Covariation: The Dance of Two Variables
Understanding the link between two or more variables is crucial in the large field of statistical analysis. Covariation, which is sometimes confused with correlation, sheds light on how different variables evolve in tandem. It serves as the foundation for many cutting-edge analytical methods and offers detailed knowledge of the joint variability of paired data sets. The essay elucidates the concept of covariation, delving into its definition, applications, distinctions from related concepts, and inherent limitations. However, proper care must be taken in its interpretation, especially when distinguishing it from correlation or deducing causation. As with all tools, its power lies not just in its application but in the knowledge and discretion of its application.
Covariation Definition
The term "covariation" describes how well one variable may predict the change in another. It shows the direction of a link rather than its intensity (like correlation). Positive covariation is the growth or decrease of two variables concurrently. In contrast, they show negative covariation when one variable rises while the other falls.
Order custom essay Covariation: The Dance of Two Variables with free plagiarism report
Investment and portfolio management: In the realm of finance, recognizing how returns on assets covariate (or covariance) may help to diversify a portfolio. Assets with negative covariance may aid in risk mitigation.
The covariation principle is crucial to causal reasoning in psychology and medicine. Professionals look for covariation as proof when analyzing the link between a certain stimulus and reaction or medicine and its effects.
Covariation helps econometricians comprehend how many economic variables relate to one another. For instance, knowing how consumer spending and economic growth co-vary might provide important information about the state of the economy.
Correlation vs. covariance
Although they are both used to describe connections between variables, these phrases are not interchangeable. A linear relationship's strength and direction are shown by the correlation, a standardized metric that has a range from -1 to 1. The interpretation of covariation, on the other hand, is more difficult since it is non-standardized and its value might vary from negative infinity to positive infinity.
The constraints of covariation are similar to those of other statistical metrics. It may not effectively capture more complicated connections since it simply records linear correlations. Furthermore, an important difference in scientific investigations is that a significant covariation does not necessarily suggest causality.
Conclusion:
Although complicated, covariation offers a fundamental knowledge of how variables interact. Its uses in a variety of domains, including psychology and finance, demonstrate its adaptability. However, adequate caution must be used when interpreting it, particularly when separating it from correlation or determining causality. Like other tools, its effectiveness depends on both how it is used and how it is done so with expertise and tact. It is the bedrock on which many advanced analytical techniques are built, providing nuanced information about the joint variability of paired data sets.
References:
- P. L. Meyer (1970). Statistical Applications and Basic Probability. Addison-Wesley.
- K. A. Bollen (1989). Equations for Structures with Latent Variables. Wiley & Sons, Inc.
- D. C. Howell (2007). Psychology Statistical Methods. Watson, Thomson.
- J. Pearl (2009). Causality: Models, Inference, and Reasoning. Press of Cambridge University.
Cite this Page
Covariation: The Dance of Two Variables. (2023, Aug 22). Retrieved from https://phdessay.com/covariation-the-dance-of-two-variables/
Run a free check or have your essay done for you
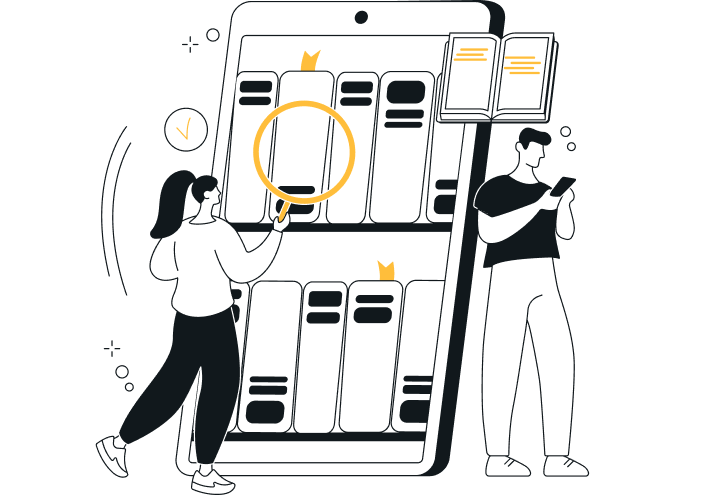