Private statistical database
Abstract
As the statistical databases consist of important and sensitive information, the preservation of the privacy in these databases is of extremely significance. Despite the complexity of the statistical databases’ protection, there are diverse sorts of mechanisms which can keep out the confidential data. This report discusses methods as data perturbations, query restriction methods and differential privacy which provide privacy in the statistical databases.
Keywords: statistical databases, privacy, input perturbation, output perturbation, differential privacy.
Order custom essay Private statistical database with free plagiarism report
1. Introduction
Nowadays, there is a wide-spread access to data. Having a lot of advantages to omnipresent access of information, there is also the possibility to break the privacy of individuals. In the statistical databases, personal data with very large number of individuals is stored. The statistical databases contain multiple statistical information. They give to their users the ability to acquire this information and also to protect the privacy of individuals. However, supporting security in the statistical databases against the revealing of confidential data is complicated and ambitious task. This problem of privacy in the statistical databases has expanded in the recent years. This report will examine the main methods for providing privacy in the statistical databases.
2. Body
2.1. Definition of statistical databases
A statistical database is a set of data units which has permissive access to the statistical information connected to these data parts. The statistical database could be described as a database system which allows to its users to obtain only aggregate statistics for a subset of items introduced in the database [1]. The statistical database posses limited querying interface which is restricted to operations such as sum, count, mean, etc. The statistical database also could be defined as query responsive algorithm which permits the users to access the content of the database through statistical queries [2].
The statistical database is concerned with the multidimensional datasets and is related to the statistical summarizations of the data sets’ dimensions.
The statistical database is mainly oriented to socio-economic databases which are normally the field of statisticians. An example of statistical database is the census data which is linked to collection of information about the assessment of the population trends. Another example of statistical database is the economic database which includes statistics for the industries’ sales and income or statistics for the use and production of diverse products [3].
2.2. Privacy in statistical databases
The privacy can be described as the right to specify what type of information about individuals or items is allowed to be shared with others. The benefits from analyzing the statistical database are very significant but the release of the information from this database could cause a lot of problems, troubles and damages. Thus, one of the main aims of the statistical database is to ensure privacy of the information. To be an effective statistical database, it should protect all its records [4].
As the statistical database should provide statistical information, it should not disclose private information on the items or individuals it refers to. The releasing of a statistical data may offend the privacy rights of the individuals. Therefore, the statistical database should follow some ethical and legal behavior to defend the individuals’ records. For legal, ethical and professional grounds, the users of the statistical database are not authorized to receive special information on individual records. The statistical database should protect the sensitive information allowing its
users to get aggregate information. The restricted access should be permitted either from the point of view of the groups of people to whom this information is available or from the point of view of the certain aspects of this information. However, it is possible sometimes when statistics are correlated, the sensitive information to be
inferred. If a combination of aggregate queries is used to obtain information, we say that the information in the database is compromised and therefore the database is also compromised [5].
The main duty for the privacy of statistical database is to find appropriate methods which could ensure that no queries are sufficient to infer the values of the protected records.
2.3. Methods for providing private statistical databases
The following methods and techniques are used to secure the privacy in statistical databases.
2.3.1 Perturbation methods
There are two main perturbation methods for preserving privacy in statistical databases. The first one is the input perturbation where the primary data is randomly modified and the results are calculated based on this modified data. The second perturbation method is the output perturbation which computes the results from the queries exactly from the actual data [6]. In other words, the input perturbation is detected when the records are computed on the queries while the output perturbation is applied to the query result after computing it on the original data. The perturbation methods look for accomplishment of the masking of item or individual’s confidential information while trying to maintain the basic aggregate relationships of the statistical database. One of the main aims of these methods is to ‘conceal’ particular confidential record. It is also necessary to notice that the perturbation techniques are not encryption techniques which first modify the data, then usually send it, receive it and finally decrypt it to the original data. The primary difficulty of these methods is to assure that the introduced error is within the satisfactory limits. There is an exchange between the level of protection that could be attained and the variance of the presented perturbation.
2.3.1.1 Input perturbation
The fundamental idea behind this method is that the result which is returned by the queries is based on a perturbed data. This means that the primary data in the statistical database is not used to create query results. One side that is necessary to be taken into account is the duplicated database. This database, which is used to turn back to results, must maintain the similar statistical characteristics as the original database.
This technique introduces random noise to the confidential information and thus protects the data. Adding statistical noise in the database makes the input perturbation an important method in the enhancement of the privacy. The original database is generally changed into modified or perturbed statistical database which is afterwards accessible to the users. The input perturbation permits the users to access the necessary aggregate statistical information from the whole database when it makes changes to the original data. Therefore this process helps to protect the records [7]. The records of the database contain values that are variations of their adequate values in the true database. As a whole this method tries to minimize the severe bias in the query results by allocating the corresponding bias in the data so that it could cancel out in the huge query sets.
In the input perturbation, the data is perturbed for instance via swapping attributes or adding the random noise before this data releases the whole statistical database.
There are two well-known subcategories in the input perturbation. The probability distribution interprets the statistical database as a sample from a given data that has a certain probability distribution [1]. The main purpose is to transform the primary statistical database with a different sample which is from the same probability distribution. This input perturbation creates a substitute database from the original one. This method is also called data swapping. The second subcategory is the fixed – data perturbation where the values of the records in the statistical database are perturbed only once and for all the records. Since the perturbation process is done only once, the repeated queries have consistent and logical values. This perturbation also constructs an alternative database as the probability
distribution. This alternative database is created by changing the value of every record by a randomly produced perturbation value. The fixed – data perturbation could be applied to both numerical and categorical data.
2.3.1.2 Output perturbation
The output perturbation differs notably from the input perturbation. In the input perturbation, the data is specified by all statistical features of the database. As longs as in the output perturbation, the perturbed results are directly introduced to the users [8]. Another difference is that in the output perturbation, the problem with the bias is not as harsh as in the input perturbation. This is because the queries are based on the original values but not on the perturbed ones. The output perturbation method is based on calculation of the queries’ responses on the statistical databases. This method adds the variance to the result. The result is produced on the original database however the noise is added to the result before to return it to the users. As the noise is not added to the database, this method generates results that include less bias that the input perturbation. It is necessary to note that if the noise is random then this noise could be reduced by performing the same query over and over again. Some limitations exist. For example if there is very large number of queries to the statistical database, the amount of the noise added to the results should be also very large [9].
The output perturbation has pretty low storage and computational overhead [10]. This method is rather easy to carry out because it does not influence the query process.
The output perturbation consists of different approaches as random sample queries, varying – output perturbation and rounding. The random sample queries technique shows a technique where a sample is created from the query set itself. The random sample queries method denies the intruder accurate control which covers the queries records [11]. One drawback of this method is that it could not ensure enough certainty for users to prevent the confidential data. However, the random sample queries may present precise statistics for number of records. The USA Census Bureau for example mainly works with this technique to restrict the inference in their statistics records. Every reported query is founded upon a gratuitously chosen subpopulation of the query set. The USA Census Bureau is satisfied with this method and applies it very successfully in its activity. The second approach of the output perturbation is the varying – output perturbation [1]. This method is suitable for the SUM, COUNT and PERCENTILE queries. The varying – output perturbation presents a varying perturbation to the data where random variables are used to calculate the answer to a variant of a given query. The last approach of output perturbation is the rounding where all queries are computed based on unbiased data. Afterwards the results are transformed before they are returned to the users. There are three types of rounding – systematic rounding, random rounding and controlled rounding [1]. It is advisable to combine the rounding method with methods to provide more privacy in the statistical database.
2.3.2 Query restriction method
The main idea of this method is even if the user does not want to receive deterministically right answers, these answers should be exact, for example numbers. As these answers to queries give the users forceful information, it might be important to deny the answers of some queries at certain stage to prevent the disclosure of a confidential data from the statistical database. The type or the number of queries that a user puts to the statistical database is restricted. This method discards a query which can be compromised. Nevertheless, the answers in the query restrictions are always precise. It could be concluded that the restricted group of the accepted queries considerably reduces the real usefulness of the statistical database. This method provides a protection for the statistical database by limiting the size of the query set, by controlling the overlap among the consecutive queries, by maintaining audit of any answered queries for every user and by making the small-sized cells inaccessible to users of the statistical database.
There are five subcategories of the query restriction method – query set size control, query set overlap control, auditing, partitioning and cell suppression [1].
2.3.2.1 Query set size control method
The query set size based method declines the answers to queries which have an influence on a small set of records. Fellegi [12] sets lower and upper limits for the size of the query answer which are based on the characteristics of the database. If the number of the returned records is not within these two limits, the request for the information could not be accepted and therefore the query answer may be denied. The query set size control method can be explained by the following equation [12]:
K ? |C| ? L – K,(1)
where K is a parameter set by the database administrator, |C| is the size of the query set and L is the number of the entities in the database. The parameter K must satisfy the condition [12]:
0 ? K ?(2)
The main advantage of this method is its easy implementation. However, its robustness is low so it is advisable to use it in a combination with other methods.
2.3.2.2 Query set overlap method
The query set overlap method permits only queries which have small overlap with formerly answered queries. Thus, the method controls the overlap over the queries. The lowest overlap control restrains the queries responses which have more than the predetermined number of records in common with every previous query [3]. This surveillance is valuable in the defense againstthe trackers as a compromise tool. In spite is all, this method has some drawbacks [13]. This query set overlap control is not enough effective when several users together try to compromise the statistical database. As well as the statistics for both a set and its subset are hard released which limits the efficiency of the database.
2.3.2.3 Auditing
The third subcategory of the query restriction method is the auditing. It requires the maintenance of up-to-date logs of all queries which are made by every user. It also requires a continuous check-up for potential disclosure whenever a new query is published. One main advantage of this method is that it permits the statistical database to support the users with unperturbed data and ensure that the response will not be compromised. A disadvantage of the auditing method is its excessive CPU and the requirements for the storage and processing of the collected logs [1].
2.3.2.4 Partitioning
The partitioning method groups the individual entities of a population in a number of reciprocally excessive subsets, known as atomic populations. Therefore, the records are stored in groups which consist of predetermined number of records [4]. A query is permitted only to the entire groups, but not to a subset of a group. The statistical features of these atomic populations form the raw materials which are attainable to the database users. While the atomic populations include exactly one individual entity, a high level of protection can be achieved. A research, taken by Schlorer, found that there is an emergence of the large number of atomic populations with only one entity. The result of this will be a considerable information loss when these populations are clustered. One major drawback of this method is the retrieved value of the statistical information. When the database is partitioned, the statistical data is toughly obscured. This restricts the flow of potential wanted statistical information by the users. In reality, the users may not have the chance to acquire the desired information.
2.3.2.5 Cell suppression
The cell suppression method is frequently used by the census bureau for information which is published in tabular form. This technique protects the tabular data from a compromise. The main idea is to conceal the cells that can lead to a disclosure of a confidential data. In this way, the cell suppression minimizes the suppressed cells with private information. These cells are called primary suppressions. The other cells with non confidential data, which may be a threat and lead to a disclosure, should also be suppressed. These cells with non private information are
called complementary suppressions. These complementary suppressions provide a pre-defined level of protection to the primary cells.
2.3.3 Differential privacy
As Dalenims (1977) points out that an access to a statistical database should not be allowed to a user to acquire information about an individual’s record which cannot be found out without the access of the database. This form of privacy is difficult to be achieved because of the auxiliary information. The auxiliary information is information which is available to the adversary without an access to the statistical database [14]. For example, let presume that one’s exact weight is considered as highly sensitive information and revealing this information is regarded as a privacy break. Next, it is assumed that the database provides the average weights of people of different nationalities. An adversary of the statistical database who has an access to the auxiliary information, that a particular British person is 10 kilogram thinner than the average French person, can learn the British person’s weight, as long as anyone gaining only the auxiliary information without having an access to the average weights, learns not much [15].
This leads to the application of the concept of differential privacy. In spite of the fact that the differential privacy does not exclude a bad disclosure, it ensures the individual that his or her data will not be included in the database that produces it. The differential privacy is defined as one of the successful methods of providing privacy for the statistical databases. The basic description of the differential privacy is that it is focused on providing ways to increase the accuracy of the queries from the statistical database while trying to minimize the chances of recognizing its records. The differential privacy is a randomized algorithm which accepts the database as input and generates an output [15]. A more precise definition of this method is the following formulation: A randomized function K that gives ?-differential privacy if for the databases D1 and D2, which only differ on at most one element and all S? Range (K),
Pr [K (D1) ? S] ? exp (?) x Pr [K (D2) ? S](3)
When this function K satisfies the above definition, it can ensure an individual that though this individual removes his or her data from the database, the outputs cannot become indicatively more or less acceptable.
The differential privacy strives to guarantee an adjustment to the statistical disclosure control’s problem. The differential privacy aims to publicly let out statistical information relating to a set of individuals without allowing a compromise for privacy. This method demands that there is an inherently the same probability distribution on the produced results. This probability distribution should be independent of whether each individual chooses or not the data set [16]. This process is done indirectly as at the same time it addresses all potential forms of harm and good by concentrating upon the probability of every given output of a privacy method and upon the ways for changes of the probability when any row is added or deleted from the database.
The statistical database is usually developed to reach social goals and the expanded participation in the database allows more precise analysis. Therefore, the differential privacy assures the support for the social goals by guaranteeing every individual that there is a quite little risk by connecting to the statistical database.
The differential privacy has some advantages. Firstly, this privacy preserving method is independent of any extra and auxiliary information including also other databases which are available to the adversaries. Secondly, the differential privacy is easily implemented through the using of rather sample and general techniques. The last advantage is that the differential privacy usually permits very accurate analysis.
3. Conclusion
To conclude, the statistical database provides to users statistical information for values which are based on various criteria. The field of the statistical database is highly important because it encompasses a broad variety of application areas which in principle deal with great amount of data. This statistical database may consist of confidential data which should be protected from unauthorized user access. It is very important to provide a precise statistical database with professional, legal and ethical responsibilities for privacy protection of the individual records.
Providing security in the statistical database proves to be a complicated task. There is no single solution to this problem. Therefore, numerous methods and techniques are suggested to be used to ensure privacy in the statistical database. The analysis presented in the report shows that the perturbation methods, the query restriction methods and the differential privacy are clearly among the most promising methods for the private statistical database.
References
1. N. Adam and J. Wortmann, Security – control Methods for Statistical Databases: A comprehensive Study. ACM Computing Surveys. 21 (1989).
2. I. Dinur and K Nissim, Revealing Information while Preserving Privacy – In proceeding of twenty-second. ACM SIGMOD – SIGACT-SIGART Symposium on Principle of Database Systems. (2003) p. 202-210.
3. A. Shoshani, OLAP and Statistical Databases: Similarities and Differences. (1997) p. 187
4. C. Guynes, Protecting Statistical Databases: A matter of privacy. Computer and Society. 19 (1989).
5. Z. Michalewicz, J-J Li and K-W Chen, A Genetic Approach for Statistical Database Security.13 (1990) p. 19
6. C. Dwork, F. McSherry, Calibrating Noise to Sensitivity in Private Data Analysis. Springer. 3876 (2006).
7. R. Wilson and P. Rosen, Protecting Data through Perturbation Techniques: The impact on knowledge discovery in database. Journal of Management. 14 (2003) p.13.
8. T. Wang and L. Liu, Output Privacy in Data Mining. ACM Transactions Database Systems. 36 (2011) p.11
9. S. Chawla, C. Dwork et al, Toward Privacy in Public Databases. Theory of Cryptography Conference. (2005).
10. J. Schatz, Survey of Techniques for Securing Statistical Database. University of California at Davis
11. D. Denning, Secure Statistical Databases with Random Sample Queries. ACM Transactions on Database Systems. 5 (1980) p. 292
12. I. Fellegi, On the question of statistical confidentiality. Journal of American Statistical Association. 67 (1972), 7-18.
13. D. Dobkin, A. Jones and R. Lipton, Secure Databases: Protection Against User Influence. ACM Transactions on Database Systems. 4 (1979).
14. C. Dwork, Differential Privacy. 33rd International Colloquium on Automata, Languages and Programming, part II (ICALP). Springer Verlang. (2006).
15. C. Dwork, Ask a better question, get a better answer – a new approach to private data analysis. 11th International Conference on Database Theory (ICDT ). Springer Verlang (2007).
16. C. Dwork, Differential privacy in New Settings. Society for Industrial and Applied Mathematics. (2010).
Cite this Page
Private statistical database. (2019, Mar 29). Retrieved from https://phdessay.com/private-statistical-database/
Run a free check or have your essay done for you
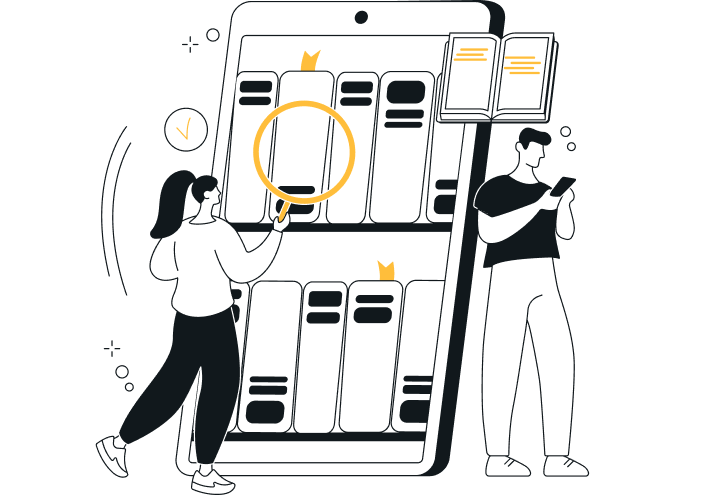