What is the Influence of alternative splicing on the topology of interaction networks?
Abstract
Motivation:
Alternative splicing is one of the main phenomena that add diversity to the proteome. Through a co-transcriptional modification of the pre-mRNA, higher organisms are able to generate much more protein products than expected by their number of genes. In this research, we attempt to study the influence of alternative splicing on the topology of the physical and genetic interaction networks in Homosapiens, Caenorhabditis elegans and Drosophila melanogaster.
Order custom essay What is the Influence of alternative splicing on the topology of interaction networks? with free plagiarism report
Result:
The analysis showed that the degree of AS and non-AS genes follow a power law distribution and there is a very small difference in the average number of interactions in the two sets of genes (i.e.: AS and non-AS). It also showed that there is no bias for alternatively spliced gene to be in a hub or to be a single node interaction. Network analysis gave an intriguing result. There are no major differences between nodes with AS and nodes without AS. In some cases after deleting random AS nodes, the diameter of the network decreased while when random non-AS nodes were deleted the diameter increased. We also found that the two classes of nodes are not randomly distributed.
Keywords:
AS – Alternative splicing.
Supplementary Data:
Supplementary data are available in a CD.
Introduction
Alternative splicing has emerged as one of the major mechanisms that add diversity to the proteome and regulate the repertoire of gene functions. It allows many gene products with different functions to be produced from a single coding sequence (Brenton et al., 2001; Brett et al., 2001). An increasing number of experiments have demonstrated the biological significance of the diversification of protein structure generated by alternative splicing (AS), which results in diversification of interactions with other bio-molecules (Stetefeld et al., 2005; Meijers et al., 2007).
Alternative splicing is a process by which the exons of the RNA, produced by transcription of a gene, are reconnected in multiple ways. After RNA splicing an exon can either be included or excluded from the final transcripts, or there can be two splice sites at one end of an exon which can be recognized by the spliceosome (the complex which carries splicing reaction). All these actions will lead to the production of multiple transcripts (Roger . et al., 1987; Douglas et al., 2003). AS changes the structure of transcripts and hence their encoded proteins (Stammet al.,2005) .
Generating multiple isoforms from a single gene makes alternative splicing a major contributor to the diverse repertoire of transcriptomes and proteomes (Hallegger et al., 2009). Protein isoforms are differently present in several tissues and developmental stages, some of them being highly specific. In addition, they can have functional differences, such as antagonistic effect, affinity variation and modification of interaction partners (Stammet al.,2005).
In this research we deal with both genetic and physical networks. A genetic network is broadly defined as an association of many genes whose members interact with one another and can affect their phenotypic activity. Furthermore, Noveen et al., (1998) refer to the interactions between genes in a network as a way of direct or indirect adjustment of gene’s expression or function. Changes in gene expression (on the mRNA or protein level) and alternative splicing can be some major reasons affecting the gene activity (Wagner,2002).
Protein interactomes are networks in which the nodes represent proteins and the edges correspond to physical interactions between them. A large amount of protein interaction data has been produced using advanced experimental and in silico methods leading to a better understanding of the cellular function at the system level (Matthews et al., 2001; Bock et al., 2001). Protein interaction networks are useful to understand the molecular basis for most cellular functions, such as metabolism, regulation and signal transduction in organisms.
In this work we consider all genetic interactions and only those physical interactions which are identified by affinity capture i.e. when a bait protein is affinity captured from cell extracts by either polyclonal antibody or epitope tag and the associated interaction partner is identified by Western blot with a specific polyclonal antibody or second epitope tag (http://thebiogrid.org/).
Alternative splice data evidently have the potential to contribute substantially to our understanding of proteomic diversity and function. It generates multiple protein isoforms from single genes and hence can be a main contributor to generate many interactions. To understand this, a systematic analysis was done by comparing features of two classes of network nodes: those having AS and those only having one isoform.
1 Materials and Methods
1.1 Genetic and Physical Interactions
Genetic and Physical interactions were downloaded from the Biogrid database (http://thebiogrid.org/download.php). All genetic interactions and only those physical interactions which were identified by affinity capture were considered for this study. In case of Drosophila both genetic and physical interactions were studied, while in case of C.elegans and Human only genetic and physical interactions respectively were considered. A total of 1096 and 984 genetic interactions for C.elegans and Drosophila, respectively; and 6246 and 416 physical interactions for human and Drosophila respectively were extracted for further analysis.
1.2 Isoforms
We considered two independent sources – one based on manually curated database (Uniprot), and another based on computational delineation of splice isoforms from EST sequences (Ensembl). Uniprot has less false positive, but has more false negatives, and vice versa for ensemble so We used two datasets because none of them is absolutely reliable.
Isoform sequence data were obtained from Uniprot in FASTA format (http://www.uniprot.org/uniprot/) and file was processed to count the number of isoforms per gene. Transcript and protein isoforms were extracted from ENSEMBL (http://www.ensembl.org/biomart/martview/ 12e358353d41d96c12dfafb68d83fe17 ). Ensembl Genes 61 database in Biomart was used for the current study. In case of Drosophila – transcripts, proteins and isoforms were studied. Transcript and isoforms were considered in case of C.elegans, while in case of human protein and isoform information was used.
A Perl script was designed to identify genes which are alternatively spliced and the ones which are not alternatively spliced. Genes which have more than one isoforms were considered to be alternatively spliced whilst the rest were not alternatively spliced. Number of AS and non-AS genes were calculated and distribution of number of interactions in AS and non-AS genes was measured.
1.3 Mapping gene names to protein interactions.
For every gene name corresponding genetic or physical interaction and the number of isoforms were identified. This mapping was performed because it is not uncommon for a particular gene to have isoforms but no annotated genetic or physical interaction. So only those genes which have annotated protein interactions were investigated further.
Average number of interactions in genes which are AS and which are not AS were analysed. Ratio of nodes which have only one interaction and nodes which are highly connected (hubs) was calculated.
1.4 Statistical analysis
Mean, standard deviation and z-scores for the number of interactions were calculated. T-test was performed on the data set to find out if the difference between the average number of interactions in AS and non-AS genes was significant or not.
To test the significance, the risk level (alpha) was set to 0.05. This means that five times out of a hundred you would find a statistically significant difference between the means of AS and non-AS genes even if there was none (i.e., 5% times the difference will be significant by chance).
Z test was done to investigate the significance of the difference between the populations of one interaction nodes which are alternatively spliced and the total number of nodes which are alternatively spliced.
A similar Z test was performed to measure the significance of the difference between the populations of hubs which are alternatively spliced and the whole population of AS nodes.
1.5 Network properties
In order to determine the diameter a script was developed. In this program, interaction networks are treated as undirected graphs in adjacency list format, and all the self-interactions are excluded. The breadth-first-search algorithm is used to compute the network’s longest shortest path length i.e. the diameter.
A set of ten randomizations were performed for AS and non-AS genes to study the diameter perturbations. In each randomization, 100 nodes were deleted in sets of ten. After deletion of every set, the network diameter was calculated.
Details of the network diameters are provided in section 3.4.
2 Results and Discussions
2.1 Degree distribution
Degree is the elementary character of a node, accounting for the number of other nodes linked to it and percentage frequency represents the percentage of nodes which have that degree.
Degree distribution can also define the type of network. For example, the Poisson distribution indicates random networks, in which the nodes are linked randomly, while the power law distribution (i.e. most of the genes with very less number of interactions and very few genes with high number of interactions) indicates scale-free networks (Barabasi, et al., 1999).
We see a qualitative difference in the distribution of number of interactions in genes. Most of the genes have single node interactions while there are a very less percentage of genes that have more number of interactions,(Figure 1). Degree distribution follows the power law, indicating that they are all scale-free networks.
All the graphs follow the same trend. For example, in graph (C) we observe that in Drosophila (genes with isoforms and genetic interactions) most of the AS and non – AS genes (29.52% and 35.28% respectively) have one node interaction while very few AS and non-AS genes (15.56% and 12% respectively) have 10 or more interactions i.e. a slight difference between AS and non-AS nodes.
C.elegans and Human also follow a similar distribution with most of the genes having one node interactions and very few genes which 10 or more interactions.
Figure 1: Degree distribution of interactions in the networks of three organisms has been displayed. (A) C.elegans (Genetic interaction in isoform genes);(B) Human (physical interactions in isoform genes);(C) Drosophila (Genetic interaction in isoform genes); (D) Drosophila (physical interactions in isoform genes). The node degree is represented on x axis, and the percentage of nodes with that particular degree is represented on y axis. Note that their degree distributions follow the power law, indicating that they are all scale-free networks. Here we have discussed the interactions between the genes having isoforms extracted from uniprot. Data from Ensembl also follow the same trend. (Refer supplementary data)
2.2 Average number of interactions in AS and NON -AS genes
There are no statistical differences in the average number of interactions in genes which are alternatively spliced and which are not alternatively spliced.
Figure 2 shows graphs with average number of interactions in Drosophila (physical and genetic interactions in isoform genes, graph (A) and (B) respectively), C.elegens (C) (Genetic interaction in isoform genes) and (D) Human (Physical interactions in isoform genes).
From the graphs we observe that the average number of interactions in AS genes is slightly higher than in the non – AS genes.
Figure 2: Average number of interactions in AS and non-AS genes have been displayed. (A) Drosophila (genetic interactions in isoforms);(B) Drosophila (physical interactions in isoforms);(C) C.elegen (genetic interactions in isoforms);(D) Human (physical interactions in isoforms ). Small difference between the average degree of AS and non-AS genes was observed.
T-test was done to compare the average number of interactions in AS and non-AS genes. Significance was evaluated by comparing the alpha level (set to 0.05) and the p values. If the p-value was greater than the alpha level, the null hypothesis would be retained and the difference will not be significant.
But this difference is not significant except in case of humans (average no. of interactions in genes with isoforms and physical interactions) where p value 0.309798 > 0.05. But a conclusion cannot be drawn from the obtained p value because it can be dependent on a lot of sample size related factors.
In the case of C.elegans and drosophila, similar trend is followed. A slight difference is observed in the average number of interactions in AS and non-AS genes but this difference is not significant.
An interesting point to notice here is that since there is not much difference in the average number of interactions and the degree distribution in alternatively spliced and non-alternatively spliced genes, both sets follow a similar trend.
2.3 Hubs and one interaction nodes
Ratio of genes which are AS and have one interaction nodes or are in hubs was obtained as shown in Table 1.
We used Z scores to evaluate the interaction significance. Genes which had a z score > 1.96 were considered to identify hubs.
Table 1: Table shows the ratio of alternatively spliced genes which have one interaction nodes (genetic/physical) and ratio of hubs (genetic/physical) which are alternatively spliced. isoforms were extracted from uniprot and transcript and protein isoforms from Ensembl.
To measure the significance of the difference between the populations of hubs which are alternatively spliced and the whole population of AS nodes, Z test was performed. Additionally, same test was performed for the population of alternatively spliced genes with one interaction nodes and whole population of AS nodes.
According to the z test, for all the three species the difference was found to be insignificant except for in two cases-human ratio of one node interaction in physical network of protein isoforms, and in genetic interactions of transcripts in Drosophila. These values are mentioned in bold in figure 3. But these values are found to be significant because of the sample size and will be considered insignificant only.
The insignificant difference implies that there is no bias for the alternatively spliced gene to be a single interaction node or to be in a hub.
2.4 Graph analysis
The shortest path length distribution between any two linked
nodes of the network (genetic/physical) was studied for the three species. In undirected networks, the distance between any two nodes is defined as the number of edges along the shortest path connecting them. As there are many possible paths between two nodes, the shortest path plays a special role. The longest shortest path of the network (i.e.: the shortest path to measure the distance between the farthest nodes) is defined as the diameter of one network, which essentially characterizes the network’s interconnectivity.
After performing ten randomizations changes in the path length(diameter) were obtained. In every randomization 100 random AS nodes (in sets of 10) were deleted and the diameter was calculated after deletion of every set.
Figure 4 shows how diameter changes in the ten randomizations. A very interesting observation was made that when AS gene nodes were deleted the diameter of the network slight decreased while, when non-AS gene nodes were deleted the diameter slightly increased. (See figure 3).
Figure 3: Diameter perturbation after random deletion of AS and non-AS gene nodes. Graphs (A) and (B) show the diameter perturbations in the genetic network of C.elegan (isoforms and transcripts respectively), graphs (C) shows diameter changes in genetic interaction of Drosophila(isoform genes) and graph (D) show the diameter perturbations in physical network of Drosophila(isoforms).It is observed that when AS gene nodes were deleted the diameter of the network slightly decreased while, when non-AS gene nodes were deleted the diameter slightly increased.
This result can suggest that if the diameter decreases when AS gene nodes were deleted, then there are high chances that AS gene nodes are present in the outer side of the network i.e. when these nodes are deleted the network tends to slightly shrink.
Additionally, when non-AS genes are deleted the diameter slightly increases. Suggesting that most of the non-AS genes would be present in the interiors of the network that is why when the nodes are deleted the path length tends to increase.
A similar trend was observed in the three species. Furthermore, location of the nodes can further be analysed by calculating the degree of betweenness or the degree of centrality.
Conclusion
Uncovering the factors affecting interaction networks is a starting point for understanding complex biological networks and the analysis of alternative splicing consequences has been one of the most stimulating fields of the last decade.
This analysis showed that degree of interactions in AS and non-AS genes follow a power law distribution moreover, there is very slight difference in the average number of interactions in the two sets of genes (i.e.: AS and non-AS). Concluding that AS genes and non-AS genes follow a similar trend and that there is not a major influence of alternative splicing on the topology of the network
One node interaction and hub ratios reveal that there is no bias on an alternatively spliced gene to be in a hub or to be a single node interaction.
Furthermore, topological analysis of the network gave an attention grabbing result. Diameter perturbations showed that when AS gene nodes were deleted the diameter of the network slight decreased while, when non-AS gene nodes were deleted the diameter slightly increased.
This research also confirmed that the three species follow a similar strategy.
This research, to study the influence of alternative splicing on the topology of the interaction networks, as an attempt hopefully provides a point for further research of the network architecture.
The architecture of the network can further be analysed by studying the degree of betweenness or the degree of centrality which shows how well connected a particular node is.
acknowledgements
I am grateful to Simon Lovell for his insightful comments and David Talavera for his fruitful remarks and discussions on the manuscript. I am also thankful to the Uniprot team for their support.
Funding: The author received no funding for this study.
References
Albert-Laszlo Barabasi, et al. Emergence of Scaling in Random Networks, Science 286, 509 (1999);
Andreas Wagner, Perturbation Data Estimating Coarse Gene Network Structure from Large-Scale Gene; Genome Res. 2002 12: 309-315
Brenton r. Graveley et al., Alternative splicing: increasing diversity in the proteomic world. Trends in genetics volume 17, 2001 pages 100-107
David brett et al. Alternative splicing and genome complexity. Nature genetics, 2001, 29-30
Douglas l. Black, Mechanisms of alternative pre-messenger rna splicing, Annual review of biochemistry Vol. 72: 291-336; july2003)
Joel R. Bock and David A. Gough, Predicting protein–protein interactions from primary structure , Vol. 17 no. 5 2001, Pages 455–460
Lisa R. Matthews, Philippe Vaglio, Jerome Reboul, et al., Identification of Potential Interaction Networks Using Sequence-Based Searches for Conserved Protein-Protein Interactions or ”Interologs”. Genome Res. 2001 11: 2120-2126
Martina Hallegger*, Miriam Llorian* and Christopher W. J. Smith, Mini review Alternative splicing: global insights, doi:10.1111/j.1742-4658.2009.07521.x; Department of Biochemistry, University of Cambridge, 200
Masafumi shionyu1, akihiro yamaguchi1, kazuki shinoda1, ken-ichi takahashi1 and mitiko go, as-alps: a database for analyzing the effects of alternative splicing on protein structure, interaction and network in human and mouse ,Nucleic Acids Research Volume37, D305-D309.
Meijers R, Puettmann-Holgado R, Skiniotis G, Liu JH, Walz T, Wang JH, Schmucker D, . Structural basis of Dscam isoform specificity. Nature 2007;449:487–491.
Noveen, A., Hartenstein, V. And Chuong, C.M. (1998). Gene networks and supernetworks: Evolutionary conserved gene interactions. In Chuong C.M. ed ., Molecular Basis of Epithelial Appendage Morphogenesis, Landes Bioscience, Austin. Pp 371-391.
Roger e. Breitbart, athena andreadis, and bernardo nadal-ginard; Alternative splicing: a ubiquitous mechanism for the generation of multiple protein isoforms from single genes;ann. Rev. Biochem. 1987. 56:467-95
Stefan Stamm, Shani Ben-Ari, Ilona Rafalska, Yesheng Tang, Zhaiyi Zhang, Debra Toiber, T.A. Thanaraj, Hermona Soreqb; Function of alternative splicing Gene
Volume 344, 3 January 2005, Pages 1-20
Stetefeld J, Ruegg MA, Structural and functional diversity generated by alternative mrna splicing. Trends Biochem. Sci. 2005;30:515–521.
Cite this Page
What is the Influence of alternative splicing on the topology of interaction networks?. (2019, Mar 18). Retrieved from https://phdessay.com/what-is-the-influence-of-alternative-splicing-on-the-topology-of-interaction-networks/
Run a free check or have your essay done for you
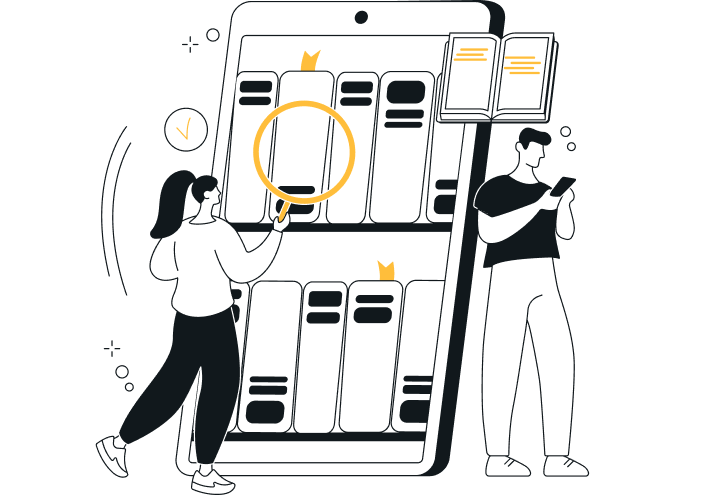