the data quality and standards under four dimensions
- Fidelity
- Linked data
- Validation of data
- Permissibility (Mark A. Et al. , 2011).
3. 2. Challenges and impacts of Big Data
Out of all dimensions, volume is the only dimension concentrated by most vendors and more researches were held to solve the big data issue for high volume.
Unprepared is a framework or model developed by Google to handle its large data sets which are distributed everywhere. Unprepared has two functions map: to find the related data in the distributed systems using linked list data structures and the other function reduce: combines these linked list data as single cluster. Based on this concept Apache developed hoodoo software framework which could handle appetite of data but it is modeled to work only with HIDES Hoodoo Distributed File System.
These two techniques served as initiator or even served as a basement for other vendor to find a solution for big data issue. It is more expected to others vendors and serves as basement for other technologies or domain like business intelligence and EDM to implement this concept and find solution for big data issue. Vendors like Microsoft, oracle and more others are funding a lot on this issue and also motivate researchers by providing requirements and environment to undergo their research to develop a new data strategy that handles large datasets.
But there are few challenges associated in finding solution for big data. They are firstly most of the researches on progress were based on big data volume analytics but Garner has omen with 12 dimensions which has to considered and develop a solution for big data issue in all these dimensional aspects. This is going to be the future information management strategy. Secondly, the new information management strategy should be able to integrate with existing information management technologies.
Finally the new information strategy should be cost effective in implementing and low in risk greenroom combine to utilize Unprepared technique for accessing data in business intelligence domain. Similarly MM, the only vendor made a separate platform to resolve or to address the big data issue. The reason for creating big data with a separate platform is to accept existing technology and to adopt with newly emerging technology. (Taylor, 2011) The concept of big data is matured and extreme improvements are found, which on controversy brings severe Impacts too.
It is expected that once after resolving the big data issue the current Enterprise Information Management (MIM) will be replaced by the new data strategy, Extreme Information Management (MIM). The impacts are
- Organization seeks for highly skilled employee for the handling with new data standards and new technology extreme information management,
- Confusion arises while doing data integration process regarding which data format to be used,
- Existing hardware, software will be forced to adopt or make compatible to new data strategy,
- Increased competition pressures organization to invest on new technologies and finally standardization of new data strategy or extreme information management (Howard, 2012).
So the Coo's and Coo's of organizations are advised to bring awareness about extreme information management and start training staffs on this technology (Mark A. Et al. , 2011).
4. Conclusion
Master data management is very slowly moving towards region of plateau of Garter's hype cycle because the second generation MD technologies researches are slow in progress.
Cite this Page
Enterprise Data Management. (2018, Jul 26). Retrieved from https://phdessay.com/enterprise-data-management/
Run a free check or have your essay done for you
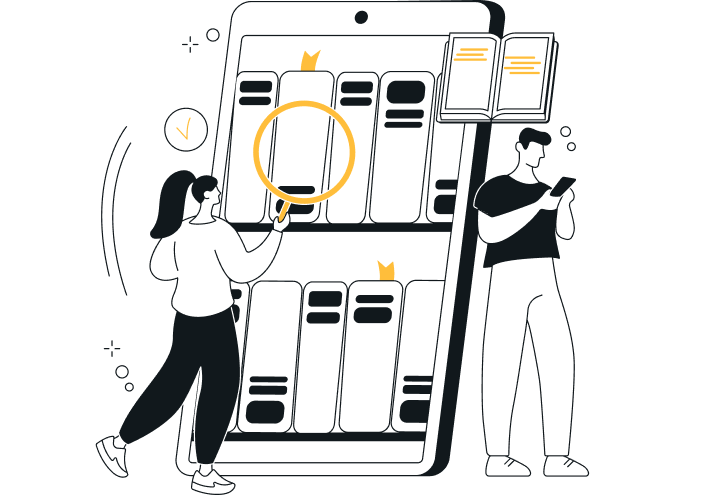