Bankruptcy Prediction Models
Introduction
The continuous increase in business bankruptcy over the past several decades has been alarming. Despite numerous studies and predictions from researchers and analysts, there seems to be inconclusive evidence to prevent business bankruptcy. The subject of bankruptcy has had significant publicity recently with the Enron and WorldCom business practice irregularities. These misdeeds have raised many questions about management obligation, honesty, and auditor responsibilities. This situation has sharpened everyone’s awareness of the significant impact of bankruptcy on the economy and its destruction to investors, lenders, and the public.
Order custom essay Bankruptcy Prediction Models with free plagiarism report
There are many reasons for bankruptcy. These reasons can be classified as external and internal. Among the external reasons are competition, economic recession and depression, and federal regulation and deregulation (Altman & Hotchkiss, 2005). Some of the internal reasons are executive incompetence, corruption, unwillingness to adjust to environmental changes, and corporate fraud (Altman & Hotchkiss, 2005).
Many bankruptcy studies find that the probable reasons for an organization failure differ from one business to another (Altman & Hotchkiss, 2005). Additionally, the effect of a failing firm may take many years before its impact shows on the organization. Hence, bankruptcy should be uncovered through the examination of financial information (Altman & Hotchkiss, 2005).
There are many approaches to bankruptcy prediction. Financial ratios seem to be the dominate method of analysis. This method has the ability to predict business failure accurately and can be used for early warning to prevent bankruptcy. This paper reviews the relevant theoretical literature pertaining to business failure using financial ratios to predict bankruptcy models. A numbers of models are classified according to the statistical methodologies used. Finally, some conclusive remarks are presented.
Bankruptcy Theory and Prediction Model
The history of bankruptcy theory dates back to 1542 in England. During this period, a bankrupt individual was believed to be a criminal and was subject to imprisonment or the death penalty (New Generation Research, Inc., 2000). Early bankruptcy laws in the United States were enacted because of land speculation, panic in the economy, and economic upheaval in 1800, 1837, and 1867 (New Generation Research, Inc., 2000). These laws were repealed in 1803, 1843, and 1878 respectively.
Modern U.S. bankruptcy laws provide protection for organizations in distress. The first law in this category was enacted in 1898 to rehabilitate and reorganize organizations in debt. In 1933 and 1934, new bankruptcy acts were enacted as a result of the Great Depression. The Chandler Act of 1938 reexamined these laws by providing significant conditions for reorganization of businesses.
The 1978 Bankruptcy Reform Act significantly overhauled bankruptcy procedures. This law gave the courts more power, and in 1982, the Supreme Court ruled against bankruptcy judges for enormous power and overlap duties. In 1984, the Bankruptcy Amendment Act was enacted with part of its provisions limiting the rights of business organizations to terminate labor contracts (Levins, 1997).
During the 1980s and 1990s, a significant number of organizations filed for bankruptcy. These numerous bankruptcies produced several tests for the court system. However, the courts seemed to control the situation very well. The 1994 Bankruptcy Reform Act provided comprehensive protection for both businesses and individuals. In 2005, the Bankruptcy Abuse Prevention and Consumer Protection Act were enacted. This was the first major reform of the bankruptcy system in more than 25 years. The consumer bankruptcy provisions of the act were in response to several factors including an increase in bankruptcy filings and losses associated with bankruptcy filings (Altman & Hotchkiss, 2005).
The number of bankrupt firms is important for the economy and can be an indicator of economic health. For example, in 1998, bankruptcy filings surpassed 1 million for the first time in U.S. history. The number of bankruptcy filings has almost doubled over the past decade to more than 1.6 million in 2004 (Altman & Hotchkiss, 2005). These bankruptcies resulted in many jobs losses and attributed to economic depression. The use of financial ratios to predict bankruptcy is nothing new among academicians and analysts.
The attention given ratio analysis, however, has been significant within the past decades for several reasons. Bankruptcy is considered a major event relating to financial information that concerns stakeholders. Firstly, during the period before 1930, creditors were the major providers of goods and services that focused on the liquidity ratio for business decisions. Secondly, financial ratio is straightforward and relevant to creditors’ analysts. Lastly, bankruptcy prediction has the ability to evaluate financial information.
Early Analysis Prediction Model
The work of Smith and Winakor (1930) is one of the early analysis prediction models. Their study tested the efficiency ratio to predict business failure. During the 1930s, there was no empirical testing of financial ratios to confirm the claims of several bankruptcy studies. Smith and Winakor selected firms with financial problems and used 21 ratios to analyze their performance for a period of 10 years before failure. The study indicated that firms with financial problems had worsening ratios for many years before they actually failed. However, it should be noted that the overall economy was declining because of the 1929 U.S. stock market crash (Levins, 1997).
The next early analysis prediction model is the work of Fitzpatrick (1934). He compared the relationship of profitable industrial enterprises with those of failed enterprises. His objective was to establish if financial ratios could provide important assessment in differentiating between failed and nonfailed firms. His study represented one of the early studies that evaluated business failure with the use of financial ratios. Fitzpatrick collected information about 20 failed firms and 20 nonfailed firms during the period 1920–1929 and matched failed and nonfailed firms one by one. Fitzpatrick used 13 popular ratios to test the validity of the assumptions, and concluded that financial ratios displayed differences in the performance of failed and nonfailed firms (Levins, 1997).
Another early analysis prediction model is the work of Merwin (1942). He used financial ratios to analyze data collected from the income tax returns of 200 failed firms and 381 nonfailed firms during the period 1926–1935 from manufacturing firms in five industries (clothing, stone clay, furniture, machine tool, and baking). During this time, a firm failed if income tax was not filed or if the firm was continuously in deficit and was not part of a business merger or acquisition. The analysis indicated that current ratio, net working capital to total assets, and net worth to total debt were most useful (Levins, 1997).
It is beneficial to all stakeholders when traditional ratios such as cash over total debt, net income over total assets, current liabilities and long-term liabilities over total assets, working capital over total assets, and current assets over current liabilities are used to evaluate the short-term credit worthiness of a firm. Ratio analysis exposes poor performance, and it is a good indicator of short-term financial problems.
Univariate Analysis Prediction Model
Univariate analysis is a statistical method that requires the use of one financial ratio only to predict business failure model. Beaver (1966) uses this method successfully. He initiates the use of univariate analysis of financial ratios to predict business failure. It is a theory of cash flow that serves as a framework for testing financial ratio. Some people believe his work is a revelation into the future of financial ratio analysis. The objective of the study was to test the usefulness of financial ratio in the business environment to predict bankrupt.
Business failure is the lack of ability of an organization to meet its immediate responsibility. According to Beaver, events such as bankruptcy, bond default, bank overdraw, and nonpayment of preferred stock dividend are examples of business failure. The poor circumstances that give rise to deteriorating financial ratios are often reflected in clear situations, such as slowing down in the payment of vendors’ bills, slow deliveries to customers, and supplier and customer complaints.
Beaver (1966) selected a sample of 79 failed firms and 79 nonfailed firms based on size and industry. He applied a univariate test to each of the 30 different ratios. The ratio was selected based on literature popularity, previous performance, and a defined cash-flow concept. The prediction result was then compared to the actual business status as failed or nonfailed to determine the accuracy of the prediction.
Beaver selected six financial ratios as the most accurate prediction for business failure: cash over total debt, net income over total assets, current liabilities and long-term liabilities over total assets, working capital over total assets, current ratio (current assets to current liabilities), and no-credit interval (defensive assets less current liabilities over fund expenditures for operations) (Beaver, 1966).
The model uses a number of procedures including a mixed industry sample (firms from different industries except financial services), a split sample to test for validity and bias, and separation of the results into type I and type II errors. A type I error is when a failed company is classified as nonfailed, and a type II error is when a nonfailed company is classified as failed.
The model achieves a moderate prediction of business failure with the use of financial ratio. The overall classification yields the rate of 87%, 79%, 77%, 76%, and 78% for year 1, year 2, year 3, year 4, and year 5 respectively before failure. This rate will always be better than the random-prediction model, which has a probability rate of 50%. The best ratio is the cash flow over total debt. Total debt over total assets and net income over total assets also predict business failure far better than 50% for each year.
The rest of the ratios perform satisfactorily in year 1 and year 2 but unsatisfactorily in year 3, year 4, and year 5. Beaver’s (1966) model reveals a major relationship between the accounting numbers and financial strength of a firm. The use of financial ratio should be based on judgment because financial ratio does not predict with the same accuracy and does not predict equally between failed and nonfailed firms. Moreover, the ratio might not reflect the actual situations that exist among the variables.
The significant measure is to test the anticipated loss with the Bayes’ decision rule (a fundamental statistical approach to the problem of pattern classification that minimizes the probability of error) based on the financial ratio of interest, and match the anticipated loss with the one based on a naïve model in order to get a sign of the value of the financial ratio. The ratio might be very useful in some situations, whereas it might be useless in other situations.
The difficulty in evaluating the anticipated loss arises because the curves need to fit the ratio’s distributions for failed and nonfailed firms. Moreover, the data in Beaver’s (1966) study were based on roughly small samples that did notfurnish sufficient information about the functional forms of these distributions, particularly about their tails. The major weakness is the categorization of the sample as failed or nonfailed firms without realizing that a failing firm may bounce back before collapse.
Another significant study in this area is the work of Wilcox (1971). His theory is based on the gambler’s ruin model of probability theory to predict business failure. This theory states that a gambler begins a financial game with money that will grow by winning money (1+P) or diminish by losing money (1-P), where 1 is the initial money and P is the probability of winning or losing. The game continues for a sequence of independent tryout until the gambler is bankrupt. The method calculates the probability of final gain or loss and the length of the game.
In the application of the gambler theory, a firm is financially bankrupt when its net worth reduces to 0. The theory applies alternative cash flow ratios using five or six observations to financial data and then sums up for several years. Adjusted cash flow is averaged to obtain a mean value and a variance of the probability of bankruptcy. Interpretation of the model is that any value greater than 0 indicates a healthy firm, and any value less than 0 indicates a distressed, failing firm.
Multivariate Analysis Prediction Model
Multivariate (many variables) in statistical terms implies that at least two dependent variables (outcome variables) are concurrently reviewed in a single analysis. The principal concept of the multivariate analysis is to mix data of different financial ratios into one weighted index (Laitinen, 1991). A multiple discriminant analysis (MDA) is similar to a regression analysis resulting in either accepting or rejecting the outcome. MDA is a multivariate analytical technique of statistical analysis. Some of the significant research in the area of business failure has adopted the use of multivariate analysis.
However, only a handful of researchers that have developed models to predict business failure using this technique will be discussed. The first significant research in the area of business failure using multivariate analysis is the work of Altman (1968). He utilized MDA to predict business failure using financial ratios. The benefit of this method is that it regards all characteristics at once while perceiving their connection. Altman developed a theory known as the Z-score bankruptcy prediction model that uses financial data information. The theory evaluates the responsiveness of the ratio analysis to statistical technique and the ability of the multiple discriminant analysis technique to improve the business failure models.
In the model, independent variables use information from periods prior to failure, whereas dependent variables are classified into two groups as bankrupt firms and nonbankrupt firms. Altman used firms that had filed for bankruptcy protection during the period 1946 to 1965. He used a matched sample of 33 failed firms and 33 nonfailed firms selected by a convenience sample based on industry and size. Drawing statistically valid conclusions from a convenience sample is impossible.
However, the matching technique gives advantages, particularly in regulating variables not correctly included in the model (Zavgren, 1983). MDA was applied to 22 financial ratios selected based on their popularity, newness, and relevancy. The ultimate discriminant function contains five ratios named the Z-score model. The formula for the Z-score is Z = 1.2x(1) + 1.4x(2) + 3.3x(3) + 0.6x(4) + 1.0x(5). The explanation of the formula for Z, x(1), x(2), x(3), x(4), and x(5) is overall classification index, working capital over total assets, retained earnings over total assets, earnings before interest and taxes over total assets, market value of equity over book value of total debt, and sales over total assets respectively.
Cash flow to total debt, which Beaver (1966) described as an excellent predictor, is not part of the variables set because of unsmooth behavior and accurate depreciation information. A high probability of failure is assigned to a Z-score of less than 1.81. Any firm in this category is a business failure and is considered bankrupt. A financially healthy firm is assigned a Z-score greater than 2.99. This category of firms has no problem in continuing as a business. The zone of ignorance according to Altman is the region of a Z-score between 1.81 and 2.99 inclusively. This category of firms has the tendency to be misclassified. However, Altman adopted a cutoff point of 2.675. Any firm with a Z-score below the cutoff point is considered a business failure.
This model is accurate for 2 years before failure with a prediction rate of 95% and 82% respectively. The use of Z-score can improve prediction of financial failure and provide a better opportunity to serve the stakeholders. The accomplishment of the theory is based on the reality that financial information of failed firms is different from the financial information of nonfailed firms.
Another significant study in the area of business failure using multivariate analysis is the work of Deakin (1972). He suggested a different business failure theory to the ones pioneered by Beaver (1966) and Altman (1968). In his work, Deakin duplicated Beaver’s (1966) model for the 14 ratios considered to be an excellent predictive ability. He uplifted the correctness of Altman’s theory by utilizing all of Beaver’s ratios as established. Furthermore, Deakin used a probabilistic grouping rule rather than a critical value.
Deakin defined failure as insolvency, bankruptcy, or liquidation of firms (Deakin, 1972). He selected 32 firms on a random basis for both failed firms and nonfailed firms during the period 1964 to 1970 based on industry and size. He used univariate analysis to duplicate Beaver’s results and used the cash flow over total debt ratio to classify results with a similar outcome. Deakin concluded that the cash flow over total debt ratio was the best predictor for both failed and nonfailed firms during the 5 years before failure.
Moreover, Deakin (1972) also performed a multivariate discriminant analysis using all the ratios adopted from Beaver. He achieved higher classification accuracy with prediction rates of 97%, 95%, and 95% for year 1, year 2, and year 3 respectively before failure. However, the prediction rate decreases in year 4 and year 5 to 80% and 83% respectively. Deakin concluded that discriminant analysis can be applied to predict business failure for 3 years in advance with extreme accuracy. The result of this study showed some improvement over Altman (1968), which predicted for only 2 years before failure.
Furthermore, Deakin illustrated the bias of prediction models to misclassify nonfailing firms when the sample selected is increasingly made up of the population of all firms. He explained that the outcome is a mean of speculating failure. In other words, there is a propensity to assume that many firms fail when those firms do not fail. Deakin commented on prior research that ignored the opportunity for a failing firm turnaround. His conclusion is to use financial condition to evaluate the population of both failing and nonfailing firms. However, this is just one of the recognized uses of business failure prediction models.
The next significant research in the area of business failure using multivariate analysis is the work of Altman (1993). He revised the original Z-score model, calling it the revision four-variable Z-score bankruptcy prediction model. He included nonmanufacturing firms and eliminated the sales over total assets variable to reduce the possibility of industry effect. Moreover, Altman replaced market value of equity with book value of equity. These changes affect the coefficients of the variables, the group means, and the cutoff points.
In the revised model, the ultimate discriminant function contains four ratios. The newly revised Z-score is Z = 6.567x(1) + 3.26x(2) + 6.72x(3) + 1.05x(4). The explanation of the formula for Z, x(1), x(2), x(3), and x(4) is overall classification index, working capital over total assets, retained earnings over total assets, earnings before interest and taxes over total assets, and book value of equity over book value of total debt respectively.
In the revised model, a high probability of failure is assigned to a Z-score of less than 1.10. Any firm in this category is a business failure and is considered bankrupt. A financially healthy firm is assigned a Z-score greater than 2.60. This category of firms has no problem continuing as a business. The zone of ignorance or gray area according to Altman is the region of a Z-score between 1.10 and 2.60 inclusively. This category of firms has the tendency to be misclassified. The results of Altman’s revised model is rarely less dependable than Altman’s original model. The revised model seems useful especially in the leasing industry, where assets financing differ among firms.
The last significant research in the area of business failure using multivariate analysis is the work of Poston and Harmon (1994), who considered the effectiveness of financial ratio to differentiate between financially distressed firms that succeed in turning around and those that do not. Their sample consisted of distressed manufacturing firms during the period of 1970 to 1976. Previous studies failed to examine this situation. A distressed firm has at least 2 years of continuous operating losses, or less than 1.0 in current ratio during any year or negative retained earning balance (Poston & Harmon, 1994).
The study tested the ability of Altman’s (1968) Z-score model and the ratio-based failure prediction model. A firm that accomplishes turnaround can change prediction results, and yet these financially distressed and turnaround firms are not likely to be identified. Poston and Harmon (1994) commented that prior bankruptcy prediction studies incorrectly classified and failed to consider turnaround firms nearing bankruptcy. They distinguished between turnaround firms in distress and distressed failing firms. Their study concluded that financial performance can affect prediction outcome significantly.
Other Models
Regression Analysis Prediction Model
Many situations demand decisions or inferences about how data from a certain variable can be used to determine the value of some other related variable. Regression analysis is the relationship or correlation between two factors, events, or characteristics, and the data are at least of the interval measure. Regression and correlation analysis assume recorded data; a scatter diagram created to see any obvious relationships or trends; a correlation coefficient factor used to obtain objective analysis that will uncover the magnitude and significance of the relationship between the variables; and a test to determine if the correlation coefficient is statistically significant.
The purpose of correlation is to find relationship between two or more variables with the hope of better understanding the conditions and events. The linear correlation coefficient measures the strength of the linear relationship between two paired variables in a sample. If there is a linear correlation (the correlation coefficient is large enough between two variables), then regression analysis is used to identify the relationship with the hope of predicting one variable from the other. However, if there is no significant linear correlation, then a regression analysis cannot be used to make predictions (Meyer and Pifer, 1970).
Logit and Probit Analysis Prediction Models
Logit and probit analysis are statistical techniques used for regression and categorizing observations. They are nonlinear probability models that represent a single selection, using the utmost probability procedure that predicts the best combination given rise to the patterns of observation in the data. Logit analysis is similar to probit analysis except for the calculation of probability. Logit analysis uses the cumulative logistic function, whereas probit analysis uses the cumulative standard normal distribution. Some of the significant research in the area of business failure has used logit and probit analysis (McKelvey and Zavoina,1975; Gentry, Newbold, and Whitford, 1985).
Recursive Partitioning Algorithm (RPA)
Recursive partitioning algorithm is an automated nonparametric grouping procedure based on figure identification. RPA is likewise called a classification and regression tree. It has characteristics of both the multivariate data content and univariate clarity. The binary tree of this model explains a specific firm’s failure and can avoid assumption about the distribution of the independent or dependent variable. This method seeks to reduce the lack of grouping costs when the likelihood and costs of error are specified (Jones, 1987). The disadvantage of this model is a forward selection method. It does not consider any previous grouping when introducing new grouping rules. RPA is subject to overfitting by doing extensive partitioning processes and is not widely used for predicting business failure.
Conclusion
The study of financial ratios as predictors of business organization failure has been in practice for decades. Several models have been formulated to deal with business failure. The evolution of prediction models is well documented (Levins, 1997). Most of these studies have handled or built models using samples from companies in different industries, particularly in manufacturing and retail. Financial ratios seem to be the dominate method of bankruptcy prediction. This method has the ability to predict business failure accurately and can be used for early warning to prevent bankruptcy.
A model with specific industry samples is necessary and particularly significant due to the continuous growth rate of businesses in the industries such as Internet, consulting, and computer services. The use of this model can enhance different types of business decisions such as financing and investment from interested parties. In addition, the ability of this model can provide sufficient warning to businesses before bankruptcy. Comparison between bankruptcy prediction models is beneficial from a research standpoint. Gaskill, Van Auken, and Manning (1993) suggest that comparisons are necessary between failed and nonfailed firms. It is desirable to have a model that reliably differentiates between firms likely to be successful and those likely to be unsuccessful.
References
Altman, E. I. (1968). Financial ratios, discriminant analysis and the prediction of corporate bankruptcy. The Journal of Finance, 23(4), 589–610.
Altman, E. I. (1993). Corporate financial distress and bankruptcy: A guide to predicting and avoiding distress and profiting from bankruptcy (2nd ed.). New York: John Wiley & Sons.
Altman, E. I., & Hotchkiss, E. (2005). Corporate financial distress and bankruptcy: Predict and avoid bankruptcy, analyze and invest in distressed debt (3rd ed.). New York: John Wiley & Sons.
Beaver, W. H. (1966). Financial ratios as predictors of failure. Journal of Accounting Research, 4(Suppl. 3), 71–111.
Deakin, E. B. (1972). A discriminant analysis of predictors of business failures. Journal of Accounting Research, 10(1), 167–179.
Gaskill, L. R., Van Auken, H. E., & Manning, R. A. (1993). A factor analytic study of the perceived causes of small business failure. Journal of Small Business Management, 31, 18–31.
Gentry, J., Newbold, P., & Whitford, D. T. (1985). Classifying bankrupt with fund flow. Journal of Accounting Research, 23(1), 146–160.
Jones, F. L. (1987). Current techniques in bankruptcy prediction. Journal of Accounting Literature, 6, 131–164.
Laitinen, E. K. (1991). Financial ratios and different failure process. Journal of Business Finance and Accounting, 18(5), 649–673.
Levins, J. W. (1997). A review of bankruptcy models. The Journal of Applied Management and Entrepreneurship, 3(2), 171–184.
Meyer, P. A., & Pifer, H. W. (1970). Prediction of bank failures. Journal of Finance, 25(4), 853–868.
McKelvey, R. D., & Zavoina, W. (1975). A statistical model for the analysis of ordinal dependent variables. Journal of Mathematical Sociology, 4, 103–120.
New Generation Research, Inc. (2000). A brief history of bankruptcy. Retrieved 2008, from http://www.BankruptcyData.com/Researchcenter/briefhistory
Poston, K. M., & Harmon, W. K. (1994). A test of financial ratios as predictors of turnaround versus failure among financially distressed firms. Journal of Business Research, 10(1), 41–56.
Wilcox, J. W. (1971). A simple theory of financial ratios as predictors of failure. Journal of Accounting Research, 2, 389–395.
Zavgren, C. V. (1983). The prediction of corporate failure: The state of the art. Journal of Accounting Literature, 2, 1–38.
Cite this Page
Bankruptcy Prediction Models. (2018, Nov 29). Retrieved from https://phdessay.com/bankruptcy-prediction-models/
Run a free check or have your essay done for you
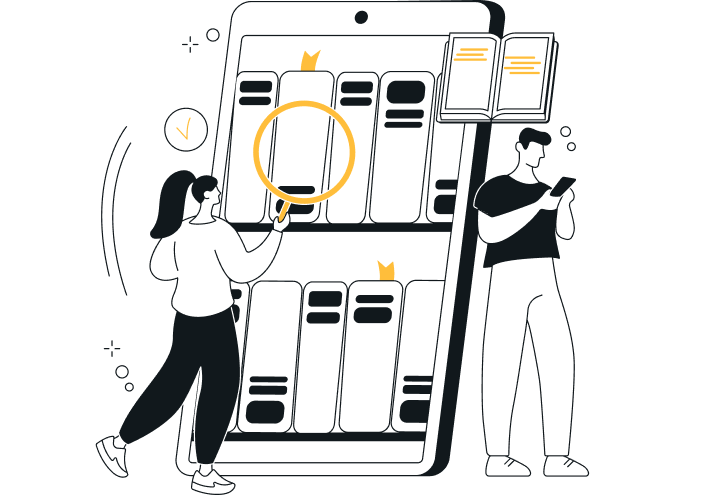