Data mining crime of data
1 INTRODUCTION
1.1MOTIVATION AND BACKGROUND
In the society crime issue is very important. It is common knowledge within the society that crime induces vast psychological, human and economical damages to individuals, environment and the economy of a particular society itself. It is very important that a society through its government, judiciary and legislative endeavours to control crime within their environment. Data mining is a brawny technique with expectant potency to help criminal investigators concentrate on the most important information in their crime data [1]. The cognition discovered from existing data goes to reveal a value added of its information. Successful data mining methodology depends intemperately on the peculiar selection of techniques employed by analyst. Their pragmatic applications are, for example, the criminal detectives, market sale forecasting and playing behaviour analysis in sport games. However the more the data and more composite question being treated and maintained, the more potent the system is required. This includes the potentiality of the system to analyze large quantity of data and composite information from various sources. In crime control of the law enforcement, there are many storage data and formats have to be revealed. When its amount has risen, it is hard to analyze and explore some new knowledge from them. Therefore, the data mining is employed to crime control and criminal curtailment by using frequency happening and length method under which these presumptions can be achieved. All these techniques give outcome to benefit detectives in searching behavioural practices of professional criminals. [1] The application in the law enforcement from data analysis can be categorised into two vital component, crime check and criminal curtailment. Crime check tends to use knowledge from the analysed data to control and prevent the happening of crime, while the criminal curtailment tries to arrest criminal by using his/her account records in data mining. Brown [2] has built a software model for mining data in order to arrest professional criminals. They suggested an information system that can be used to apprehend criminals in their own area or regions. The software can be employed to turn data into useful information with two technologies, data fusion and data mining. Data fusion deals fuses and translates information from multiple sources, and it overcomes confusion from conflict reports and cluttered or noisy backgrounds. Data mining is concerned with the automatic discovery of patterns and relationships in large databases. His software is called ReCAP(Regional Crime Analysis Program), which was built to provide crime analysis with both technologies (). When the terrorism was burst by 9/11 attacks, fears about national security has risen significantly and the world has varied forever. The strategy against a terrorist must be more advanced in order to prevent suicide attacks from their stratagem [5]. In the congressional conference, Robert S. Mueller – The Director of investigative department of FBI, suggested that they excessively emphasize to arrest the criminals with slightly attention for crime checks is the main problem of the law enforcement in the world [4]. It is more interesting now in data collection for criminal control plan. Abraham et. al. [1] suggested a method to use computer log register as account data search, some relationship by employing the frequency happening of incidents. Then, they analyze the outcome of produced profiles. The profiles could be employed to comprehend the behaviour of criminal. It should be observe that the types of crime could be exchanged over time determined by the variation of globalization and technology. Therefore, if we want to prevent crime efficiently, the behaviour must be employed with another kind of knowledge. We need to know the crime causes. de Bruin et. al. [3] introduced a new distance standard for comparing all individuals established on their profiles and then clustering them accordingly. This method concedes a visual clustering of criminal career and changes the identification of categories of criminals. They present the applicability of the data mining in the area of criminal career analysis. Four important elements play a role in the analysis of criminal career: crime nature, frequency, duration and severity. They also develop a particular distance standard to combine this profile difference with crime frequency and vary of criminal behaviour over time. The matrix was made that describe the number of variation in criminal careers between all couples of culprits. The data analysis can be employed to determine the trends of criminal careers. Nath[6] suggested a method for data division in order to use them present in the pattern of geographic map. We could decide the data division to be the veer of offend across many fields.
Order custom essay Data mining crime of data with free plagiarism report
1.2 PROBLEM DEFINITION
The report of the headline findings represents the 2006 Offending, crime and justice survey (OCJS). This gives description of style and degrees in youth offending anti-social behaviour (ASB) and victimisation amongst youth between the ages of 10-25 residing in a private household in England and Wales. Couple of years now data are obtained from respondent representatives’ interview 4,952 including 4,152 panel members and 799 fresh samples on the frequency consequences and characteristics of offender’s victimization in England and Wales. This survey enables the Offending, crime and justice survey (OCJS) to forecast the probability of specified outcome of victimization by assault, rape, theft, robbery, burglary, sexual assault, vehicle related theft, drug selling, for the population as a whole. The OCJS provides the largest forum in England and Wales for victims and offenders to describe the impact of crime and characteristics of offenders.
1.3 PROJECT GOAL
This project aim to identify which of the data mining technique best suit the OCJS data.
Identify underlying classes of offenders.
1.4 GENERAL APPROACH
Data mining analysis has the tendency to work from the data up and the best techniques are those developed with a preference for large amount of data, making use of as much of the gathered data as potential to arrive at a reliable decision and conclusion. The analysis procedure starts with a set of data, employs a methodology to develop an optimal delegacy of the structure of the data during which time knowledge is gained. Once knowledge has been gained this can be broadened to large sets of data working on the effrontery that the larger the data set has a structure similar to the sample data. Again this is analogous to a mining process where large numbers of low grade materials are sieved through in order to find something of value.
Target Data
Figure 1.1 Stages/Procedures identified in data mining adapted from [4]
1.5 ORGANISATION OF DOCUMENT
The remainder of this report is as follow: Chapter 2 reviews the approach to data mining and also described the mining techniques. Chapter 3 introduced the basic theory for the algorithm. Chapter 4 described the adopted method. Chapter 5 presented the application and a discussion of the result.
2 LITERATURE REVIEW
2.1 INTRODUCTIO
The major reason that data mining has pulled a big deal of attention in information industry in recent years is due to the broad accessibility of vast amount of data and the impending need for turning such data into useful information and knowledge. The information and knowledge acquired can be employed for application ranging from business management, production control, and market analysis, to engineering design and science exploration. [4] Having focused so much attention on the collection of data the problem was what to do with this valuable resourceIt was distinguished that information is at the centre of business operations and that decision makers could make use of the data stored to acquire valuable insight into business. Database management systems gave access to the data stored but this was only a small part of what could be acquired from the data. Traditional online transaction processing systems, OLTPs, are good at putting data into database quickly, safely and efficiently but are not good at delivering meaningful analysis in return. Analyzing data can provide further knowledge about a business by going beyond the data explicitly stored to derive knowledge about the business. This is where data mining or knowledge discovery in database (KDD) has obvious benefit for any enterprise. [7]
2.2 What is Data mining?
Data mining is concerned with extracting or “mining” knowledge from large amount of data. The term is really misnomer. Recall that the mining of gold from rocks and sand is concerned with gold mining rather than sand and rocks mining. Thus “data mining” should have been more suitably named “knowledge mining from data”, which is unfortunately so long. “Knowledge mining” a shorter term might not show the emphasis on mining from large amount of data.[4,6] Nevertheless, mining is a bright term characterising the procedure that discovers a small set of treasured pearl from a large conduct of raw materials (figure 1). Thus, such
Fig.2.1 data mining-searching for knowledge (interesting patterns) in your data. [4]
Misnomer which contains both “data” and “mining” became a big choice. There are many other terms containing a similar or slightly dissimilar meaning to data mining, such as data archaeology, knowledge extraction, data/ pattern analysis, and data dredging knowledge mining from database. Lots of people treat data mining as an equivalent word for another popular used condition, “knowledge discovery in database” or KDD. Alternatively, others regard data mining as just an essential step in the procedure of knowledge discovery in database. [2, 4] Knowledge discovery as a process is described in fig.2 below and comprises of an iterative sequence of the following steps:
Data cleaning (removal of noise or irrelevant data)
Data integration (where product data source may be mixed)
Data selection (where data applicable to the analysis task are recovered from the database)
Data transformation (where data are translated or fused into forms appropriate for mining by doing summary or collection operations, for instance)
Data mining (an essential procedure where intelligent methods are used in other to extract data forms or patterns),
Pattern evaluation (to discover the truly concerning forms or patterns representing knowledge based on interest measure)
Knowledge representation (where visualization and knowledge representation proficiencies are used to deliver the mined knowledge to the user)
Fig.2.2 Data mining as a process of knowledge discovery adapted from [4, 7]
The data mining steps may interact with the user or a knowledge base. The interesting patterns are presented to the user, and may be stored as new knowledge in the knowledge base. It is very important to note that according to this view, data mining is only one step in the entire process, albeit an essential one since it uncovers the hidden patterns for evaluation. Adopting a broad view of data mining functionality, data mining is the process of discovery interesting knowledge from large amount of data stored either in database, data warehouse, or other information repositories. Based on this view, the architecture of a typical data mining system may have the following major components:
(1) Data warehouse, database, or other information repository. This is one or a set of database, data warehouse, spread sheets, or other kind f information repositories. Data cleaning and data integration techniques may be performed on the data.
(2) Database or data warehouse server. The database or data warehouse server is responsible for fetching the relevant data, base on the user’s data mining request.
(3) Knowledge base. This is the domain knowledge that is used to guide the search, or evaluate the interestingness of resulting patterns. Such knowledge can include concept hierarchies, used to organize attributes or attributes values into different level of abstraction. Knowledge such as user beliefs, which can be used to assess a pattern’s interestingness based on its unexpectedness, may also be included. Other examples of domain knowledge are additional interestingness constraints or thresholds, and metadata (e.g., describing data from multiple heterogeneous sources).
(4Data mining engine. This is essential to data mining system and ideally consists of a set of functional module for tasks such as characterisation, association analysis, classification, evaluation and deviation analysis.
(5)Pattern evaluation module. This component typically employs interestingness measure and interacts with the data mining modules so as to focus the search towards interesting patterns. It may access interestingness threshold stored in the knowledge base. Alternatively the pattern evaluation module may be integrated with the mining module, depending on the implementation of the data mining method used. For efficient data mining, it is highly recommended to push evaluation of patterns interestingness as deep as possible into the mining process so as to confine the search to only the interesting patterns.
(6)Graphical user interface. This module communicate between the user and the data mining system, allowing the user to interact with the system by specifying a data mining query or task, providing information to help focus the search, and performing exploratory data mining based on the intermediate data mining results. In evaluate mined patterns, and visualize the pattern in different forms.[4, 6, 7]
The quantity of data continues to increase at a tremendous rate even though the data stores are already huge. The common problem is how to make the database a competitive job advantage by changing apparently meaningless data into useful information. How this challenge is satisfied is vital because institutions are increasingly banking on efficient analysis of the data simply to remain competitive. A variety of new techniques and technology is rising to assist sort through the information and discover useful competitive data. By knowledge discovery in databases, interesting knowledge, regularities, or high-ranking information can be elicited from the applicable set of information in databases and be looked-into from different angles; large databases thereby assist as ample and dependable source for knowledge generation and confirmations. Mining information and knowledge from large database has been recognized by many research workers as a key research subject in database systems and machine learning. Institutions in many industries also take knowledge discovery as an important area with a chance of major revenue. [8] The discovered knowledge can be applied to information management, query processing, decision making, process control, and many other applications.
From data warehouse view, data mining can be considered as an advance stage of on-line analytical processing (OLAP). However, data mining extends far beyond the constrict measure summarization mode analytical processing of data warehousing systems by integrating more advance techniques for information understanding [6, 8]. Many individuals treat data mining as an equivalent word for another popularly applied condition, Knowledge Discovery in Databases, or KDD. Alternatively, others view data mining as simply an indispensable measure in the process of knowledge discovery in databases.
For example, the KDD process as follow:
Learning the application domain
Creating a dataset
Data cleaning and pre-processing
Data reduction and projection
Choosing the function of data mining
Choosing the data mining algorithm(s)
Data mining
Interpretation
Using the discovery knowledge
As the KDD process shows, data mining is the fundamental of knowledge discovering, it needs elaborated data training works. Data cleaning and pre-processing: includes basic operations such as removing noise or outliers, gathering the necessary data to model or account for noise, resolving on strategies for dealing with missing data fields, and accounting for time sequence data and recognised changes, as well as settling DBMS issues, such as mapping of missing and unknown values, information type, and outline. Useful data are selected from the arranged data to increase the potency and focus on the job.
After data preparation, selecting the purpose of data mining determine the aim of the model gained by data mining algorithm (e.g. clustering, classification and summarization). Selecting the data mining algorithm includes choosing method(s) to be used for researching for patterns in the data, such as determining which models and parameters many are captured and corresponding to a particular data mining method with the overall standards of the KDD process. Data mining explores for patterns concern in a particular realistic form set of such representations; including classification rules, or clustering, regression, sequence modelling, trees, dependency and line analysis. The mining outcomes which correspond to the demands will be translated and mobilised, to be taken into process or be introduced to concerned companies in the last step.
For the importance of data mining in KDD process, the term data mining is turning more popular than the longer term of knowledge discovery [3, 8]. Individuals gradually conform a broad opinion of data mining functionality to be the equivalent word of KDD. The concept of data mining holds all actions and techniques using the gathered data to get inexplicit information and studying historical records to acquire valuable knowledge.
2.3 Data mining definitions
Larose [9] stipulated, data mining refers to the process of discovering meaningful new correlations, patterns and trends by sifting through large amount of data stored in repositories, using pattern recognition technologies as well as statistical and mathematical techniques. Hand et. al.[10] stated Data mining the analysis of (often large ) observational data sets to find unexpected relationship and to summarize the data in novel way that are both understandable and useful to the data owner. Peter et.al.[11] stipulated Data mining is an interdisciplinary field bringing together techniques from machine learning, pattern recognition, statistics, databases, and visualization to address the issues of information extraction from large data bases. The SAS institute (2000) defines data mining as the “process of selecting, researching and simulating huge amount of data set to reveal antecedent strange data form for business advantage. Data mining refers to as knowledge discovery in databases, meaning a process of little extraction of implicit, previously obscure and potentially useful information (such as knowledge rules, regularities, constraints) from data in databases.[12] From the business view, several data mining techniques are used to better realize user conduct, to improve the service provided, and to enhance business opportunities. Whatever the definition, data mining process differs, from statistical analysis of data. First predictive data is controlled by the need to reveal, in a well timed style, rising courses whereas statistical analysis is associated to historical information and established on observed information. Secondly statistical analysis concentrates on findings and explaining the major origin of variation in the data, in contrast, data mining strives to discover, not the apparent sources of variation, but rather the significant, although presently neglected, information. Therefore statistical analysis and data mining are complementary. Statistical analysis explains and gets rid of the major part of data variation before data mining is employed. This explains why the data warehousing tool not only stores data but also comprises and performs some statistical analysis programs. As to on-line analysis processing (OLAP) its relationship with data mining can be considered as disassociation.[9,12] OLAP is a data summarization/collection tool that assist modify data analysis, while data mining allows the automated discovery of implicit form and interesting knowledge concealed in large amount of data. OLAP tools are directed toward backing and changing interactive data analysis; while data mining tools is to automate as much of the analysis process as possible. Data mining goes one step beyond OLAP.
As noted in the former section, data mining is almost equal to KDD and they have like process. Below are the data mining processes:
Human resource identification
Problem specification
Data prospecting
Domain knowledge elicitation
Methodology identification
Data pre-processing
Pattern discovery
Knowledge post-processing
In stage 1 of data mining process, human resource identification, and the human resource should be required in the plan and their various purpose are identified. In most data mining job the human resources involved are the field experts, the data experts, and the data mining expert. In stage 2 concerned jobs are analyzed and defined. Next, data searching requires in analyzing the available data and selecting the predicting subset of data to mine. The aim of stage 4, field knowledge induction, is to extract the useful knowledge already known about the job from field experts. In stage 5, methodology identification, the most reserve mining prototypes are chosen. In stage 6, data pre-processing is depicted to transform data into the state fit for mining. Pattern discovery stage which includes the computation and knowledge discovery is talked about in section 7. The patterns found in the former stage are filtered to attract the best pattern in the last stage. [8]
Fayed et al. (1996), on the other hand suggested the following steps:
Recovering the data from large database.
Choosing the applicable subset to work with.
Resolving on reserve sampling system, cleaning the data and coping with missing domain records.
Employing applicable transformations, dimensionality simplification and projections.
Equipping models to pre-processed data.
The processes of data mining are elaborate and complicated. Many requirements should be observed on the follow of data mining, so challenges of growing data mining application are one of the crucial matters in this field. Below are the listed of challenges growth:
Dealing with different types of data.
Efficiency and measurability of data mining algorithm.
Usefulness, certainty, and quality of data mining results
Formula of several forms of data mining results.
Synergistic mining knowledge at product abstraction stages.
Mining data from different sources of information.
Security of privacy and data protection.
2.4 Data mining structure
The architecture of a distinctive data mining system may have the following major elements:
Database, data warehouse, or other data deposit
Database or data warehouse server
Knowledge base
Data mining engine
Pattern rating module
Graphical user interface
The information sources of a data mining system can be divergent information deposits like database, data warehouse, or other deposits. The database or data warehouse server is responsible for getting the applicable data to accomplish the data mining postulation. Data mining engine is the heart of data mining system. The operational module of data mining algorithms and patterns are maintained in the engine. Knowledge base stores the field knowledge that is used to lead the data mining process, and provides the data that rules evaluation module motives to formalise the results of data mining. If the mining results has passed the establishment step then they will get to user via the graphical user interface, user can interact with the system by the interface. [4, 8, 11]
2.5 Data mining methods and Techniques
Various techniques have been suggested for resolving a problem of extracting knowledge from volatile data, each of which follow different algorithm. One of the fields where information plays an important part is that of law enforcement. Obviously, the raising amount of criminal data gives rise to various problem including data storage, data warehousing and data analysis. [11] Data mining methods relate to the function cases that data mining tools provides. The abstract definition of each data mining method and the classification basis always disagree for the ease of explanation, the condition of present situation, or researcher’s scope. Association, classification, prediction, clustering are usually the common methods in different works, while the term description, summarization, sequential rules etc. Might not always be used and named in the first place. If some methods are not named it does not refer these methods are not created because the researcher may allot special term to methods to indicate certain significant characters. Progressive specification and jobs sectors can also be a good ground to consider the terminology. For example “REGRESSION” is often used to substitute “PREDICTION” because the major and conventional techniques for prediction are statistical regression. “Link analysis” can be discussed severally outlying “association” in telecommunication industry. Table 1.1 shows the method recognised by scholars:
Data mining methods comprise techniques which develop from artificial intelligence, statistics, machine learning, OLAP and so on. These most often observed methods are classed into five categories according to their work types in business applications, and the five types of data mining methods are clustering, classification, association, prediction and profiling.
Table 1 Data mining classification literatures
Sources: This research
AuthorData Mining Classification
Barry (1997)Prediction, Classification, Estimation, Clustering, Description, Affinity grouping.
Han, et al. (1996)Clustering, Association, Classification, Generalization, Similarity search, Path traversal pattern.
Fayyed, et (1996)Clustering, Regression, Classification, Summarization, Dependency modelling, Link analysis, Sequence analysis.
Association: reveals relationship or dependence between multiple things, such as Link analysis, market basket analysis, and variable dependency. Association is in two levels: quantitative and structured. The structural level of the method assigns (often in graphical form) which things are associated; the quantitative level assigns the strength of the relationship using some numerical scale. Market basket analysis is a well recognized association application; it can be executed on a retail data of customer deal to find out what item are often purchased together (also known as item sets). Apriori is a basic algorithm for finding frequent item sets. The denotation of apriori can further deal with multi-level, multi-dimensional and more composite data structure. [7]
Classification: function (or classifies) a data detail into one of several set of categorical classes. Neural network, Decision tree, and some probability advances are often used to execute this function. There are two steps to carry out classification work. In the first step, classification model is form describing a predetermined set up of classes or concepts. Second step the model is used for categorization. For example, the classification learned in the first step from the analysis of data from existing customers can be used to predict the credit evaluation of new or future customers.
Prediction: admits regression and part of time series analysis. Prediction can be regarded as the structure and use of a model to evaluate the value or value ranges of a property that a given sample is probably to have. This method functions a data item to a real-value prediction variable, and the goal of time series analysis is to model the state of the process generating the sequence or to extract and study deviation and style over time. In our opinion, the major deviation between prediction and classification is that prediction processes with continuous values while classification centres on judgements.
Clustering: functions a data item into one of various categorical classes (or clusters) in which the categories must be determined from the data different assortments in which the classes are determine. Clusters are defined by determinations of natural grouping of data detail based on similarities metrics or probability density models, and the procedure to form these groups is named as unsupervised learning to distinguish from supervised learning of classification.
Data mining techniques and methods render capable extraction of concealed predictive data from huge datasets or databases. It is a very powerful new technology with great potency to assist institutions concentrate on the important information in their database and data warehouse. Data mining instruments forecast future behaviours and trends, allowing businesses to make active, knowledge aimed decision. The automated, potential analyses proposed by data mining go beyond the analyses of previous issues provided by retrospective instruments typically for decision support systems. [2, 4, 12] Data mining instruments can respond to business question that traditionally were times consuming to conclude. They examine databases for hidden patterns, finding predictive information that experts may miss because it lies outside their expectation.
Most institutions already collect and refine large quantities of data. Data mining methods and techniques can be carried out quickly on existing hardware and software program to raise the scope of the existing information resources, and can be merged with new systems and products as they are brought on-line. When enforced on high performance client/server or parallel processing computers, data mining instrument can analyze huge databases to present answers to questions such as, which clients are most likely to answer my next promotional mailing, and why?[10, 12]
Recent progress in data collection, storage and manipulation instruments such as extraordinary storage and computational capability, use of the internet, modern surveillance equipments etc, have widen the range and limit for the same.
Moreover, the increasing dependence on high technology equipment for common man has facilitated the process of data collection. [13]
The data might be in the direct form or may not be in the direct form and might need some interpretation based on former knowledge, experience and most importantly is determined by purpose of data analyses. This job is further augmented by sheer intensity, texture of data and lack of human capabilities to deduce it in ways it is supposed to be. For this reason many computational instruments are used and are broadly named as Data mining tools. [10]
Data mining tools constitutes of basic statistics and Regression methods, Decision trees, ANOVA and rule based techniques and more importantly advanced algorithm that uses neural networks and Artificial Intelligence techniques. The applications of data mining tools are limitless and basically aimed by cost, time constraints, and current demand of the community, business and the government. [14]
2.6 Data mining modelling
Data mining modelling is the critical part in developing business applications. Business application, such as “cross selling”, will be turn into one or more of business problems, and the goal of modelling is to formulate these business problems as a data mining task. For example, in cross selling application, the association in the product area is determine, and then customers will be classified into several clusters to see which product mix can be matched to what customers.
To know which data mining task is most suitable for current problem, the analysis and understanding of data mining task’s characters and steps is needed. Data mining algorithm consists largely of some specific mix of three components.
The model: There are two relevant factors: the function of the model (e.g., clustering and classification) and the representational form of the model (e.g., a linear function of multiple variables and a Gaussian probability density function). A model contains parameters that are to be determined from the data.
The preference criterion: A basis preference of one model or set of parameters over another, depending on given data. The criterion is usually some form of goodness-of-fit function of the model to the data, perhaps tempered by a smoothing term to avoid over fitting, or generating a model with too many degrees of freedom to be constrained by the given data.
The search algorithm: The specification of an algorithm for finding particular models and parameters, given data, a model (or family of models), and a preference criterion.
The choice of what data mining techniques to apply at a given point in the knowledge discovery processes depends on the particular data mining task to be accomplished and on the data available for analysis. The requirement of the task dedicate to the function f data mining, and the detailed characteristics of the tasks influence the feasibility between mining methods and business problems. The so called detail characteristic includes data types, parameter varieties, hybrid approaches and so on. Slightly difference in the model will cause enormous performance change, so modelling stage effects the quality of data mining tools heavily.
REFERENCE
[1] T. Abraham and O. de Vel, “Investigative profiling with computer forensic log data and association rules,” in Proceedings of the IEEE
International Conference on Data Mining (ICDM’02), pp. 11 – 18,2006.
[2] D.E. Brown, “The regional crime analysis program (RECAP): A frame work for mining data to catch criminals,” in Proceedings of the
IEEE International Conference on Systems, Man, and Cybernetics,Vol. 3, pp. 2848-2853, 1998.
[3] J.S. de Bruin, T.K. Cocx, W.A. Kosters, J. Laros and J.N. Kok, “Data mining approaches to criminal career analysis,” in Proceedings of the Sixth International Conference on Data Mining (ICDM’06), pp.171-177, 2006.
[4] J. Han and M. Kamber, “Data Mining: Concepts and Techniques,”Morgan Kaufmann publications, pp. 1-39, 2006.
[5] J. Mena, “Investigative Data Mining for Security and Criminal Detection”, Butterworth Heinemann Press, pp. 15-16, 2003.
[6] S.V. Nath, “Crime pattern detection using data mining,” in Proceedings of the 2006 IEEE/WIC/ACM International Conference on Web Intelligence and Intelligent Agent Technology, pp. 41-44,2006.
[7] S. Nagabhushana “Data warehousing OLAP and Data mining”, published by new age international,pp251-350, 2006
[8]Takao Terano, Huan Liu, Arbee L.P. Chen (Eds.) 2000 “knowledge discovery and data mining current issues and application”
[9]Larose, Daniel T. 2005 Discovering Knowledge in data mining. An introduction to data mining (pg.3)
[10]Hand, D. J.Heikki Mannile, padhraic Smyth, 2001 Principle of data mining (pg. 1)
[11] Peter cabena, Pablo Hadjinian, Rolf stadler, Jaap verhees, and alessandro zanasi, 1998 [12]Eric D. Kolaczyk 2009 Statistical analysis of network data, method and models
Discovering data mining: from concept to implementation” (pg. 2)
[13]Trevor Hastic, Robert Tibshirani, Jerome freedman 2001 The Element of Statistical learning, data mining, inference, and Prediction
[14] An Introduction to Data Mining:
http://www.thearling.com/text/dmwhite/dmwhite.htm (Internet site accessed on 27th April 2011.)
[15] Padhye, Manoday Dhananjay “Use of Data Mining for Investigation of Crime Patterns”,
[16]Graham J. williams, simeon J. simmoff (edu).2006 Data mining: Theory, methodology, Techniques, and applications
[17]Hill Kargupta, Jiawei Han, Philip S. Yu, Rajeev Motwani and Vipin Kumar 2009 Next generation of data mining
[18]Robert G. Cowell, A. Philip David, Steffen L. Lauritzen, David J. Spieglhalter 1999 Statistics for Engineering and Information science
[19]Deepayan Sarkar, 2008 Multivariate data Visualization with R.
[20]Luis Torgo2011 data mining with R. learning with case studies
[21] Everitt, Brian and Graham Dunn 2001 “Applied multivariate data analysis”
Master’s Thesis,West VirginiaUniversity. 2006.
P. Thongtae and S. Srisuk An Analysis of Data Mining Applications in Crime Domain Computer and Information Technology Workshops, 2008. CIT Workshops 2008. IEEE 8th International Conference on
Tabachnick, Barbara G., 1936- Using multivariate statistics / Barbara G. Tabachnick, Linda S. Fidell . – 5th ed. . -Boston,Mass.;London: Pearson : Allyn and Bacon, 2007 . – 0205465250
Cite this Page
Data mining crime of data. (2019, Apr 08). Retrieved from https://phdessay.com/data-mining-crime-of-data/
Run a free check or have your essay done for you
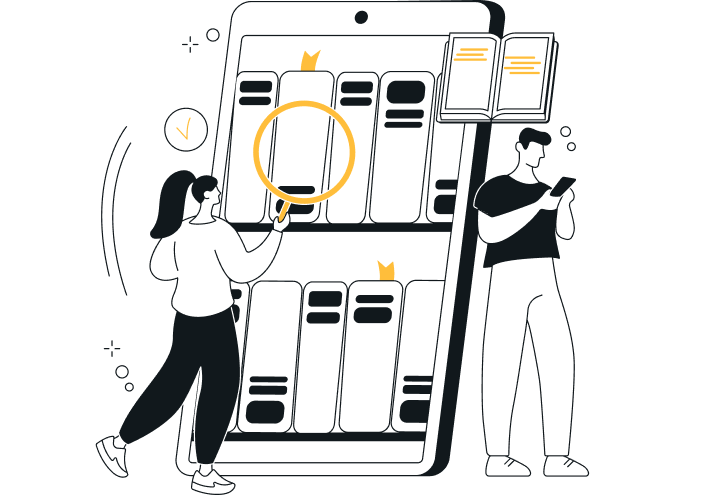