Non Linear Regression Of The Quadratic Form
We can however cross check the presence of any pattern repeat with the help of correlation between the raw data and the lagged data to ascertain any pattern repeat. The largest value in correlation we see is that of lag 4 which is 0. 843. Though this is not a sufficient big number to conclude a four point pattern repeat, based on the graphs given above, we can assume the presence of a four point pattern repeat. To make the pattern repeat clearer, we difference the data once and plot a line graph. The above graph makes the four point pattern repeat clearer after the trend has been removed.
The above ACF and the PACF plots also suggest complementary results. There is no straight line edge in the ACF and the data dissipates slowly which suggest a short term correlation. The PACF plot also indicates the presence of a small four point pattern but as the data is random, the pattern is not highly evident. First of all for defining the dates, Data-->Define Dates option is chosen from the SPSS menu and 1994 and 1st quarter are entered as per as the inputs. For the dummy variable model we take variables D1, D2 and D3 as the dummy variables along with the variable time for building the model.
The predicted model will be Investment = a + b1*D1 + b2*D2 + b3*D3 + c*time + Error Essentially due to the seasonal factors present in the data, we need to perform the seasonal decomposition procedure to find out the SAS(Seasonally adjusted series) We choose Analyse-->Time series -->Seasonal decomposition from the SPSS menu. And choose a multiplicative model type. Four new variables are created . Sequence plot of the SAS will be like given below. Now using the SAS data, we can perform a Linear regression analysis, which includes the three dummy variables D1, D2 and D3 for building the model.
Order custom essay Non Linear Regression Of The Quadratic Form with free plagiarism report
We have to predict the holdback data by applying the above model. After applying the function Transform-->Compute in SPSS, and entering the obtained model , the predicted model is created. The resultant predicted variables are very close to the output produced by SPSS which suggests that the predicted model is correct. To perform an regression analysis of the dummy variable model with an non linear trend , we just have to repeat the earlier steps of the dummy variable model estimation followed by the command Analyze-->Regression-->Non - Linear
But when we are considering a quadratic model, we have to first create a variable using the time series function . After creating the variable we perform a non linear regression model on the data, using the three dummy variables. The output is as given below. So by looking at the two regression models that we have got we can see that significance factors and the adjusted R square being better for the quadratic data. So i choose the quadratic regression model be the final dummy variable model. Q3 Box-Jenkins ARIMA model.
A Box - Jenkins model involves the analysis of the time series plot , ACF and the PACF plots of the raw data and first order differenced data. As we have seen from our earlier analysis about the time series plot we can seemingly conclude that there is no presence of trend, but were faint seasonal factors present in the data. The time series plots is given below To estimate the ARIMA parameters, the trend and the seasonal patterns are to be removed from the data. For that the following two steps are made 1) For the raw data the plots are seasonally decomposed , which gives the SAS variable.
The seasonally adjusted data is devoid of any seasonal patterns. 2) Then the Seasonally adjusted data, is differenced once to remove the trend presence and the final sequence will be devoid of any seasonal patterns, or trend or cycles After these two major operations are made the ARIMA parameters are estimated from the ACF and the PACF plots. The time series plots of the SAS data is as given below Looking at the plot we can see that seasonal factors are completely withdrawn. But then there are small presence of trend and the cyclical factors, which we can remove by differencing the SAS data once.
The time series plots of the differenced SAS data is given below. The differenced time series plot clearly shows us the removal of the trend, seasonal,and cyclical pattern which can be used to make the ARIMA model now. The ACF and the PACF plots of the differenced deseasonalised data are From the above ACF graph we can see a diminishing sine series wave, so we can say the ACF graph comes down to zero, so we need not difference the data once more . Looking at the PACF graph we can see a spike at lag 3 of the data, and then the rest of the data fall correspondingly within the confidence limits.
So it could be a ARIMA(3,1,0) model for the differenced data. Or also we can fit an ARIMA(3,1,1) for the data. We can only fit and compare the models based on a lower BIC value that can be enabled from the statistics tab of the ARIMA modeller in SPSS. After seeing the normalized BIC for both the models seems to be lesser in the ARIMA(3,1,1) model , which seems to be acceptable. This model has been chosen as the best amongst all the methods by a trial and error basis. The holdback data also comes out neatly as predicted against the forecast data as indicated by the line graph.
Also all the variables are within the 5% significance limit. The rest of the models are ignored but the results are separately annotated. This equation accommodated the dummy variables D1, D2 and D3 which were entered manually in the SPSS data sheet. After which the models were predicted. Based on the fit of the data, indicated by the Adjusted R square methods , a linear regression is factored. The linear regression returned a satisfactory fit with an adjusted R square of around 39. 2% which was of a good fit, but was due for a better replacement model.
So a non linear regression of the quadratic form yielded a model with a better fir for the data given. The adjusted R square was 71. 1%. So I took the quadratic form as my final model. Of course, if a regression of the cubic form could have yielded better results but the possibility was explored but the final results are not documented. Predicting the holdback data, was taken as the last step of the SPSS output which can be calculated by the estimating the deviation of error variable from the main data values. In effect there was not presence of any major deviations and forecasting seemed to be of a fairly accurate measure.
Cite this Page
Non Linear Regression Of The Quadratic Form. (2018, Jan 09). Retrieved from https://phdessay.com/business-forecasting-3/
Run a free check or have your essay done for you
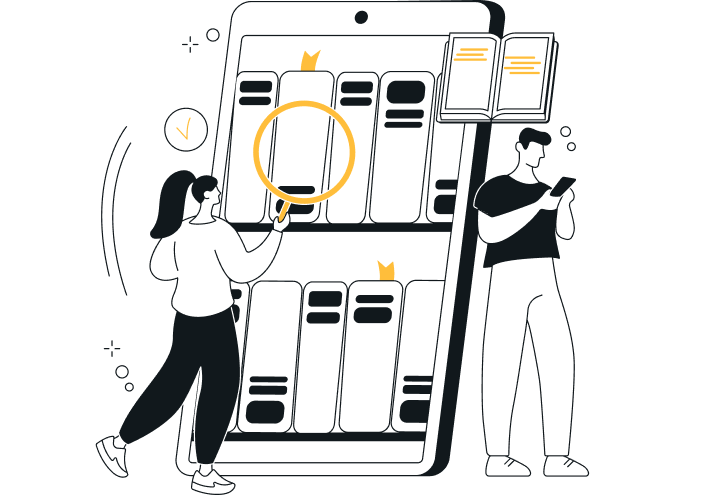