Specific Crop Mapping Temporal Data Approach Environmental Sciences Essay
To know apart specific harvest of involvement, in this work we have used temporal informations. These temporal informations sets were pre-processed with regard to geo-registration, set ratio and eventually fuzzy based categorization attack was applied. For accuracy appraisal fuzzy based mistake matrix was applied and soft mention informations was generated utilizing IRS-P6, LISS-III temporal informations sets. It has been tried to accomplish defined aim of this research undertaking and elaborate methodological analysis has been explained further.
Study Area
The present survey is undertaken to research the feasibleness of using AWIFS ( IRS-P6 ) and LISS-III ( IRS-P6 ) information for function and proving individual category ( Wheat ) of involvement. The trial location was selected in parts supplying a scope of disputing environments for specific harvest monitoring. india
Order custom essay Specific Crop Mapping Temporal Data Approach Environmental Sciences Essay with free plagiarism report
The country is situated in the southern portion of the Uttarakhand province between 29° 11 ' 21.99 '' N to 29 ° 42 ' 06.73 '' N and 78 ° 38'18.30 '' Tocopherol to 79 ° 10 ' 191.05 '' E. The country is located in Terai part and is a portion of Kumaon Division. The survey country is celebrated for its agribusiness and irrigation on synchronized forms from the past as granary of popularity for its productiveness in paddy harvests in the whole Uttarakhand province. Khariff and Rabi are two major cropping seasons. The chief Khariff harvests are rice, soyabean, Urd, and Moong and the Rabi harvests are wheat, barley, Gram, Masoor, Mustard, and Sunflower.
uttranchal pantnagar
Pre-processing of the Data
( Geometric Correction of ( IRS-P6 ) LISS-III and AWIFS Data )
The mention LISS_III images available were already pre-processed as follows: SOI toposheets were scanned and converted into digital signifier before geo-registration. Images were co-registered with regard to the toposheet maps on 1:50,000 in UTM projection with WGS-84 North ellipsoid of revolution and data point, zone 45 ( Kashipur Area ) Uttarakhand. The images were re-sampled at 20m spacial declaration by utilizing the nearest neighbour re-sampling method ( first order multinomial ) and entire 17 GCPs were collected from the toposheet for the intent of geo-registration.
The trial images available for categorization were likewise pre-processed. These AWIFS images of same country were co-registered with regard to the LISS-III dataset in UTM projection similar to LISS-III images. Similarly, the images were re-sampled at 60m spacial declaration by utilizing the nearest neighbour re-sampling method and entire 17 GCPs were collected from the LISS-III images for the intent of geo-registration. The difference between trial image ( AWIFS ) and mention image ( LISS-III ) is 1:3, which implies that, one pel of AWIFS image is equal to nine pels of LISS-III image.
Methodology Adopted
In the present survey the medium spacial declaration AWIFS informations sets are used. In order to accurately map the specific crop-wheat, we use temporal informations sets. Different datasets with changing temporal declaration were taken in order to happen out the most suited time-series ( multi-date ) image set that gives the best truth of categorization.
In 2008-2009 Rabi Season- Wheat Growth Period
Nov Dec Jan Feb Mar Apr
Suitable set ratio method
Suitable fuzzy based supervised categorization for pull outing individual category of involvement
Accuracy appraisal ( Using soft mention informations )
Figure: Methodology adopted
The enhanced images were available for our survey. The images are, in general, enhanced by pre-processing like geometric and atmospheric corrections. The images are geo-referenced utilizing land control points and so registered. This work is done utilizing commercially available ERDAS package tool.
Training sites for wheat were identified in the digital LISS III and AWIFS image with the aid of land control point inside informations provided and the visually interpreted FCC images.
Band ratio techniques were employed to extinguish the consequence of incline and facet and the difference of light. Normalized Difference Vegetation Index ( NDVI ) , MIN/MAX and Transformed Vegetation Index ( TVI ) were taken for all the images from AWIFS and LISS-III. This was done utilizing the SMIC package tool.
The fuzzy set theory based sub-pixel categorization technique was used for farther categorization utilizing temporal informations. The samples of wheat were taken from both AWIFS and LISS-III time-series images severally. SMIC package tool at IIRS was used. Sample was taken in the mode that, one image was in the spectator while, other temporal images were arranged as the chink on spectator pick the value of all the images at that peculiar pel ( category ) . The fuzzy based classifier was used for categorization. The categorization was done by Possibilistic c-Means classifier attack. The end product was a individual classified fractional image, from which we analyzed the individual category ( wheat harvest ) .
Further, the truth appraisal of all the classified AWIFS fractional images was taken with regard to LISS-III image. FERM ( Fuzzy Error Matrix ) was used for truth appraisal.
Concepts Employed
Band Ratio Techniques
The procedure of spliting the pel values in one spectral set by the corresponding pel values in another spectral set is known as set ratioing. It is merely a simple transmutation process applied to remote feeling images. The form of the spectral coefficient of reflection curves of different land screen / land usage types can be identified by this technique. Second it can cut down the recorded unwanted topographic consequence like incline and facet and extinguish the effects of difference in light. The ratioing technique such as spectral flora index are widely utile and benefits the legion subjects like appraisal of biomass, H2O usage, works wellness, harvest production and works emphasis. Vegetation Indices combine different spectral sets, quantitatively step and measure the flora screen denseness, sort the harvest and besides help in harvest favoritism.
The ratioing techniques used in this survey are as follows
Min/Max
It is one of the easy to calculate set ratio technique. It is obtained by taking the ratio between the minimal set value and maximal set value matching to that pel.
Ratio Image = Minimum Value/Maximum Value
NDVI
One of the first successful flora indices based on set ratioing was developed by Rouse et Al. ( 1973 ) . They computed the normalized differences of brightness values from MSS7 and MSS5 for supervising flora. They called it the Normalized Differences Vegetation Index ( NDVI ) Jenson 1996. The NDVI is measured in graduated table of -1 to +1. Snow, H2O organic structures, desert and exposed dirts come in a scope of -0.2 to 0.05. While the increasingly increasing sums of green flora come in the scope of 0.05 to 0.7. NDVI informations are strongly correlated with the fraction of exposure synthetically active radiation ( 0.4 to 0.7 Aµ.m. beckon length ) absorbed by flora canopies. The brighter the image pel after categorization, the greater the sum of photosynthesizing flora nowadays.
NDVI = ( NIR-R ) / ( NIR+R )
TVI
TVI was introduced by Deering et Al. ( 1975 ) . They added 0.5 to NDVI and took the square root, bring forthing the transformed flora index ( TVI ) . The TVI can be linearly correlated with leaf country index, and has a higher sensitiveness than that of NDVI in high biomass country ( Huete et al. , 2002, Sakamoto et al. , 2005 ) . Since the biomass of harvest Fieldss is low compared with woods, TVI retains one-dimensionality in harvest Fieldss. Furthermore, TVI is more practical than NDVI when humidness is high.
TVI = a?s ( NDVI + 0.5 )
Image Classification Techniques
For the readying of thematic maps and quantitative analysis of the images, capableness of computing machine to construe the images, place pels and label them based on their numerical belongingss is exploited. The method is normally referred to as Image Classification. While the images can processed in digital environment it is better known as digital image categorization.
A traditional difficult categorization technique does non assist in this type of state of affairs. Fuzzy logic may be good where multiple categories exist within a pel. To integrate the assorted pel job in past research workers have proposed the 'soft ' categorization technique that decomposes the pel into category proportions ; fuzzed categorization is a soft categorization technique, which deals with vagueness, ambiguity and uncertainness in category definition. Therefore fuzzed categorization technique is likely the best technique to pull out the individual category from the image and differentiate with other categories.
The construct of 'fuzzy set ' theory was introduced by Zadeh, to cover with the uncertainness in category definition. The fuzzed set theory introduces the vagueness by extinguishing the chip boundaries into grade of rank to non-membership map Binaghi et Al. 1999. It represents the state of affairss where an single pel is non a member for a individual bunch, but member for all bunchs with different grade of belongingness Dutta 2009.
In this survey fuzzy logic based algorithm, which is independent of statistical distribution premise of informations, has been studied to pull out individual land screen category from distant feeling multi-spectral images. Fuzzy based classifier in this work has been implemented in such a mode that remote feeling image from any detector can be used for individual category extraction.
Temporal Data Approach
At the 3rd degree of categorization where we map the specific flora, mapping based on categorization utilizing individual day of the month image has been merely reasonably successful.
The first job in this survey is to pull out individual harvest of involvement from the harsh declaration orbiter image. There is job when pull outing individual category with individual day of the month image. The image consists of pels. A pixel value ( brightness value ) recorded is consequence of interaction of electromagnetic moving ridges with the land objects and/or atmosphere. In add-on harvests may hold similar spectral response forms holding merely little differences. Hence, the spectral response recorded by detector may differ for same or similar type of categories while it may be possible that the dissimilar entities may demo similar spectral response, depending on land or atmospheric conditions. This introduces mistakes. So it is impossible to accurately pull out the individual harvest utilizing the individual day of the month imagination.
To get the better of the above issues, temporal informations attack best for specific harvest function. With the clip series informations the spectral response of the category proportions can be recognized and differentiate from other categories. Therefore individual harvest of involvement has been processed utilizing temporal informations.
Accuracy Assessment
Accuracy appraisal and proof for sub-pixel classifiers is still a topic of research. No standard methods are available for sub-pixel classifiers, unlike that for hard-classifiers such as mistake matrix and kappa coefficient. For the proof of the consequence, FERM ( Fuzzy Error Matrix, Binaghi et al. ) with the aid of fuzzed set theory based sub-pixel classifier was used in this survey. The truth appraisal of AWIFS fraction images has been done with regard to LISS-III fraction image. The fuzzed mistake matrix ( FERM ) method has been employed to calculate the truth.
Classified Image
Assorted Pixel
Reference Image
Figure: Sub-Pixel Accuracy Assessment Method
( Comparing coarse declaration image with all right declaration image )
Fuzzy Error Matrix ( FERM ) :
For the appraisal of soft classified informations assorted suggestion have been made, among which fuzzy mistake matrix introduced by Binaghi et al. , 1999 is one of the most suited attack. FERM takes the fraction soft classified images ( drifting value or non-negative existent figure ) as input alternatively of traditional difficult classified images ( integer value ) . The layout of a fuzzed mistake matrix is similar to that of the traditional mistake matrix that is used for accessing the truth of difficult classifiers. The component of the fuzzed mistake matrix represent category proportions, matching to soft mention informations ( Rn ) and soft classified information ( Cm ) , in categories n and m severally. Fuzzy lower limit operator are used to build the fuzzed mistake matrix and find the matrix elements M ( m, N ) in which the grade of rank in the fuzzed interaction ( Cm a?©aˆ? Rn ) is computed as ( Kumar et al. , 2007 )
M ( m, n ) = |Cm a?©aˆ?Rn| a?‘xIµX min ( AµCm, AµRn )
Where Ten is the proving sample dataset ; x is a proving sample in X and AµCn and AµRn are the category rank of proving sample tens in Rn and Cm, severally.
In our survey, fuzzy based mistake matrix was applied for truth appraisal and soft mention informations was generated utilizing IRS-P6, LISS-III temporal informations sets. Producer, user and overall truths of categorization were computed by this fuzzed mistake matrix ( FERM ) truth assessment method.
Consequences and Discussion
Sample Output Images
Following are the corresponding subdivisions of end product images from temporal informations set-6.
Acquisition Date Input Images: Min/Max Band Ratio Image: Concluding Wheat Function
21 Nov 2008 C: UsersAmolDesktopImages_ Reporta.jpg C: UsersAmolDesktopImages_ Reportm6_a.jpg
08 Jan 2009 C: UsersAmolDesktopImages_ Report.jpg C: UsersAmolDesktopImages_ Reportm6_b.jpg
27 Jan 2009 C: UsersAmolDesktopImages_ Reportc.jpg C: UsersAmolDesktopImages_ Reportm6_c.jpg
15 Feb 2009 C: UsersAmolDesktopImages_ Reportd.jpg C: UsersAmolDesktopImages_ Reportm6_g.jpg C: UsersAmolDesktopImages_ Reportm6_final.jpg
25 Feb 2009 C: UsersAmolDesktopImages_ Reporte.jpg C: UsersAmolDesktopImages_ Reportm6_e.jpg
11 March 2009 C: UsersAmolDesktopImages_ Reportf.jpg C: UsersAmolDesktopImages_ Reportm6_f.jpg
31 March 2009 C: UsersAmolDesktopImages_ Reportg.jpg C: UsersAmolDesktopImages_ Reportm6_g.jpg
Categorization Accuracy
To understand and exemplify the efficiency of the fuzzy set theory based sub-pixel classifier along with assorted sets ratio techniques and temporal informations sets, used in appraisal of specific harvest of involvement, truth appraisal and reappraisal is required.
The truths of the fraction images ( proportion of wheat harvest ) generated by sub-pixel classifier of Kashipur country are shown in table 4.
Table 4: Accuracy Assessment of wheat harvest function in Kashipur country
Vegetation Index
Accuracy
User
86.50
88.06
86.01
87.15
89.26
89.58
87.83
90.64
Manufacturer
96.30
92.92
95.36
95.00
95.34
96.07
95.62
95.63
Over All
96.30
92.92
95.36
95.00
95.34
96.07
95.62
95.63
NDVI
User
92.40
89.68
92.14
92.20
93.24
93.37
91.87
94.13
Manufacturer
94.24
93.56
94.67
95.68
94.99
95.97
95.10
95.69
Over All
94.24
93.56
94.67
95.68
94.99
95.97
95.10
95.69
TVI
User
73.83
80.13
76.00
76.46
78.73
79.86
79.06
79.37
Manufacturer
95.23
93.24
94.53
93.66
92.79
96.15
94.66
96.09
Over All
95.23
93.24
94.53
93.66
92.79
96.15
94.66
96.09
Graphic Representation
Consequences
The Min/Max set ratio technique gives the best truth of wheat harvest categorization in four sets of temporal informations and is followed by TVI flora index which gives maximal truth of wheat harvest function in three sets of temporal informations.
The truth of categorization of wheat harvest, obtained by using the Min/Max set ratio technique is highest in set-1 and about near to this maximal value in set-6. The truth of wheat harvest mapping utilizing NDVI flora index is maximal in set-6 and same is true for TVI flora index.
Discussion
In this survey fuzzy set theory based sub-pixel classifier has been undertaken for pull outing the individual harvest type ( wheat ) utilizing multi-spectral orbiter images. Three indices Min/Max, NDVI and TVI were used to supervise, estimation and discriminate wheat other harvest types. Fuzzy set theory based sub-pixel classifier which gives the fraction images was applied to band ratio images. Fuzzy Error Matrix ( FERM ) method was applied for truth appraisal of wheat harvest function and proof of the consequences.
Irrespective of the figure of images in each multi-date temporal informations set and irrespective the flora index used, this fuzzy set theory based sub-pixel classifier gives reasonably good overall truth, which is ever supra 92.5 % . It thereby establishes certificates of fuzzed set theory based classifier for mapping specific harvest.
Further comparative survey of the effects on truth, of flora index used to supervise and know apart wheat harvest shows that, Min/Max flora index is best suited to supervise and know apart wheat harvest while utilizing temporal informations sets. Min/Max flora index gives best truth in half of the temporal informations sets tested. However it does non demo specific form in giving truth as the temporal informations sets vary. TVI flora index besides gives maximal truth in three sets, but the fluctuations, in the truths is maximal. Though NDVI ratio does non acquire the highest topographic point in the truth of categorization, there is some form seen in the truth of categorization under NDVI flora index. It in general additions from set-2 to set-6 which can be explained mistily as better truth for better temporal declaration.
Reappraisal of the truth of categorization, with regard to temporal declaration and the day of the months of the images used, suggest that in general overall truth additions from set2 to set6 as the figure of images used addition. Classification truth is highest for set6. As can be seen from Table3, set6 contains images from seeding to harvest home of the harvest and about at temporal declaration of 20 yearss. There is a dip in the categorization truth in set2. That is likely due to inclusion of image of day of the month 6 Nov. 2008 ( before sowing of the harvest ) . It besides explains the dip in categorization truth for set7.
Decisions and Recommendations
Motive was to map individual harvest of involvement utilizing fuzzy based classifier with the aid of time-series multi-spectral satellite images. The temporal information helps in favoritism of harvest ( particularly wheat as tested here ) from other harvests.
It has been observed from this work that time-series multi-spectral images used for specific harvest function give good overall truth of categorization. Harmonizing to consequences obtained from this work, Min/Max flora index gives maximal truth of function for wheat harvest. NDVI flora index is besides reasonably good and TVI can besides last the intent.
Temporal informations set incorporating multi-date images that equally cover the full harvest life rhythm ( seeding to reaping ) gives the maximal accurate function of the harvest. In instance of wheat, the temporal declaration of 20 yearss gives better truth. However, inclusion of images of day of the months other so cropping season ( those before seeding the harvest and after reaping the harvest ) will cut down the overall truth of categorization.
Cite this Page
Specific Crop Mapping Temporal Data Approach Environmental Sciences Essay. (2018, Sep 01). Retrieved from https://phdessay.com/specific-crop-mapping-temporal-data-approach-environmental-sciences-essay/
Run a free check or have your essay done for you
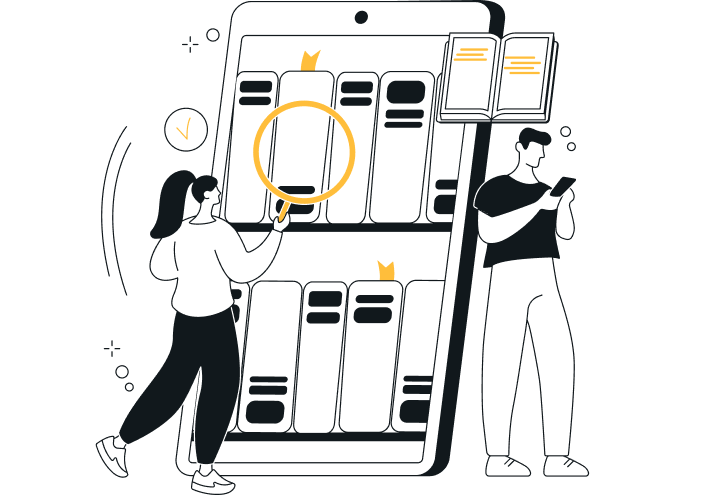