Review and Synthesis – Regression Analysis of Spatial Data
Inferential Statistics in Decision-Making - First Bi-Term
This paper presents a Statistical analysis of Spatial Data using Regression analysis (Beale, C. M.,2010). The author used synthetic but realistic data sets, including some which disregard standard regression predictions. The issue investigated by the author is concerning spatial analysis is that model fitting can include irrationally huge calculation times. The principle explanation behind this is for specific strategies; the calculation time relies upon the quantity of conceivable pairwise cooperation between focuses. The author portrays the issues that required while investigating spatial data and outline these utilizing reproduction considers. The near analysis includes strategies, including mixed models, to appraise regression coefficients from sensible data sets. Environmental reasons for this spatial autocorrelation perhaps both outward and characteristic and have been widely examined (Legendre 1993; Koenig 1999; Lennon 2000; Lichstein et al. 2002).
At first, the author considers Examples of data sets demonstrating spatial autocorrelation of both various scales and qualities and some essential exploratory data analysis to answer why space is exceptional? And infers the outcomes of correlograms for generations with comparative size of autocorrelation cross the standard line at an equivalent partition. The stature of the bend appears the quality of autocorrelation. Also, revealing insight into the Evaluation and Synthesis of Special Regression Methods utilizing Two significant outcomes of spatial autocorrelation for measurable displaying. Therefore, parameter gauges from the model fitted with Generalized Least Squares are probably going to be littler in outright extent than those from Ordinary Least Squares strategies. The reenacted blunders were typically circulated and rotted exponentially with separation, while the Generalized Least Squares technique utilized around the model for residual spatial autocorrelation.
Order custom essay Review and Synthesis – Regression Analysis of Spatial Data with free plagiarism report
Moving further towards model fitting and parameter estimation A full scope of measurable techniques are utilized in writing for fitting regression models to spatial data sets. In all situations, the covariates and blunder term have an exponential structure hidden any additional non-stationarity. R code gave in the Supporting Information gives a total depiction of everything being equal.
Additionally, Spatial analysis devices applied to every one of the 1000 recreations of eight situations. The presentation of the various techniques is then abridged, and all outcomes are given graphically regard to Distance and Moran's assigned issues with multiple hues. The plot shows mean and standard deviations from 100 reenactments of Moran's I for residuals. The mean and standard deviation of the autocorrelation structure of the reliant factors is plotted, however, that the residuals of the Wavelet Revised Models are successfully zero after the main slack. The two models are expertly fitted, despite the Generalized Least Squares fit holding residual autocorrelation.
During the time spent surveying the exhibition of spatial regression techniques, the author demonstrated that some regular discernments are mixed up. An expertly fitted spatially express model may well have autocorrelated. Thus, it infers that it is indiscreet to complete de-trending on subordinate factors alone before analysis. This work speaks to a development in a similar analysis of strategies for fitting regression models to spatial data and so gives a progressively dependable proof base to direct the decision of a technique then already accessible. Recollecting the abilities and stipulations made previously, it is dense of observational results by the different clarifications.
References
- Beale, C. M., Lennon, J. J., Yearsley, J. M., Brewer, M. J., & Elston, D. A. (2010). Regression analysis of spatial data. Ecology letters, 13(2), 246-264.
Cite this Page
Review and Synthesis – Regression Analysis of Spatial Data. (2023, Feb 14). Retrieved from https://phdessay.com/review-and-synthesis-regression-analysis-of-spatial-data/
Run a free check or have your essay done for you
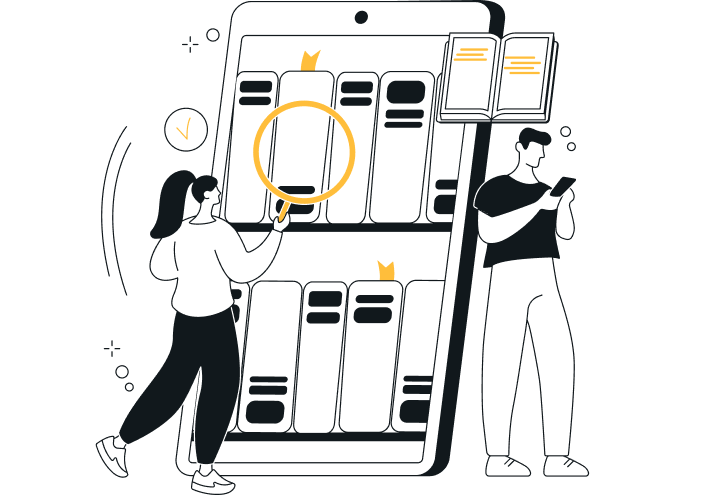