Statistics Coursework
1st Hypothesis - For my first hypothesis I will investigate the relationship between the number of TV hours watched per week by the pupils against their IQ. I am going to use the columns "IQ" and "Average number of hours TV watched per week" taken from the Mayfield high datasheet. I think that there will be a relationship between them and will attempt to reveal it.
2nd Hypothesis - For my second hypothesis I will investigate the relationship between "Average number of TV hours watched per week" and "weight (kg)". I think that there will not be any major relationship between as they will not affect each other greatly.
I will present my analysis and the results in graphs and tables and explain the results using the correlation of the graphs and arrangements of the figures.
Order custom essay Statistics Coursework with free plagiarism report
I will select a number of pupils to base my data on and will use random sampling to ascertain the correct number of male and female pupils needed to make the investigation fair.
Stratified Sampling
I do not want to use all of the data in the database for my analysis so I will need to take a sample of the number of people in the school. I would like to take about 10% of the overall figure. I will also need to use stratified sampling to make it an equal proportion of the number of males and females in the school to make it fair.
The total number of pupils at the school is 813 so I will need to take 10% as my number, 81.3 is rounded down to 81.
The overall ratio for boys and girls in the school is: 414:399
Now I will need to do my sampling
Males = 414 multiplied by 81 = 41
813
Females = 399 multiplied by 81 = 40
813
Random Sampling
Now I have the number of samples I will need to select the samples I will be taking. To do this I will use random sampling. I will take random samples until I have 81. I can do this on Excel using the following formula: = round(round()*120.
Once I have gathered the samples I am ready to start analyzing my samples.
Analysis
Hypothesis 1 Males
The first thing I need to do in my analysis is to analyze my graphs which are the source of the investigation. I have created scatter graphs to show the relationship if the two data sources for my first hypothesis. I have separated them into male and female graphs as there is a separation in the numbers.
First male scatter graph:
This first graph presented a bit of a problem. There was an anomalous result that affected the trend line and the scale of the graph. I decided to create a new graph that didn't include that 1 piece of data. This way it would help me to analyze the rest of the data.
Second male scatter graph:
This graph showed the data much clearer and I could then start analyzing it. There is no correlation between the 2 sets of data. This means that it is unlikely that there is a relationship between IQ and Average number of TV hours watched per week. In this it may be that my hypothesis is incorrect. There is only a very slight gradient on the trendline that leans towards a negative correlation, but the gradient is not steep enough to draw any conclusions about the relationship between the two sets of data. I will have to use the cumulative frequency graphs and boxplots to see if any conclusions can be made.
Cumulative frequency graphs for IQ and Average number of TV hours watched per week:
From these graphs I could create box plots and compare the two sets of data. Before that I analyzed the cumulative frequency graphs to draw initial conclusions. The majority of the IQs for males are between 90 - 105, this shows that the data is quite spread out as this section only covers a small area of the graph. For the TV hour's graph, again the data is spread among 1 main area; in this case it is between 5-25. There is almost a straight line near the top of the graph; this shows that there is likely to be some anomalous results and 0 pupils in between that result and the main bulk. Now I will create box plots so I can compare the two graphs together.
Box plots for cumulative frequency graphs of IQ and average number of TV hours watched per week: (for interquartile ranges look at copies of graphs at the back)
From the box plots I can see that the data spread is relatively the same apart from a possible anomalous result in the TV hour's data. This similarity is the reason why the scatter graph had no correlation and therefore no relationship. This means that my hypothesis is wrong.
Hypothesis 1 Females
Again I will start with the scatter graphs. As with the male graph I had an anomalous result that spread out the data and scale down the graph so most of the relevant data couldn't be analyzed. I then did another graph without that specific piece of data.
Scatter Graphs 1 and 2 to show the relationship between IQ and average number of TV hours watched per week for Females:
As you can see on both the graphs there is no correlation between the two sets of data. This again means that my first hypothesis is unlikely to be correct. There is only a slight gradient on the trend line which is not steep enough to draw any conclusions from it. There is another anomalous result on the graph but it doesn't affect the trend line and my conclusions so I left it on the graph. I will now crate cumulative frequency graphs to see if they can help me to draw conclusions.
Cumulative frequency graphs for the IQ and number of TV hours watched per week:
I will now analyze the graphs before drawing box plots to compare the graphs. The IQs graph is much more erratic which means that the data is spread over a larger range. Although there is 1 area where the data is concentrated and the gradient very steep, between 95-105. The TV hours graph is much smoother and the data less spread. The data number of hour's increases steadily to a certain point then it goes flat until the end. This means that there is a n anomalous result somewhere. I know that it can only be 1 or 2 anomalous because the point where it goes flat is at about 38 and there are only 39 sets of data in the graph. I will now look at the box plots to compare the two cumulative frequency graphs.
Box plots for cumulative frequency graphs of IQ and number of TV hours watched for females:
The box plots for these graphs show me that the IQ data has a much larger range and that it is quite evenly spread. I can see this because the interquartile range is quite large and the median evenly spread. There may be a few exceptions as 1 pupil is likey to have a very low IQ which is why the lowest value is so low. The TV hour's data seems to be much more concentrated and the data is generally lower. This shows that there can't be any relationship between them as they each grouped in certain areas. Also the box plot for TV hours shows that there is likely to bge an anomalous result as the highest value is so far out of the upper quartile.
Hypothesis 2 Males
In this hypothesis I will be comparing the Average number of TV hours watched per week and Weight, to see if there is any relationship between them. I will again start with Males and the Scatter graphs.
Scatter graphs 1 and 2 to show the relationship between Weight and the Average number of TV hours watched per week for males:
In these scatter graphs there is a slight negative correlation. This means that as the number of TV hours goes up Weight goes down. This may not be an accurate graph as there are a few anomalous results that may have caused the trend line to be that gradient. If this is so my hypothesis would have been correct, if it is not the gradient of the trend line isn't steep enough to say that it is 100% certain that it is accurate. I will need to use the cumulative frequency graphs to draw complete conclusions.
Cumulative frequency graphs for the number of TV hours watched and Weights of males:
These two graphs look quite different; the weights graph has most of its data concentrated in the middle of the range, between 30-50 and looks like a normal cumulative frequency curve. Whereas the number of TV hours has most of its data concentrated at the beginning between 0-30, showing that there is likely to be an anomalous result at the end of the range. These anomalous results on the TV hours graph are what caused the slight negative correlation on the trend line. I will be able to make complete conclusions after looking at the female sample and seeing if that graph follows suit. The box plots for these graphs will look quite different and will make it easy to make a simple comparison.
Box plots for Cumulative frequency graphs IQ and Weight for males:
From the box plots I can see that the two sets of data are almost identical in range which would cause a straight line on the scatter graph it is because of the anomalous results on the TV hours which caused the slight negative correlation. The weights box plot shows me that the data is quite evenly spread in the middle of the range apart from a very heavy person at the end which is why the highest figure is so far apart from the upper quartile. Overall the box plots show me that the similarity in the data means there is no relationship and hypothesis was correct.
Hypothesis 2 Females
Again I will start with the scatter graphs to show the relationship between Number of TV hours watched and weight. The graphs should be similar to the males and the conclusions the same. Again I had an anomalous result and had to create a second scatter graph without it there.
Scatter graphs 1 and 2 to show the relationship between the Number of TV hours watched per week and Weight:
The second scatter graph in this section, without the anomalous result completely changed the trend line. The first graph looks a lot more like the male graph whereas the second follows my hypothesis a lot better. In graph 1 there is a slight gradient on the graph which points towards a negative correlation, like those of the male sample. On the graph without the anomalous result there is clearly no correlation whatsoever as the line is nearly horizontal. I will take the results of the male sample to be wrong as I said earlier there are a few anomalous results which caused the trend line to be at that gradient. Now I will look at the cumulative frequency graphs to see what results I get from them.
Cumulative frequency graphs for Average number of TV hours watched per week and Weight for Females:
As on the males graph the TV hours for females have a lot of anomalous results. But for the scatter graphs I cancelled them all out which gave no correlation. If the line at the top of the TV hours graph is blanked out the two graphs look almost identical. This is why the scatter graph got a near horizontal trend line. The box plots for these to graphs will look alike apart from there will be a much longer line at the end of the TV hours graph because of the anomalous results.
Box plots of cumulative frequency graphs for Number of TV hours watched and weights of females:
These box plots show me the same as the males did, that the data is almost identical if placed 1 on top of the other. This is what caused the horizontal line in my scatter graphs and proves my hypothesis.
Conclusion
Hypothesis 1: My first hypothesis has been proved incorrect. The scatter graphs show that there is no correlation between the two sets of data. For my hypothesis to have been correct there would have needed to be a strong positive correlation. The cumulative frequency graphs and box plots again proved my hypothesis incorrect, the similarities in the two sets of data's box plots showed that there was no relationship and showed why the scatter graphs showed a straight line. Both the male and female samples showed that my hypothesis was incorrect although some anomalous results created a slight negative correlation in both it was obvious that it was still wrong.
Hypothesis 2: My second hypothesis was proved correct. The scatter graphs showed that there was absolutely no correlation on the graphs which means no relationship. Although the male graphs did show a a negative correlation it was proved to be made by a few anomalous results by the cumulative frequency and later the inconsistency with the female sample. The female scatter graph showed a near horizontal trend line which was what I needed to prove my hypothesis. The similarities on the cumulative frequency graphs and box plots further proved my hypothesis was correct.
Evaluation
The investigation went quite well although my first hypothjesis was incorrect it showed that careful analysis of data is needed before drawing conclusions. When I next do an investigation into data I will use histograms to aid me in my analysis as they come in useful when looking for relationships in two sets of data as the cumulative frequency graphs do. I could have made the cumulative frequency graphs a little better as the program I used did not put a scale on the x axis but only the length of the range.
Cite this Page
Statistics Coursework. (2017, Nov 07). Retrieved from https://phdessay.com/statistics-coursework/
Run a free check or have your essay done for you
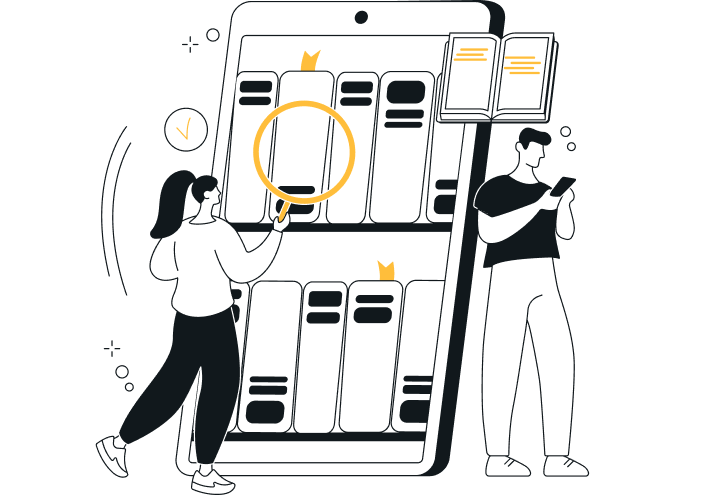