Z Score
MN 215 A & B October 02, 2012 Z Scores, Z Tests and t Tests Overview and Review At the beginning of the course we learned that there are two branches of statistics, namely, parametric and non-parametric. Further we learned that parametric statistical processes are broken down into two other categories, namely descriptive statistical processes and inferential. We learned also that descriptive statistics (mean, mode, median, standard deviation, and frequencies) are only to be used to describe the characteristics of the data rather than draw conclusions of make inferences from the measurement data collected.
However, the importance of descriptive statistics cannot be undermined as they form the basis for the workings of inferential statistical processes – especially the mean. In data analysis one of the most important concepts to remember is that regardless of the topic or issue being investigated all is based on the mean of a data set. Although we cannot draw conclusion or make predictions from descriptive statistics their usefulness in inferential statistics is significant.
As stated inferential statistics is a branch of statistics that is used in making inferences about traits or characteristics of a greater population on the basis of sample measurement data. The primary goal of inferential statistics is to leap beyond the measurement data at hand and make inferences about a greater population. Take for example a psychologist who is interested in knowing whether a new behavior modification product will likely be a seller in a certain market area.
Order custom essay Z Score with free plagiarism report
Knowing that the entire consumer population cannot be queried as to market acceptance, the psychologist would select a representative sample for the area, administer whatever measurement instrument is necessary to garner the data and, on the basis, of the sample data results, determine whether or not the new product will be profitable. The statistic used to determine whether or not the sample is representative of the entire market population would be an inferential statistics.
When using inferential statistical processes to generate information in order to make predictions about a larger population the chosen sample must always be on the basis of random selection or random assignment. Without random sampling or random assignment the mathematical values received by way of the statistical analysis are in err. Or, another way of putting is to say that the results would be “Lies, damn lies” about the data analyzed. For convenience purposes throughout the remainder of this course the following symbols will be used most extensively.
Statisticians, regardless of area, use English letters to denote sample statistics and Greek letters to symbolize population parameters. NameSample StatisticPopulation Parameter _ MeanX µ (mu) VarianceSD? ? 2 (sigma squared) Standard DeviationSD ? (sigma) Correlationr ? (rho) Proportionp ? (pi) Regression Coefficient b ? (?? beta)? ? When trying to arrive at conclusions that extend from the measurement data alone, inferential statistics are the data analysis tools of choice.
For example, inferential statistics are used to infer from the sample data to the larger population data or when there is an need to make judgments of the probability that an “observed” difference between groups is an accurate and dependable one and not those that happened by chance alone. In order too accomplish that which inferential statistics were designed two models are available: estimation testing and hypothesis testing. In the estimation model the sample measurement data is used to estimate a parameter (population) and a confidence interval about the estimate is created.
The confidence interval is basically the range of values that has a high likelihood of containing the parameter. The parameter is a numerical value that measures some part or the population measurement scores or values. The second use of inferential statistical processes is in hypothesis testing. The most common manner in which a hypothesis is tested is by developing what is commonly called a “straw man” which is what a null hypothesis is call when looking at a situation where in the research investigator wants to determine if the data collected and analyzed is strong enough to reject the null or “straw man” hypothesis.
Always remember that a null hypothesis is stated that no differences, effects or relationships will occur between and or amongst the events, occurrences, phenomenon, items, or situations being evaluated and measured as a result of some variable. A simple example of a business null hypothesis would be something like the following: There exists no statistically significant difference between widgets made of alloy A and those made of Alloy B in terms of tinsel strength acceptability. Data Requirements When Using Inferential Statistics.
Thinking back to the first part of the course we learned that statistical processes must use certain forms of numeric measurement data and this data is expressed as nominal, ordinal, interval and ratio. For descriptive statistics (frequencies and measures of central tendency) it is nominal data that is used. For inferential statistics the measurement data types to be used are either interval or ratio. However, in the social sciences and business arenas ordinal data is often times treated like interval. This is particularly true when studies attempt to assess situations by way of a Lickert scale.
For convenience and review the scale presented below will help to clarify the differences between the four scales of measurement discussed earlier in the course. Indications Indicated Direction ofIndicates Amount of Absolute Difference Difference Difference Zero NominalX OrdinalX X IntervalX X X RatioX X X On the basis of the information contained in the table above the following two conditions apply when using inferential statistical processes: * Participants selected for participation in a study should be selected randomly. If sampling is not random, then biases occur and contaminate the accuracy of the findings. The most commonly used inferential statistics that behavioral research uses are those statistical processes that provide for the determination of relationships (correlations), differences and effects between and amongst that which is being measured or evaluated. The specific tests used are the Pearson Correlation Coefficient, Chi Square, Student “t” Test, ANOVA (Analysis of Variance), and regression. All of these techniques not only require the use of a null hypothesis but independent and dependent variables as well. Z Scores
Calculating the Z Score for Research Purposes. One of the most often used statistical processes in the behavioral sciences is the Z Score. What a Z Score accomplishes is in taking a raw measurement value or score and transforms it into a standard form which then provides a more meaningful description of the individual scores within the distribution. This transformation is based on knowledge about the population's mean and standard deviation. Take for example an educational psychologist who is interested in determining how individual students are comparing to the overall group of students with respect to grades.
As we have learned before raw scores alone cannot provide insightful information to the psychologist how well an individual student is performing. However, what the psychologist can easily do is calculate a Z Score for each student and determine whether or not an individual student is functioning above or below the mean grade of all students together. When determining the placement of each individual, the Z Score permits the psychologist to calculate how many standard deviations, or the distance, each student is above or below the mean grade of all students together.
If there is an academic standard the psychologist is using as a comparative base a different statistical formula is used compared to the formula needed when comparing individual performance to a local sample of student. The formulas for each are presented below. Comparing Individual to Population Standard Comparing Individual to Sample Standard The construction of the two formulas is the same with the exception that one uses the mean and standard deviation of a population and the other of a sample.
What is very important to remember, especially for the psychologist, is that comparing an individual to a local academic setting may have entirely different results when the same student is compared to the industry standard. Although this might appear to be a dilemma, it is actually a possible “blessing in disguise”. Take for example the same psychologist compares all his students’ rate of academic success in a local facility and determines they are all functioning well above average, or above the mean, in their grades.
What happens if the same students are compared to an academic standard and the results show their grade is well below the industry standard or population mean? The conclusion drawn is, therefore, that the students, although having grades are not in line with other educational facilities and corrective programming to increase the performance rate must ensue. For ease of understanding let us look at a business situation. Example. Suppose an employee is producing 3. 5 widgets per hour and the sample average number of widgets per hour is 2. 3 with a standard deviation of 0. 33. The Z Score would be calculated as follows: X = raw score X bar = mean s = standard deviation From this we can conclude that the employee’s widget production rate per hour of 3. 5 lays 1. 73 standard deviations above the mean. We can conclude further that this employee is function above the mean all others together on the production line in terms of widget production and that the employee is doing better than 95% of the other employees and only 5% of the total employees are producing more widgets.
NOTE: The percentages are easily found on the back of the very last page of your text book. As stated earlier caution must be exercised when drawing conclusions about a single business sample as the statistical information garnered might not be representative of industry standards. Looking at the same employee on an industry standard basis the information might possibly be different. Taking the same employee with an average widget production rate of 3. 5 widgets per hour with a hypothetical population or industry standard mean of 4. 9 and a population standard deviation of 1. 15 the results would be as follows using the formula stated above: X = Employee raw production score µ = Population standard mean ? = Population standard deviation Z = (3. 5 – 4. 79) / 1. 15 = -1. 12 What can be readily seen by way of the negative value Z Score is that the employee falls below the standard industry mean with respect to the number of widgets produced in one hour. Concluding further we can say that this employee standing is surpassed by 64% of the entire population workforce for he company. Needless to say, the manager needs to take a serious look at the quality of workers in his/her plant. Interpreting the Z Score for Research Purposes. When using Standard Z Scores one must always remember that comparisons are made between individual measurement values and sample or population mean values. At no time can a one use Z Score values to make predictions or drawn inferences about any given situation. To accomplish this, inferential statistical processes must be used.
The value of the Z Score lies in the idea that individual tracking is necessary and trends can be plotted. Also, one must always keep in mind that X values do not have to be simple individual raw scores but can also reflect any investigative variable the researcher chooses to investigate. “Z” Test When to use the Z Test over the “t” Test in Research. Although both the Z test and the “t” test are used in research decision hypothesis testing each is used under a different set of circumstances than the other. The primary distinction between the two lies in the sample size requirement.
Where “t” tests can be used for small samples the Z Test cannot and is, therefore, reserved for sample situations that are larger. Both, however, perform the same function, namely to determine whether or not there are differences between the samples being evaluated or comparisons between sample and population measurements. In addition both the Z and “t” tests make use of the mean scores for raw measurement data when calculating differences. Presented below are some examples of using both the Z test and “t” test in business today. Z Test: A product safety engineer wants to investigate the average number of possible defective products in worldwide production. A sample is drawn sample (in excess of 30) and mean of the sample is compared to the population mean for evaluation. * Z Test: A psychologist wants to investigate whether or not a 10 hour shift will record more safety accidents in product production compared to the company wide population standard of eight hour shifts. * Z Test: A human resource manager wants to investigate whether or not a new employee training program will increase production numbers company wide. “t” Test: A psychologist wants to investigate whether or not the sample of 20 line employees of plant A are producing a significantly greater number of products than the sample of 20 employees of plant A. * “t” Test: A consumer product safety manager wants to investigate whether or not his small firm is producing an equal number of safe products compared to the industry standard. * “t” Test: A human resource manager is interested in knowing if customer service skills of employees in department A are the same as in department B.
What is most important to remember is that both the t and Z tests are formulated to arrive at the same conclusion but under different sampling conditions. Keep in mind as well that the Z test is used when the population mean is known. In addition when using a “t” test with a small sample base it is assumed the distribution of the data is normal; however, in larger samples the distribution does not have to be normal and a Z test can be used for comparative purposes. Further, in both situations the samples drawn must be on a random basis.
The unfortunate limitation of both tests is in the fact that neither permit any conclusions to be drawn if not differences are found between the sample means or sample and population mean. However, one must always keep in mind that Z and “t” tests are basically the same as they compare two means to determine whether or not both samples come from the same population. Calculating the Z Test. The example presented below not only provides you with a formula for both population mean testing but sample mean testing as well.
What must be closely watched is the effect on sample size with respect to any resulting Z value: Remember that the Z test requires a large sample and should a small sample be used the resulting Z value is contaminated. Formula: Sample vs. PopulationSample vs. Sample __ __ __ Z = / Z = X1 - X2 N 2(1/N + 1/N) Example Sample vs. Population:
Suppose a product manager is interested in knowing if the number of faulty washing machines being produced in his/her plant in August is indicative of the over-all number of washing machines produced in all plants during the month of August. The product manager draws two samples from his/her assembly line: a sample of 10 and a sample of 100. The example being created is to show how the size of the sample bears directly on the resulting Z Test value. Formula: __ _ Z = / N Data.
Sample Test Mean = 30 Population Mean = 25 (Industry Requirement) Population = 15 N = 10 __ Z = / N = 30-25 / 15 / 3. 16 = 15 / 4. 75 Z = 1. 58 Sample Test Mean = 30 Population Mean = 25 (Industry Requirement) Population = 15 N = 100 _ Z = / N = 30-25 / 15 / 10 = 5 / 1. 5 Z = 3. 33 Conclusion: The conclusion the production manager can draw from the above measurement example (N=10 and N=100) is relative to the size of the sample used to determine whether or not the sample is representative of the overall faulty washing machine production in August.
Had the production manager set the level of confidence at 0. 01 (99%) the Z test score needed in order to reject the null hypothesis that no differences exist in washing machine production is +1. 96. A Z test value for the 10 sample situation of +1. 58 does not meet or exceed the required value of +1. 96. Therefore, the production manager concludes there is not statistically significant difference in the August faulty washing machine production rate for his/her plant and the overall faulty washing machine production rate of all plants.
However, when the sample size is increased the resulting Z test value is extremely different. The 100 sample case, using the same values as in the 10 sample case, provides an entirely different scenario. By increasing the sample size tenfold the resulting Z test value is +3. 33. Obviously this numeric value far exceeds the required +1. 96 value and the production manager can safely conclude that statistically significant differences exist between the faulty washing machine productions in the production manager’s plant compared to the average faulty washing machine production rate of all plants.
The reason for the difference in Z test values in knowing that as sample size increases so does the Z test value. Although not shown in this example, but also extremely important, is in knowing that when the variance of the sample differs from the population variance there will exist a lower Z test value. In the 100 sample test, should the resulting Z test value not met the required 1. 96 value the production manager could have concluded that the faulty washing machine production rate of his/her plant meets the production rate of all other plants together for the month of August.
As scientific research and applied statistics application are not equipped to lend explanation as to why no differences are determined the only conclusion to be drawn is that the lack of differences is a direct result of sample size and variance. Example Sample vs. Sample vs. Sample Formula: __ __ Z = X1 - X2 2(1/N + 1/N) Example: Suppose the same product manager is interested in knowing if the number of faulty washing machines being produced in his/her plant in August is indicative of the number of faulty washing machines produced in a neighboring plant during the month of August.
The product manager draws two samples: one from his/her assembly line and one from the neighboring plant: a sample of 100 is drawn from both plants. _ Data: : Sample 1: N=100 X=30 _ Sample 2: N=100 X=25 = 15 (known or assumed) _ _ Z = X1 - X2 2(1/N + 1/N) = 30 – 25 / (15)? (1/100 + 1/100) = 5 / v (225) (. 01 + . 01) = 5 / 4. 5 = 5 / 2. 12 = 2. 35 Conclusion: On the basis of the Z test value above the production manager would have to conclude that there exists a statistically significant difference in the production rate of the two plants at the . 1 confidence level (99%) as the required critical value of 1. 96 was matched and exceeded. As such it can be stated that the two washing machine samples are not representative of each other and differences occur. Should the product manager replicate the study and use only 10 washing machines per sample the resulting Z test value would be 1. 11 and the conclusion drawn would be that no statistically significant differences are present between the two groups and the population.
Again this is an example of how sensitive the Z test is to sample size. One must always keep in mind that re-testing a product or service with artificial conveyances (smaller sample size) in order to show that differences are not present is scientifically and professionally unacceptable. Research results must be allowed to fall wherein the statistical analysis places them. Doing otherwise is using the statistical process for reasons other than that which they were intended Drawing Conclusions from the Z Test.
Business situations are not unlike any other professional situation, including the behavioral sciences, wherein the researcher or investigator is seeking information as to possible differences between samples or sample and the general population. When business managers or psychologists at any level are interested in making comparisons between products and or services the best-fit statistical tool for large sample situations is the Z test. However, the statistical value is only as good as the controls placed on it and at no time will the actual values give a reason as to why something has happened or why something has not.
With regard to the utilization of the Z test in business decision-making the following rules are always to be remembered: * Z Tests can be used to compare a sample to a population or sample to a sample for general population inference. * Z Tests are extremely susceptible to size of sample and variance and not useful when population variance is unknown. * Z Tests work best with very large samples but not with small samples as the correction factor cannot accommodate for the error associated with small samples. Z Tests are natural introductions to t Tests. * Z Tests work with only one (1) dependent variable. * Z Tests cannot work with correlated data. * Z Tests do not permit the making of strong inferences about differences or effects of the testing instrument or situation. * Z Tests have a non-parametric counterpart wherein small samples can be used. “t” Test 1a. Introduction to Difference Testing. Difference testing is used primarily to identify if there is a detectable difference between products, services, people, or situations.
These tests are often conducted in business situations to: * Ensure a change in formulation or production introduces no significant change in the end product or service. * Substantiate a claim of a new or improved product or service * Confirm that a new ingredient/supplier does not affect the perceived attributes of the product or service. * Track changes during shelf-life of a product or the length of time of a service. Differences Between Two Independent Sample Means: Coke vs. Pepsi. Let us again look at a business example wherein the independent sample t-tests are sed to compare the means of two independently sampled groups. Example: do those drinking Coke differ on a performance variable (i. e. numbers of cans consumed in one week) compared to those drinking Pepsi. The individuals are randomly assigned to the Coke and Pepsi groups. With a confidence interval or ?. 05 (corresponding probability level of 95 %) the researcher concludes the two groups are significantly different in their means (average consumption rate of Coke and Pepsi over a one week period of time) if the t test value meets or exceeds the required value.
If the t value does not meet the critical t value required then the research investigator simply concludes that no differences exist. Further explanation is not required. Presented below is a more useable situation. Example: As a manager of production let us suppose you are wanting to determine whether or not work performance is significantly (statistically) different in a noise related production line vs. a non-noise related production line. Individual Noise Production Non-Noise Production difference: 1-2 38 32 6 2 10 16 -6 3 84 57 27 4 36 28 8 5 50 55 -5 6 35 12 23 7 73 61 12 8 48 29 19 Mean 46. 8 36. 2 10. 5 Standard dev 23 19 12 Variance 529 361 N = 16 Using the raw data and formula above to calculate the t test value the actual t test value, when calculated properly, is 2. 43. Always remember that S = Standard deviation and that the mean is often times shown by the capital letter M rather than a bar mark over a capital X.
By going to the appropriate t tables in your text book find the critical value for t at the . 05 confidence interval. The value you should find is 1. 761 Differences Between Two Means of Correlated Samples: Red Bull vs. Power Drink. Again using a business example correlated t test statistical processes are used to determine whether or not there is a relationship of a particular measurement variable on a pre and post test basis. Often times when there exists a statistically significant relationship on a pre and post test basis the business manager can use the first measurement values to predict the second in future situations without having to present a post test situation.
Example: Using the same data presented above let us assume that there are not two independent groups but the same group under two different conditions – noise production environment and non-noise production environment. Individual Noise Production Non-Noise Production difference: 1-2 1 38 32 6 2 10 16 -6 3 84 57 27 4 36 28 8 5 50 55 -5 6 35 2 23 7 73 61 12 8 48 29 19 N = 8 The first step is to compute the mean of the differences: _ D = ? D N The second step is to square the differences: (6)? + (-6)? + (27)? + (8)? + (5)? + (23)? + (12)? + (19)? The third step is to calculate the standard error of the difference: SED = _ ?D - D? / n -1 n The last step is to compute the t test value: _ t = D / SED Using the raw data and formula above to calculate the t test value the actual t test value, when calculated properly, is 3. 087. By going to the appropriate t tables in your text book you can find the critical value to be, at the ? .05 confidence interval is 1. 895.
The conclusion drawn is that the differences are statistically significantly different. When to Use Independent Mean or Correlated Sample Difference Testing. In research investigation situations the choice of using an independent sample t test of a correlated sample test is dependent upon whether or not the investigator is seeking to determine differences or relationships. In some situations the need to know whether or not a difference exists between two products or services is more important than knowing if there is a relationship between the two. For example: take the consulting psychologist wants to know if training program A has better success in training managers than training method B.
The psychologist would select a sample of each training situations (generally ;30) and test the success of each sample and compare the success of program A with program B. The results would confirm if one training programs was better that the other. If, however, the psychologist was interested in determining how each program compared to the industry standard the programs would be compared, independently, to the population program mean. On the other hand should the consulting psychologist wants to determine whether or not a relationship exists, or predictability can be determine, from one program in two different situations or under two different situations a correlated t test is used.
However, knowing the relationship in pre and post test situations are generally reserved for improvement situations. Drawing Conclusions for the t Test. Any conclusion drawn for the t test statistical is only as good as the research question asked and the null hypothesis formulated. “t” tests are only used for two sample groups, either on a pre post-test basis or between two samples (independent or dependent). The t test is optimized to deal with small sample numbers which is often the case with behavioral scientists in any venue. When samples are excessively large the t test becomes difficult to manage due to the mathematical calculations involved.
Cite this Page
Z Score. (2017, Feb 22). Retrieved from https://phdessay.com/z-scores-and-z-tests-overview/
Run a free check or have your essay done for you
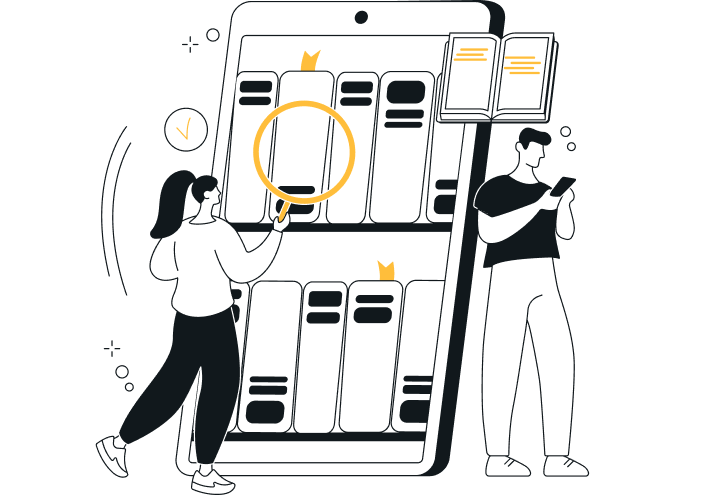